Conditional Adversarial Camera Model Anonymization.
ECCV Workshops(2020)
摘要
The model of camera that was used to capture a particular photographic image (model attribution) is typically inferred from high-frequency model-specific artifacts present within the image. Model anonymization is the process of transforming these artifacts such that the apparent capture model is changed. We propose a conditional adversarial approach for learning such transformations. In contrast to previous works, we cast model anonymization as the process of transforming both high and low spatial frequency information. We augment the objective with the loss from a pre-trained dual-stream model attribution classifier, which constrains the generative network to transform the full range of artifacts. Quantitative comparisons demonstrate the efficacy of our framework in a restrictive non-interactive black-box setting.
更多查看译文
关键词
Camera model anonymization,Conditional generative adversarial nets,Adversarial training,Non-interactive black-box attacks,Image editing/manipulation,Camera model attribution/identification
AI 理解论文
溯源树
样例
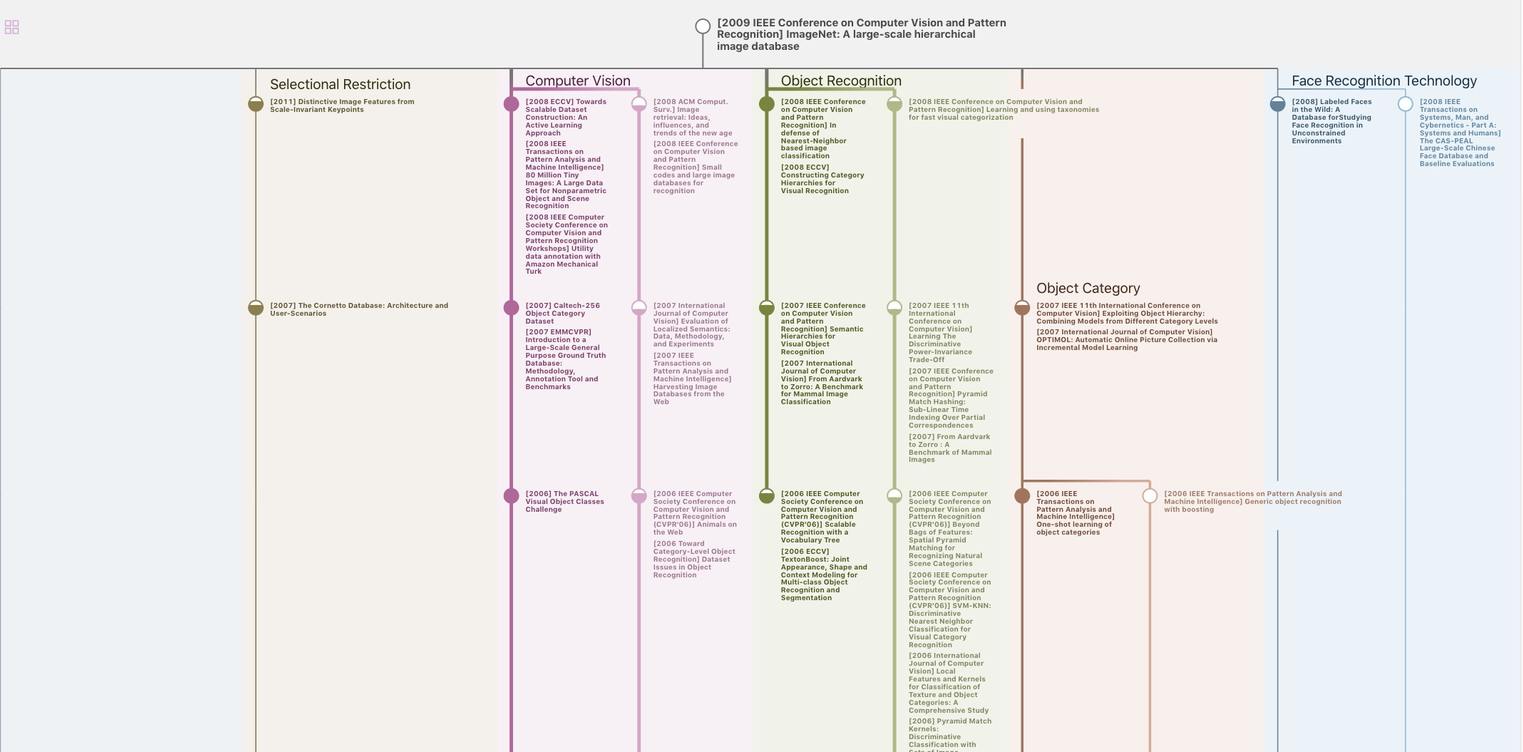
生成溯源树,研究论文发展脉络
Chat Paper
正在生成论文摘要