Geographically Local Representation Learning with a Spatial Prior for Visual Localization.
ECCV Workshops(2020)
摘要
We revisit end-to-end representation learning for cross-view self-localization, the task of retrieving for a query camera image the closest satellite image in a database by matching them in a shared image representation space. Previous work tackles this task as a global localization problem, i.e. assuming no prior knowledge on the location, thus the learned image representation must distinguish far apart areas of the map. However, in many practical applications such as self-driving vehicles, it is already possible to discard distant locations through well-known localization techniques using temporal filters and GNSS/GPS sensors. We argue that learned features should therefore be optimized to be discriminative within the geographic local neighborhood, instead of globally. We propose a simple but effective adaptation to the common triplet loss used in previous work to consider a prior localization estimate already in the training phase. We evaluate our approach on the existing CVACT dataset, and on a novel localization benchmark based on the Oxford RobotCar dataset which tests generalization across multiple traversals and days in the same area. For the Oxford benchmarks we collected corresponding satellite images. With a localization prior, our approach improves recall@1 by 9% points on CVACT, and reduces the median localization error by 2.45 m on the Oxford benchmark, compared to a state-of-the-art baseline approach. Qualitative results underscore that with our approach the network indeed captures different aspects of the local surroundings compared to the global baseline.
更多查看译文
关键词
Visual localization,Cross-view image matching,Image retrieval,End-to-end representation learning
AI 理解论文
溯源树
样例
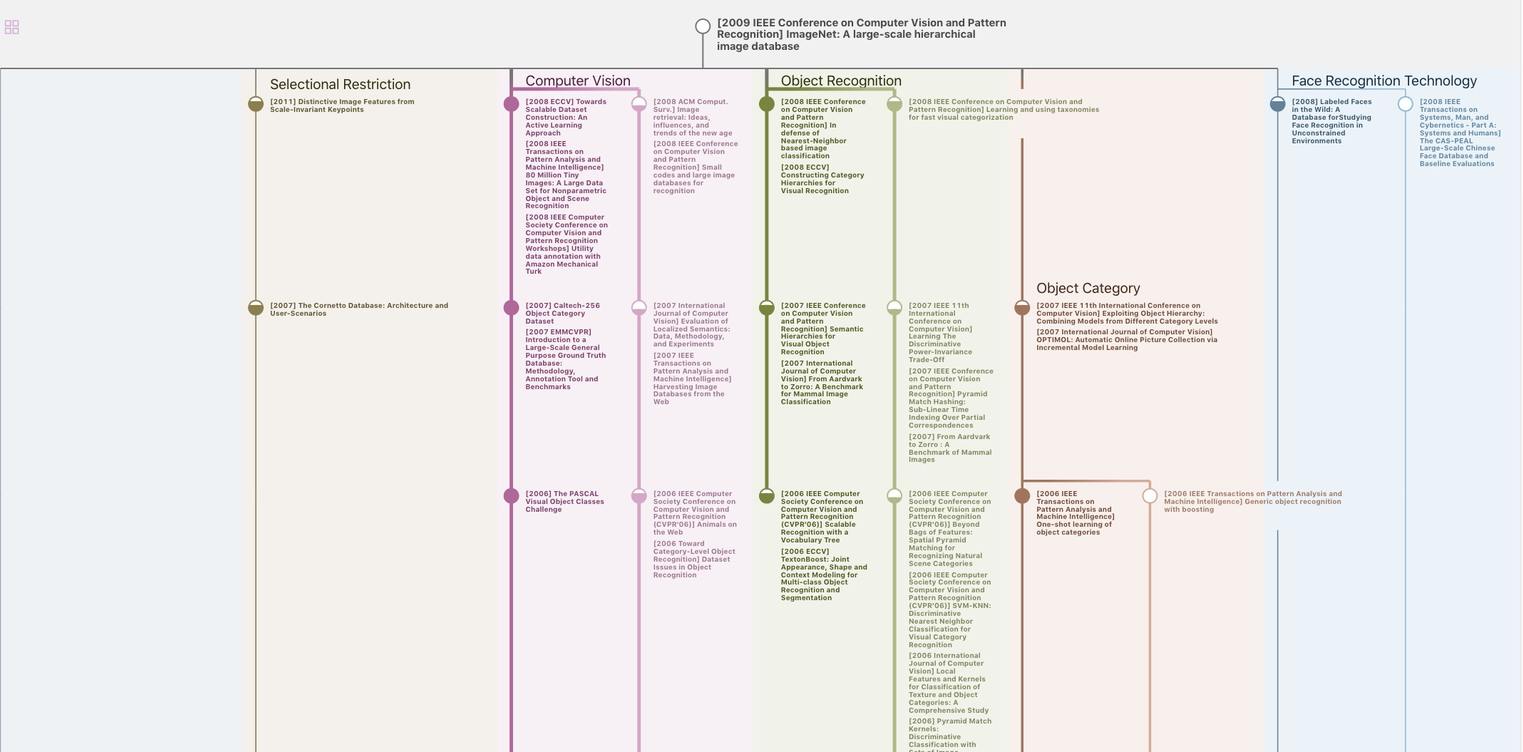
生成溯源树,研究论文发展脉络
Chat Paper
正在生成论文摘要