Automated Tag Enrichment by Semantically Related Trends.
WEBIST(2020)
摘要
The technological evolution of modern content sharing applications led to unbridled increase of video content creation and with it multimedia streaming, content sharing and video advertising. Managing huge volumes of video data becomes critical for various applications such as video browsing, retrieval, and recommendation. In such a context, video tagging, the task of assigning meaningful human-friendly words (i.e., tags) to a video, has become an important pillar for both academia and companies alike. Indeed, tags may be able to effectively summarize the content of the video, and, in turn, attract users and advertisers interests. As manual tags are usually noisy, biased and incomplete, many efforts have been recently made in devising automated video tagging approaches. However, video search engines handle a massive amount of natural language queries every second. Therefore, a key aspect in video tagging consists of proposing tags not only related to video contents, but also popular amongst users searches. In this paper, we propose a novel video tagging approach, in which the proposed tags are generated by identifying semantically related popular search queries (i.e., trends). Experiments demonstrate the viability of our proposal.
更多查看译文
关键词
Tag Annotation, Semantic Enrichment, Machine Learning, Google Trends
AI 理解论文
溯源树
样例
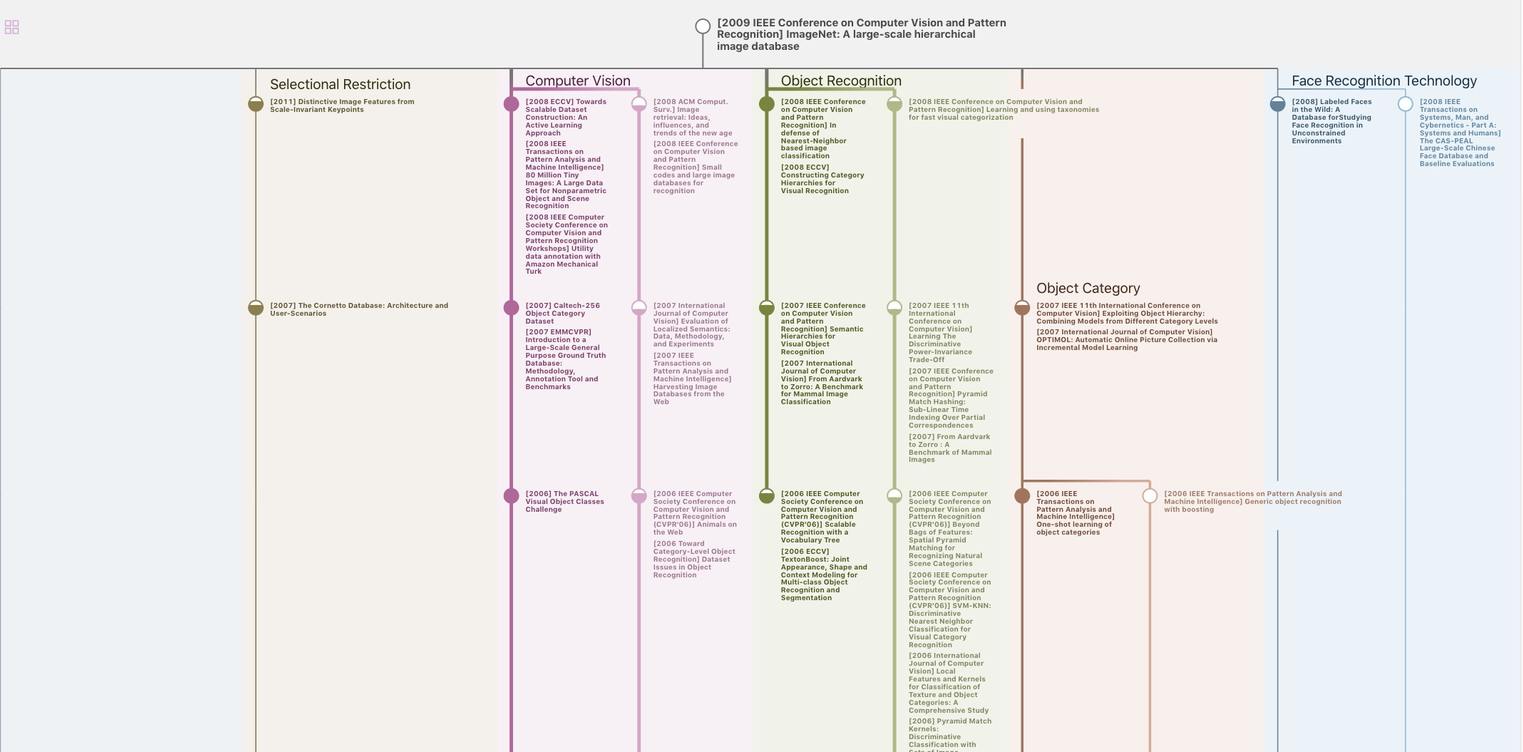
生成溯源树,研究论文发展脉络
Chat Paper
正在生成论文摘要