Deep Edge Map Guided Depth Super Resolution
SIGNAL PROCESSING-IMAGE COMMUNICATION(2021)
摘要
Accurate edge reconstruction is critical for depth map super resolution (SR). Therefore, many traditional SR methods utilize edge maps to guide depth SR. However, it is difficult to predict accurate edge maps from low resolution (LR) depth maps. In this paper, we propose a deep edge map guided depth SR method, which includes an edge prediction subnetwork and an SR subnetwork. The edge prediction subnetwork takes advantage of the hierarchical representation of color and depth images to produce accurate edge maps, which promote the performance of SR subnetwork. The SR subnetwork is a disentangling cascaded network to progressively upsample SR result, where every level is made up of a weight sharing module and an adaptive module. The weight sharing module extracts the general features in different levels, while the adaptive module transfers the general features to the specific features to adapt to different degraded inputs. Quantitative and qualitative evaluations on various datasets with different magnification factors demonstrate the effectiveness and promising performance of the proposed method. In addition, we construct a benchmark dataset captured by Kinect-v2 to facilitate research on real-world depth map SR.
更多查看译文
关键词
Super resolution, Depth map, Edge prediction, Disentangling
AI 理解论文
溯源树
样例
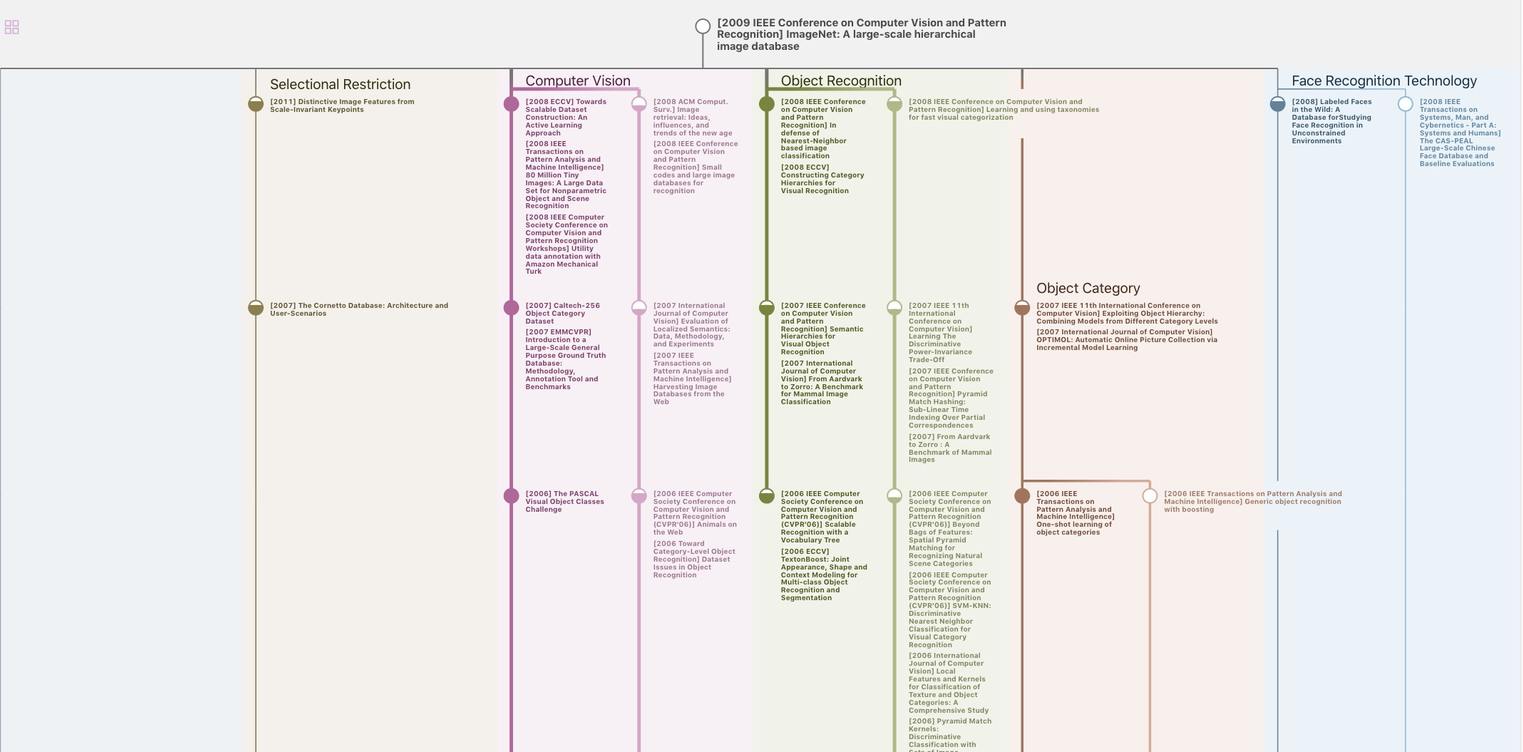
生成溯源树,研究论文发展脉络
Chat Paper
正在生成论文摘要