Dealing With Measurement Uncertainties As Nuisance Parameters In Bayesian Model Calibration
SIAM-ASA JOURNAL ON UNCERTAINTY QUANTIFICATION(2020)
摘要
In the presence of model discrepancy, the calibration of physics-based models for physical parameter inference is a challenging problem. Lack of identifiability between calibration parameters and model discrepancy requires additional identifiability constraints to be placed on the model discrepancy to obtain unique physical parameter estimates. If these assumptions are violated, the inference for the calibration parameters can be systematically biased. In many applications, such as in dynamic material property experiments, many of the calibration inputs refer to measurement uncertainties. In this setting, we develop a metric for identifying overfitting of these measurement uncertainties, propose a prior capable of reducing this overfitting, and show how this leads to a diagnostic tool for validation of physical parameter inference. The approach is demonstrated for a benchmark example and applied for a material property application to perform inference on the equation of state parameters of tantalum.
更多查看译文
关键词
Bayesian statistics, model calibration, inverse problems, overfitting, regularization, shrinkage priors, moment penalization, measurement uncertainty
AI 理解论文
溯源树
样例
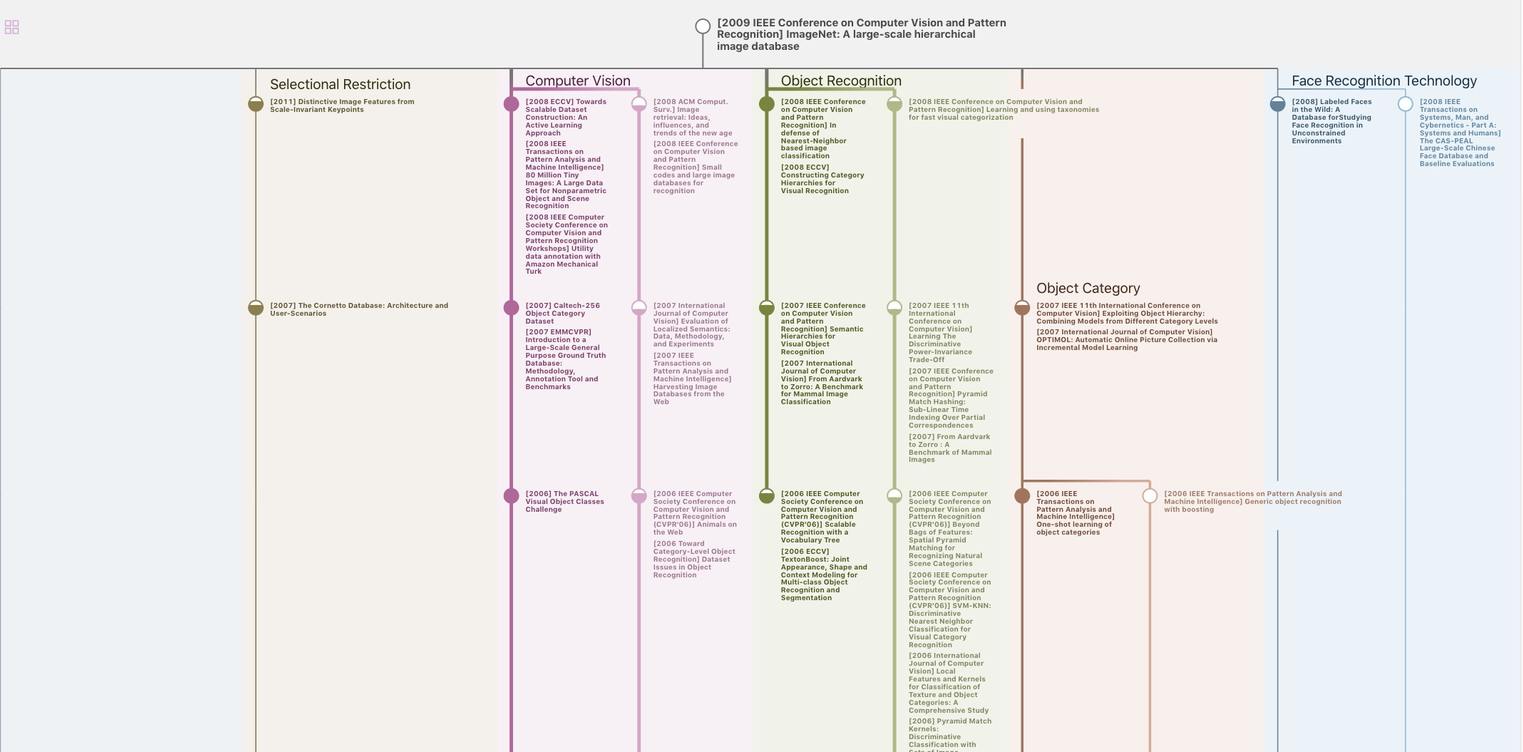
生成溯源树,研究论文发展脉络
Chat Paper
正在生成论文摘要