Tensor-Train-Based High-Order Dominant Eigen Decomposition for Multimodal Prediction Services
IEEE Transactions on Engineering Management(2021)
摘要
By leveraging neoteric analytical techniques associated with big data, numerous new data-focused computation and service models have flourished in service computing systems. Accurate future predictions based on tensor-based multivariate Markov models can vigorously support enterprise decisions. However, the computation efficiency and quick response of tensor-based multimodal prediction approach are seriously restricted by the curse of dimensionality arising from high-order tensor. Therefore, to alleviate the problem, this paper focuses on proposing a tensor-train (TT)-based computation approach with its scalable implementation for high-order dominant eigen decomposition (HODED) in multivariate Markov models. First, we present a TT-based Einstein product directly based on decomposed TT cores and guarantee that the result remains TT format. Then, we put forward a scalable implementation for TT-based Einstein product in a distributed or parallel manner. Afterwards, we propose a scalable TT-based HODED (TT-HODED) algorithm and a multimodal accurate prediction algorithm. Furthermore, a TT-based big data processing and services framework is presented to provide accurate proactive services. Experimental results based on real-world GPS trajectory dataset demonstrate that TT-HODED algorithm can significantly improve the computation efficiency and reduce the running memory on the premise of guaranteeing the almost consistent prediction accuracy compared to the original HODED algorithm.
更多查看译文
关键词
Accurate services,big data,high-order dominant eigen decomposition (HODED),multivariate Markov model,multimodal prediction,scalable tensor computations,tensor-train (TT)-based Einstein product
AI 理解论文
溯源树
样例
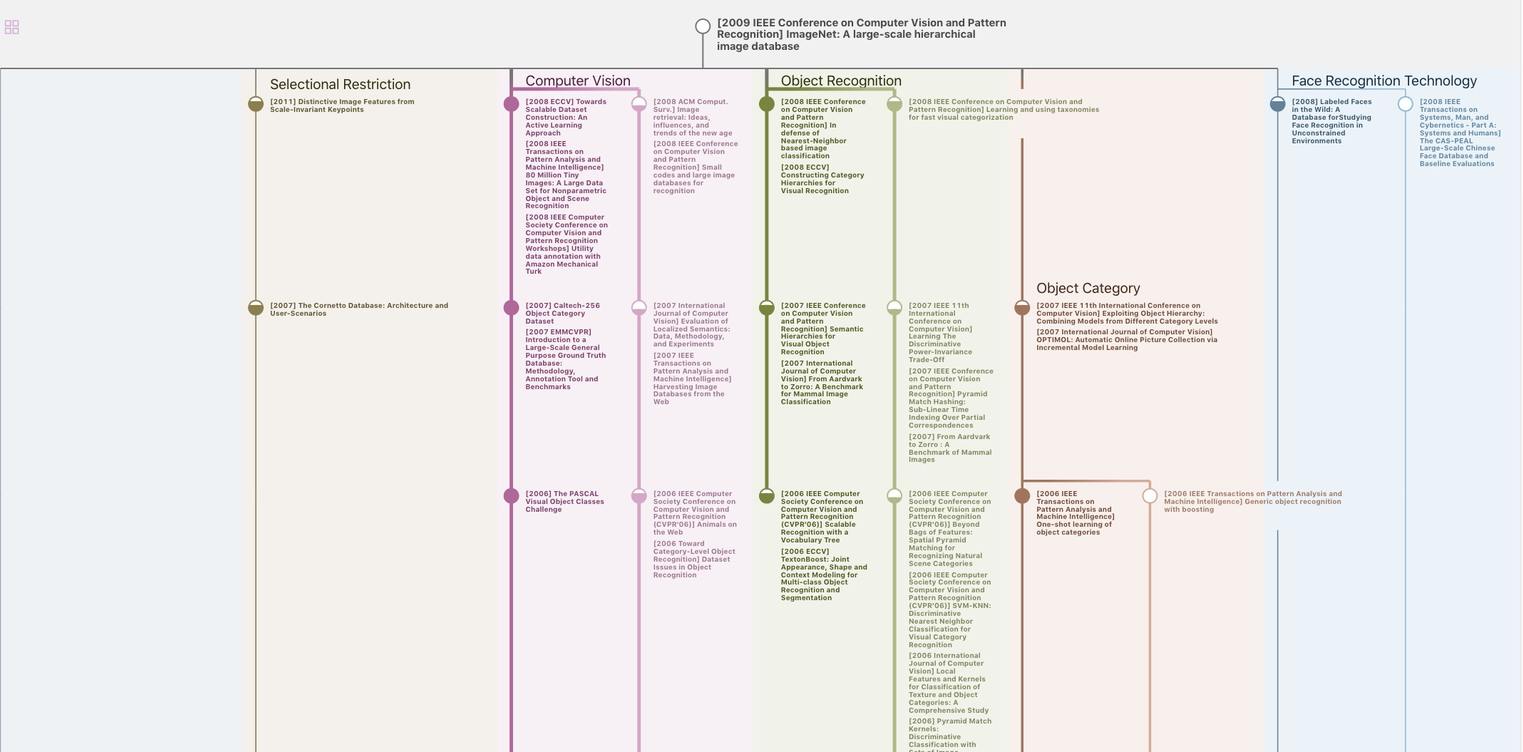
生成溯源树,研究论文发展脉络
Chat Paper
正在生成论文摘要