Neural Feature-aware Recommendation with Signed Hypergraph Convolutional Network
ACM Transactions on Information Systems(2020)
摘要
AbstractUnderstanding user preference is of key importance for an effective recommender system. For comprehensive user profiling, many efforts have been devoted to extract user feature-level preference from the review information. Despite effectiveness, existing methods mostly assume linear relationships among the users, items, and features, and the collaborative information is usually utilized in an implicit and insufficient manner, which limits the recommender capacity in modeling users’ diverse preferences. For bridging this gap, in this article, we propose to formulate user feature-level preferences by a neural signed hypergraph and carefully design the information propagation paths for diffusing collaborative filtering signals in a more effective manner. By taking the advantages of the neural model’s powerful expressiveness, the complex relationship patterns among users, items, and features are sufficiently discovered and well utilized. By infusing graph structure information into the embedding process, the collaborative information is harnessed in a more explicit and effective way. We conduct comprehensive experiments on real-world datasets to demonstrate the superiorities of our model.
更多查看译文
关键词
Recommendation system, collaborative filtering, graph convolutional network, feature-based recommendation, hypergraph neural network
AI 理解论文
溯源树
样例
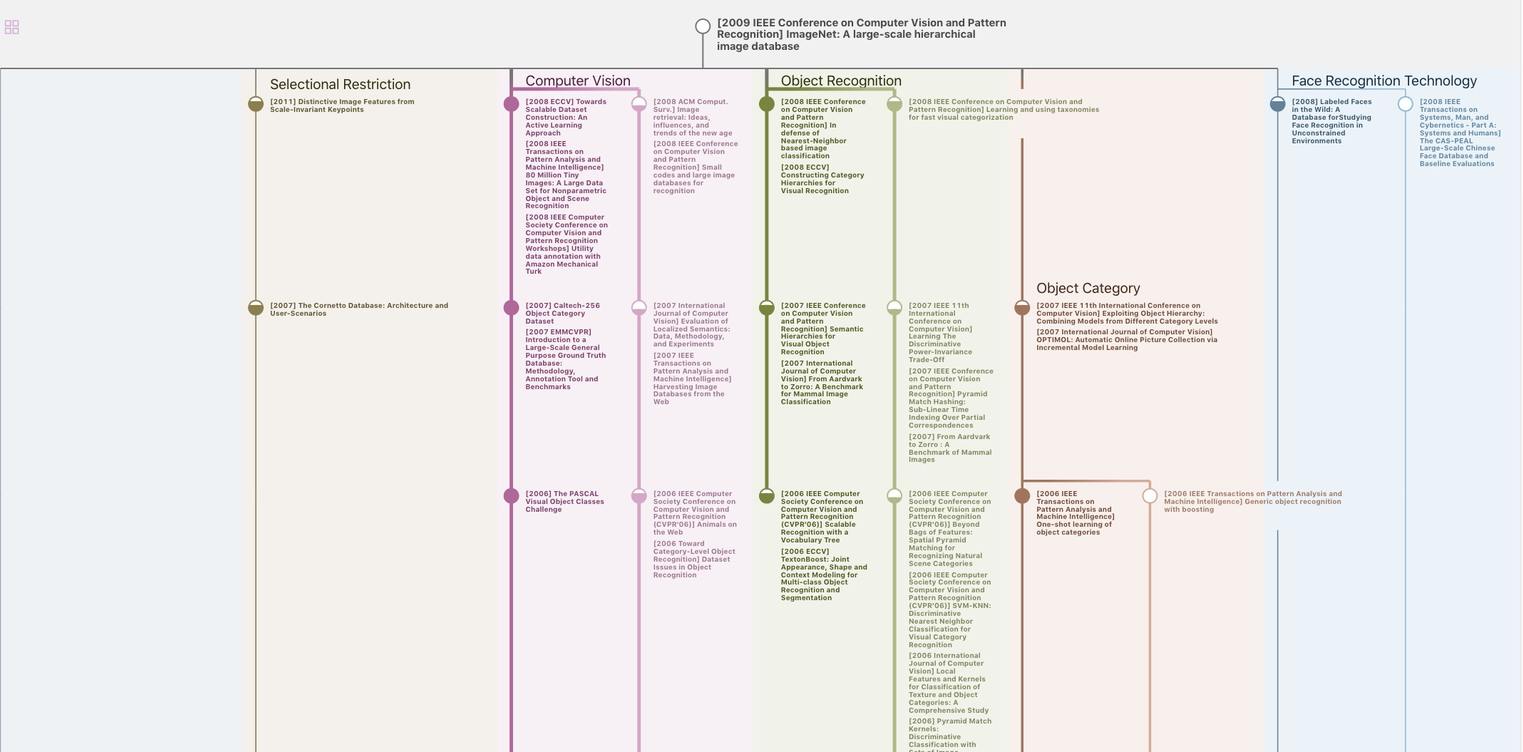
生成溯源树,研究论文发展脉络
Chat Paper
正在生成论文摘要