Feature Explainable Deep Classification For Signal Modulation Recognition
IECON 2020: THE 46TH ANNUAL CONFERENCE OF THE IEEE INDUSTRIAL ELECTRONICS SOCIETY(2020)
摘要
Signal modulation recognition plays a critical role in many fields to identify the modulation type of wireless signals. Since the deep learning based models have achieved great success in classification tasks, more deep neural networks are proposed for signal modulation recognition. In this paper, we explore the use of different deep neural networks in both macro network architecture level and micro cell size and layer level to compare and understand their effect on classification performance. We also bring up a feature explainable deep neural network by visualizing the critical features in the deep neural models. We visually show and compare the commonality and differences of hidden layer characteristics extracted by different network structure to explain and analyze the reason why some models can achieve better classification results than the others. We believe it is an effective way to explain how deep neural model based signal classification work. Thus the explanation will help users establish appropriate understand and trust in predictions from deep modulation recognition networks.
更多查看译文
关键词
deep neural network, modulation recognition, feature visualization, explainable, wireless signal
AI 理解论文
溯源树
样例
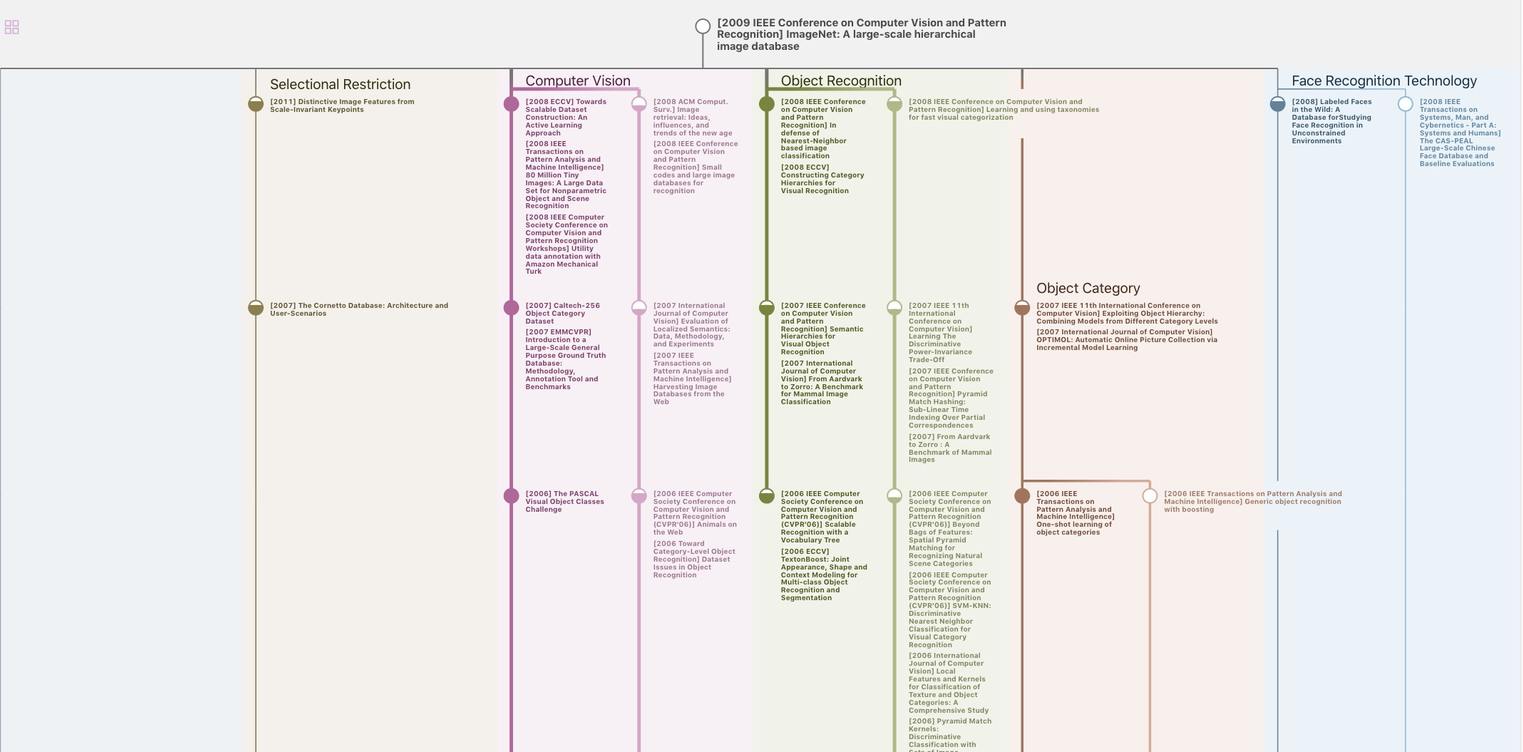
生成溯源树,研究论文发展脉络
Chat Paper
正在生成论文摘要