Towards MRI Progression Features for Glioblastoma Patients - From Automated Volumetry and Classical Radiomics to Deep Feature Learning.
MLCN/RNO-AI@MICCAI(2020)
摘要
Disease progression for Glioblastoma multiforme patients is currently assessed with manual bi-dimensional measurements of the active contrast-enhancing tumor on Magnetic Resonance Images (MRI). This method is known to be susceptible to error; in the lack of a data-driven approach, progression thresholds had been set rather arbitrarily considering measurement inaccuracies. We propose a data-driven methodology for disease progression assessment, building on tumor volumetry, classical radiomics, and deep learning based features. For each feature type, we infer progression thresholds by maximizing the correlation of the time-to-progression (TTP) and overall survival (OS). On a longitudinal study comprising over 500 data points, we observed considerable underestimation of the current volumetric disease progression threshold. We evaluate the data-driven disease progression thresholds based on expert ratings using the current clinical practice.
更多查看译文
关键词
mri progression features,glioblastoma patients,deep features learning,classical radiomics
AI 理解论文
溯源树
样例
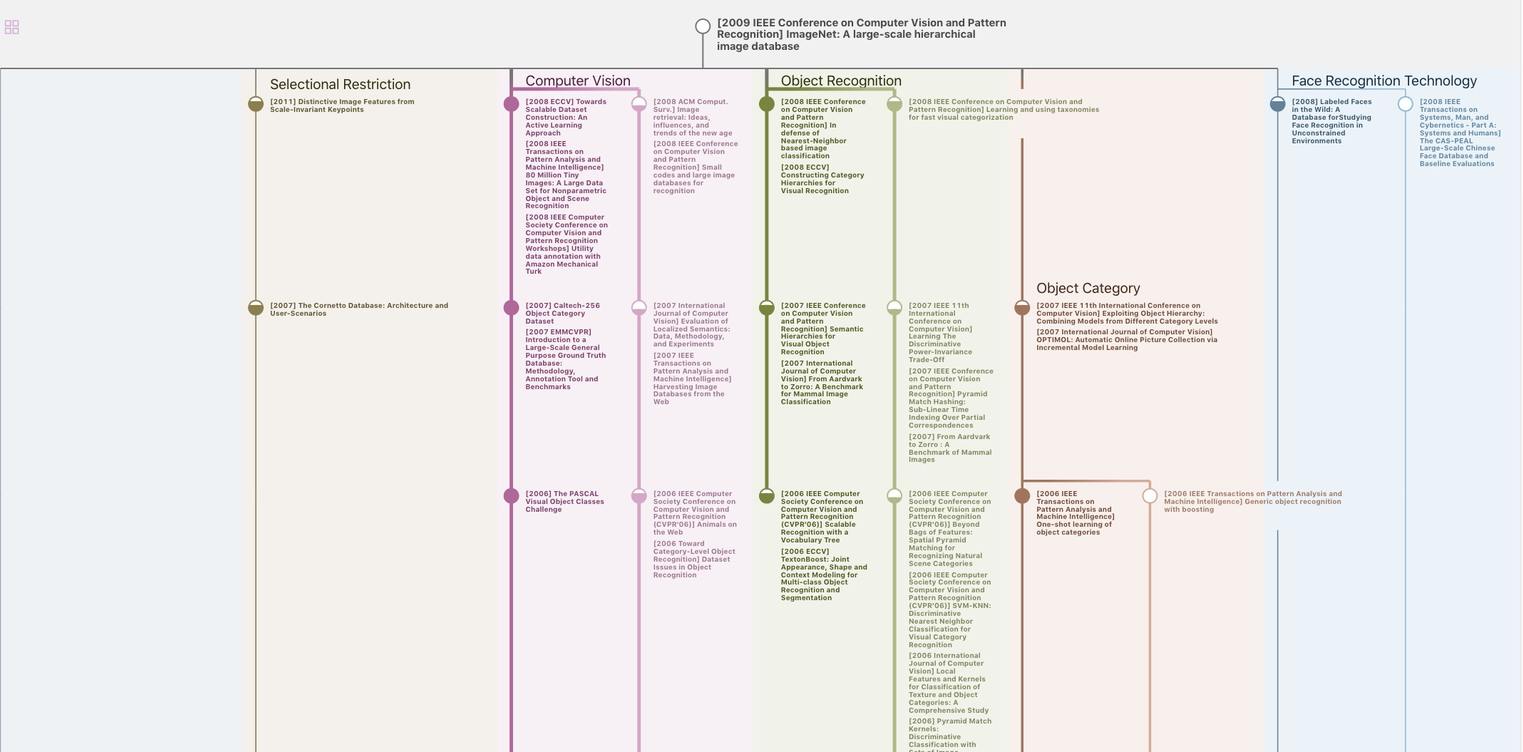
生成溯源树,研究论文发展脉络
Chat Paper
正在生成论文摘要