Speaker Variability for Emotions Classification in African Tone Languages.
LCT(2017)
摘要
In this paper, we investigate the effect of speaker variability on emotions and languages, and propose a classification system. To achieve these, speech features such as the fundamental frequency (F0) and intensity of two languages (Ibibio, New Benue Congo and Yoruba, Niger Congo) were exploited. A total of 20 speakers (10 males and 10 females) were recorded and speech features extracted for analysis. A methodological framework consisting of 4 main components: speech recording, knowledge base, preprocessing and analytic. ANOVA was used to test the intra- and inter-variability among speakers of various languages on emotions and languages, while the predictive analysis was carried out using support vector machine (SVM). We observed that language and speech features are dependent on speakers' characteristics. Furthermore, there exists a highly significant variability in the effect of emotions and languages on speech features. Results of SVM classification yielded 66.04% accuracy for emotions classification and 79.40% accuracy for language classification. Hence, classification performance favored the language classifier compared to emotion classifier, as the former produced lowroot mean squared error (RMSE) when compared with the later.
更多查看译文
关键词
speaker variability,emotions classification,tone,african
AI 理解论文
溯源树
样例
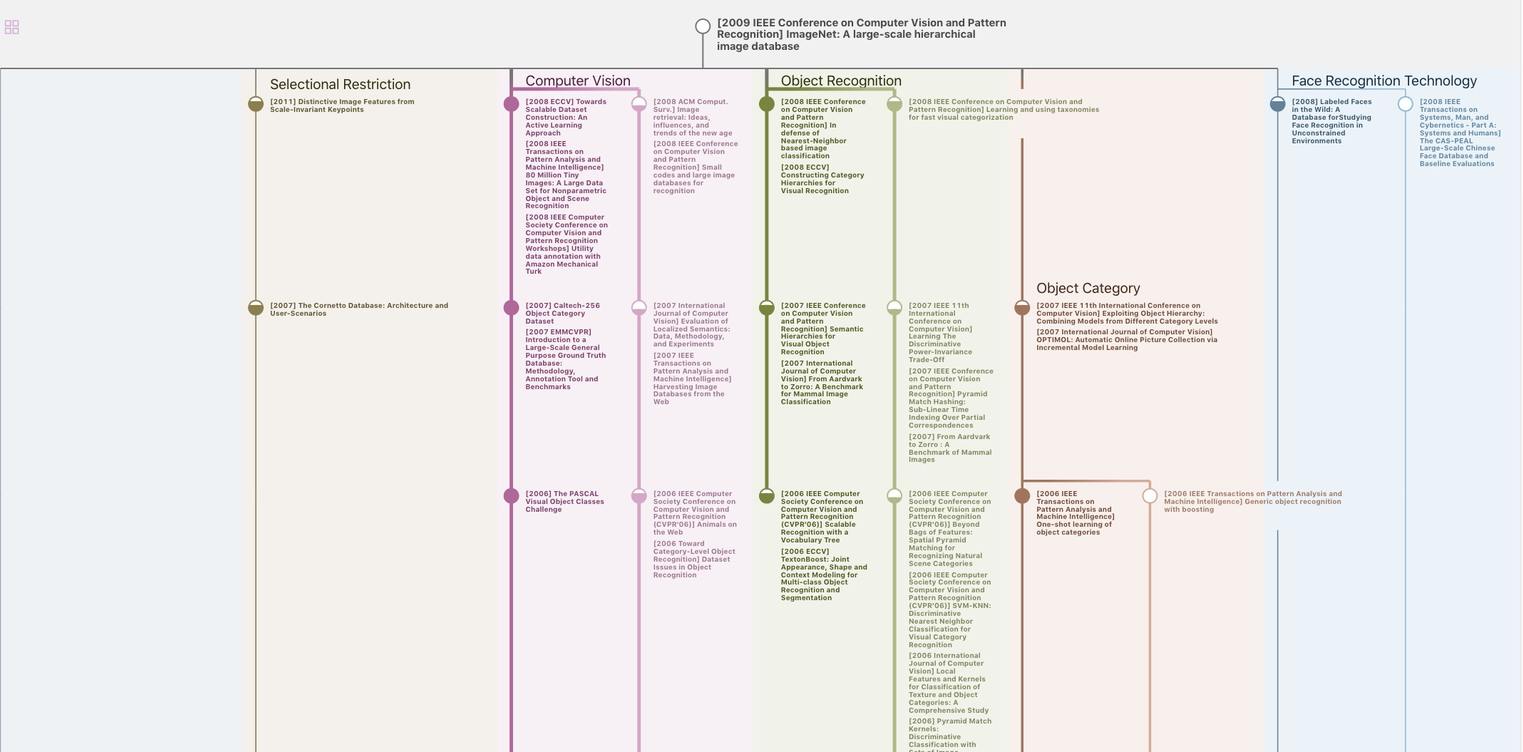
生成溯源树,研究论文发展脉络
Chat Paper
正在生成论文摘要