Synchronous Reinforcement Learning-Based Control For Cognitive Autonomy
FOUNDATIONS AND TRENDS IN SYSTEMS AND CONTROL(2020)
摘要
This monograph provides an exposition of recently developed reinforcement learning-based techniques for decision and control in human-engineered cognitive systems. The developed methods learn the solution to optimal control, zero-sum, non zero-sum, and graphical game problems completely online by using measured data along the system trajectories and have proved stability, optimality, and robustness. It is true that games have been shown to be important in robust control for disturbance rejection, and in coordinating activities among multiple agents in networked teams. We also consider cases with intermittent (an analogous to triggered control) instead of continuous learning and apply those techniques for optimal regulation and optimal tracking. We also introduce a bounded rational model to quantify the cognitive skills of a reinforcement learning agent. In order to do that, we leverage ideas from behavioral psychology to formulate differential games where the interacting learning agents have different intelligence skills, and we introduce an iterative method of optimal responses that determine the policy of an agent in adversarial environments. Finally, we present applications of reinforcement learning to motion planning and collaborative target tracking of bounded rational unmanned aerial vehicles.
更多查看译文
AI 理解论文
溯源树
样例
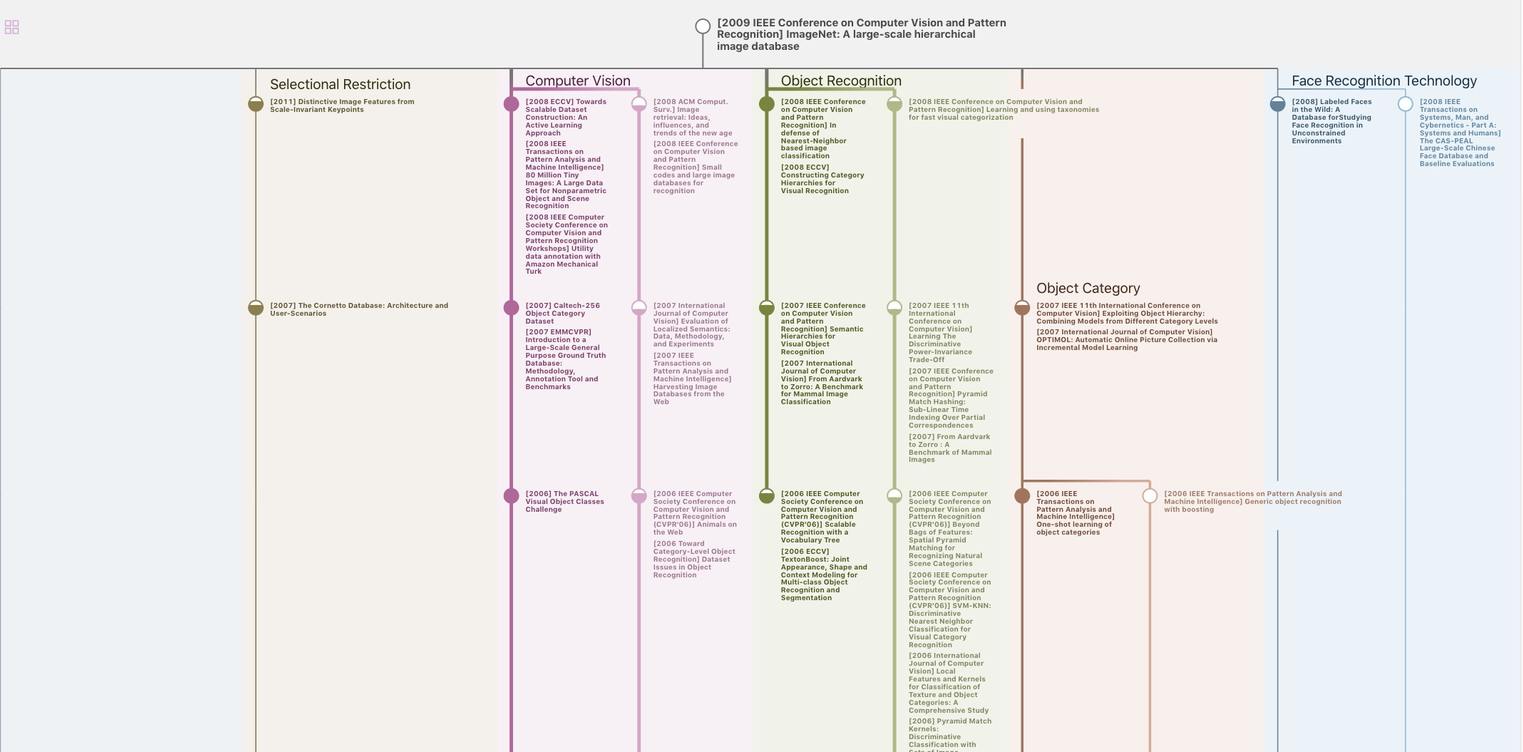
生成溯源树,研究论文发展脉络
Chat Paper
正在生成论文摘要