An Attention-based Deep Relevance Model for Few-shot Document Filtering
ACM Transactions on Information Systems(2020)
摘要
AbstractWith the large quantity of textual information produced on the Internet, a critical necessity is to filter out the irrelevant information and organize the rest into categories of interest (e.g., an emerging event). However, supervised-learning document filtering methods heavily rely on a large number of labeled documents for model training. Manually identifying plenty of positive examples for each category is expensive and time-consuming. Also, it is unrealistic to cover all the categories from an evolving text source that covers diverse kinds of events, user opinions, and daily life activities. In this article, we propose a novel attention-based deep relevance model for few-shot document filtering (named ADRM), inspired by the relevance feedback methodology proposed for ad hoc retrieval. ADRM calculates the relevance score between a document and a category by taking a set of seed words and a few seed documents relevant to the category. It constructs the category-specific conceptual representation of the document based on the corresponding seed words and seed documents. Specifically, to filter irrelevant yet noisy information in the seed documents, ADRM employs two types of attention mechanisms (namely whole-match attention and max-match attention) and generates category-specific representations for them. Then ADRM is devised to extract the relevance signals by modeling the hidden feature interactions in the word embedding space. The relevance signals are extracted through a gated convolutional process, a self-attention layer, and a relevance aggregation layer. Extensive experiments on three real-world datasets show that ADRM consistently outperforms the existing technical alternatives, including the conventional classification and retrieval baselines, and the state-of-the-art deep relevance ranking models for few-shot document filtering. We also perform an ablation study to demonstrate that each component in ADRM is effective for enhancing filtering performance. Further analysis shows that ADRM is robust under varying parameter settings.
更多查看译文
关键词
Few-shot learning, document filtering, deep learning
AI 理解论文
溯源树
样例
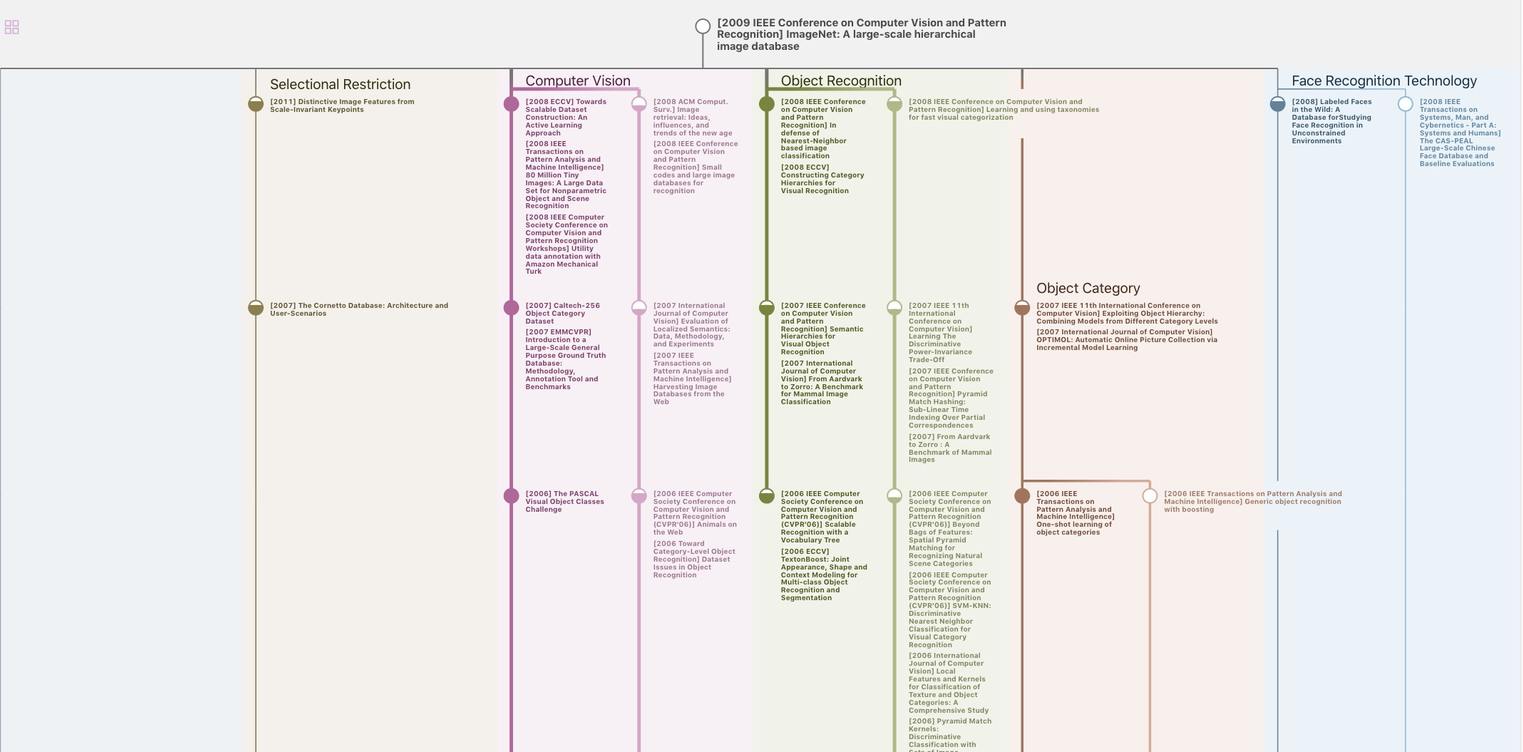
生成溯源树,研究论文发展脉络
Chat Paper
正在生成论文摘要