Nonlinear Eigenvector Methods For Convex Minimization Over The Numerical Range
SIAM JOURNAL ON MATRIX ANALYSIS AND APPLICATIONS(2020)
摘要
We consider the optimization problem in which a continuous convex function is to be minimized over the joint numerical range of two Hermitian matrices. When those matrices are of large size, solving such problems by convex optimization can be computationally expensive. The goal of this paper is to present a novel nonlinear eigenvector method to accelerate the computation. We will show that the global minimizer of the optimization problem corresponds to a solution of a nonlinear eigenvalue problem with eigenvector nonlinearity (NEPv). The special structure of this NEPv allows for an efficient sequential subspace search algorithm, which is a nonlinear analogue to the NEPv of the commonly applied locally optimal conjugate gradient descent methods for Hermitian linear eigenvalue problems. Our new algorithm can be proven globally convergent to an eigenvector of the NEPv. Implementation details such as block iteration and preconditioning will be discussed. Numerical examples, with applications in computing the coercivity constant of boundary integral operators and solving multicast beamforming problems, show the effectiveness of our approach.
更多查看译文
关键词
numerical range, Rayleigh quotient, nonlinear eigenvalue problem, eigenvector nonlinearity, sequential subspace method
AI 理解论文
溯源树
样例
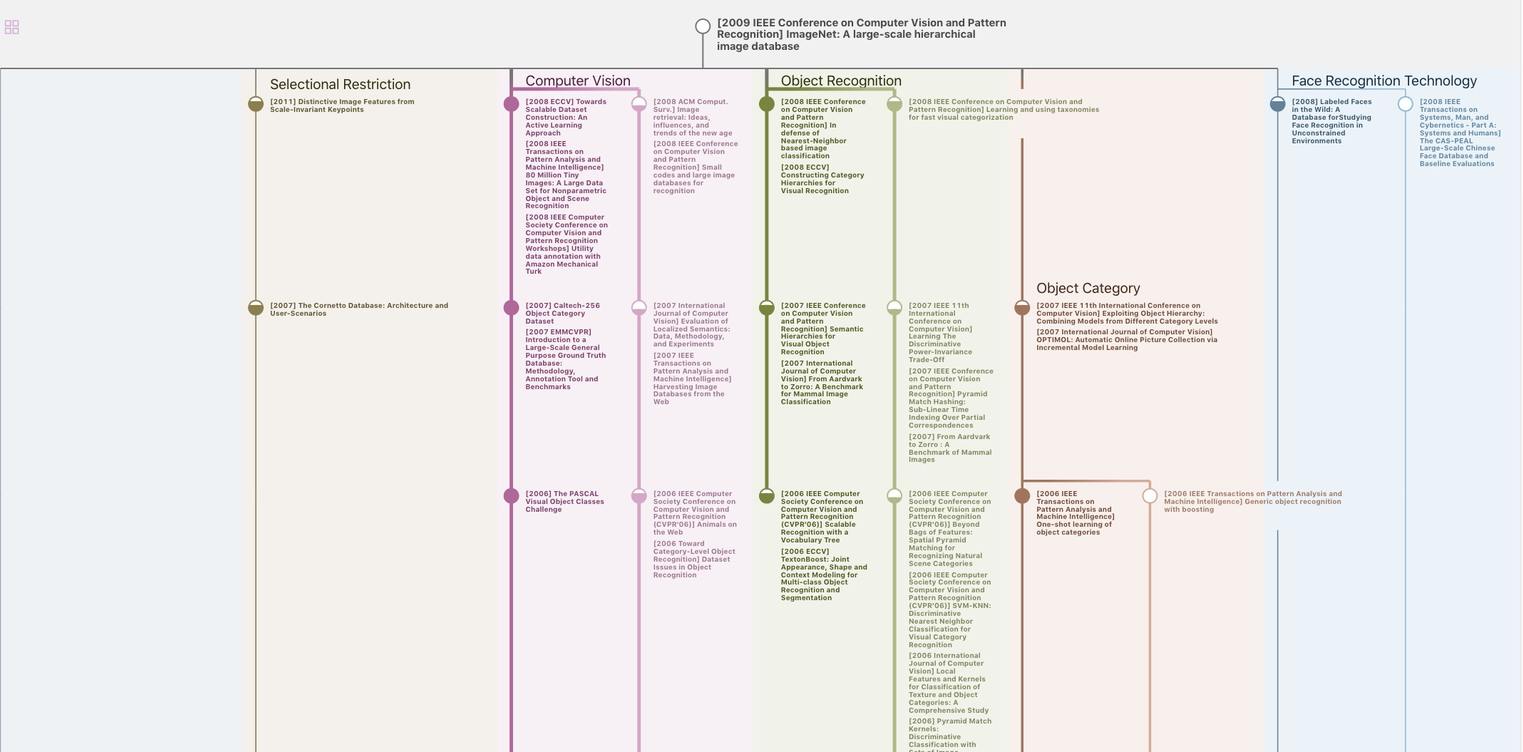
生成溯源树,研究论文发展脉络
Chat Paper
正在生成论文摘要