A Potential Energy Shaping Controller with Ground Reaction Force Feedback for a Multi-Activity Knee-Ankle Exoskeleton
International Conference on Biomedical Robotics and Biomechatronics(2020)
Abstract
This paper presents the design and implementation of a novel multi-activity control strategy for a backdrivable knee-ankle exoskeleton. Traditionally, exoskeletons have used trajectory-based control of highly geared actuators for complete motion assistance. In contrast, we develop a potential energy shaping controller with ground reaction force (GRF) feedback that facilitates multi-activity assistance from a backdrivable exoskeleton without prescribing pre-defined kinematics. Although potential energy shaping was previously implemented in an exoskeleton to reduce the user's perceived gravity, this model-based approach assumes the stance leg is fully loaded with the weight of the user, resulting in excessive control torques as weight transfers to the contralateral leg during double support. The presented approach uses GRF feedback to taper the torque control output for any activity involving multiple supports, leading to a closer match with normative joint moments in simulations based on pre-recorded human data during level walking. To implement this strategy, we present a custom foot force sensor that provides GRF feedback to the previously designed exoskeleton. Finally, results from an able-bodied human subject experiment demonstrate that the exoskeleton is able to reduce muscular activation of the primary muscles related to the knee and ankle joints during sit-to-stand, stand-to-sit, level walking, and stair climbing.
MoreTranslated text
Key words
foot force sensor,ankle joints,muscular activation,torque control,GRF feedback,backdrivable exoskeleton,motion assistance,trajectory-based control,multiactivity knee-ankle exoskeleton,ground reaction force feedback,potential energy shaping controller
求助PDF
上传PDF
View via Publisher
AI Read Science
Must-Reading Tree
Example
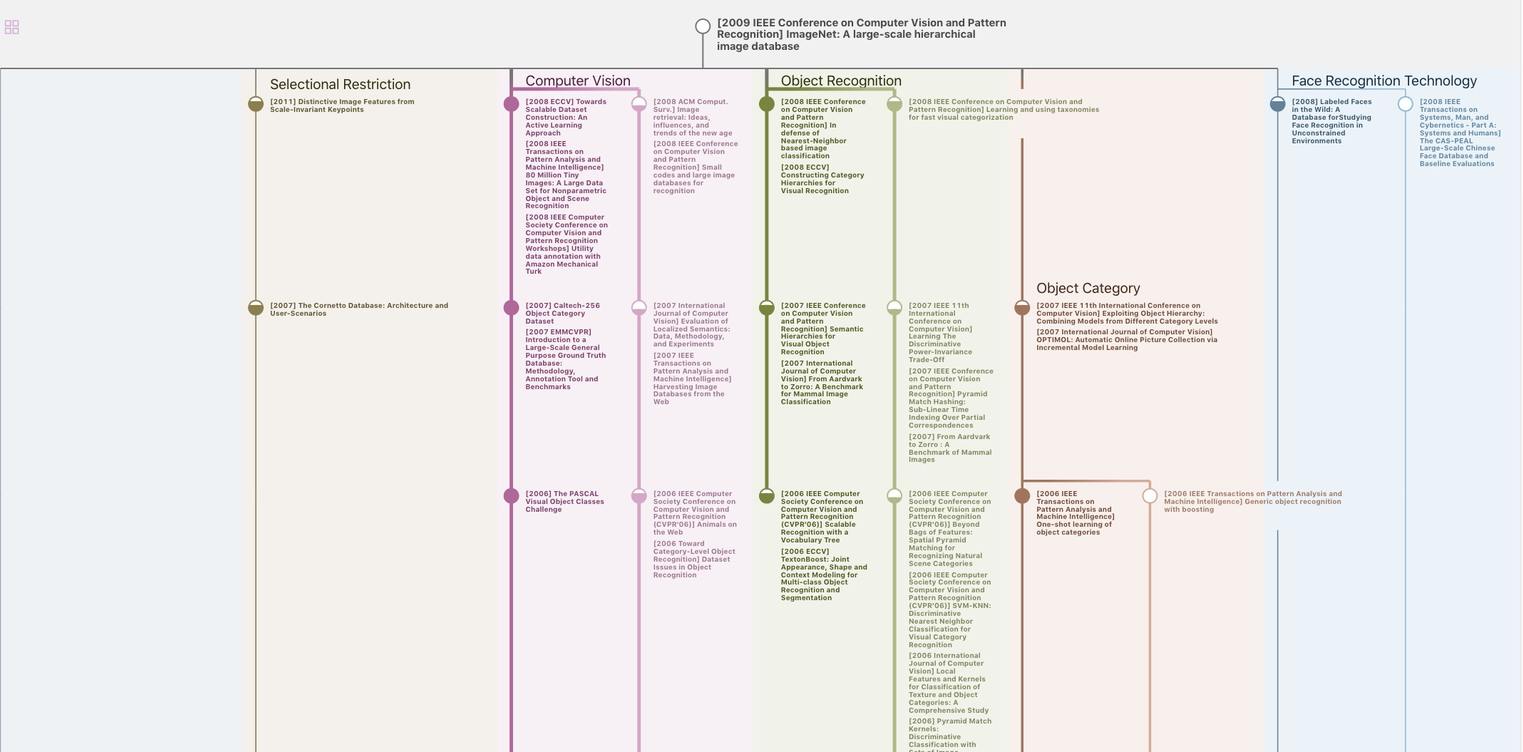
Generate MRT to find the research sequence of this paper
Data Disclaimer
The page data are from open Internet sources, cooperative publishers and automatic analysis results through AI technology. We do not make any commitments and guarantees for the validity, accuracy, correctness, reliability, completeness and timeliness of the page data. If you have any questions, please contact us by email: report@aminer.cn
Chat Paper
Summary is being generated by the instructions you defined