ε-Differential Privacy for Microdata Releases Does Not Guarantee Confidentiality (Let Alone Utility).
PSD(2020)
摘要
Differential privacy (DP) is a privacy model that was designed for interactive queries to databases. Its use has then been extended to other data release formats, including microdata. In this paper we show that setting a certain ϵ in DP does not determine the confidentiality offered by DP microdata, let alone their utility. Confidentiality refers to the difficulty of correctly matching original and anonymized data, and utility refers to anonymized data preserving the correlation structure of original data. Specifically, we present two methods for generating ϵ -differentially private microdata. One of them creates DP synthetic microdata from noise-added covariances. The other relies on adding noise to the cumulative distribution function. We present empirical work that compares the two new methods with DP microdata generation via prior microaggregation. The comparison is in terms of several confidentiality and utility metrics. Our experimental results indicate that different methods to enforce ϵ -DP lead to very different utility and confidentiality levels. Both confidentiality and utility seem rather dependent on the amount of permutation performed by the particular SDC method used to enforce DP. Thus suggests that DP is not a good privacy model for microdata releases.
更多查看译文
关键词
microdata releases,confidentiality
AI 理解论文
溯源树
样例
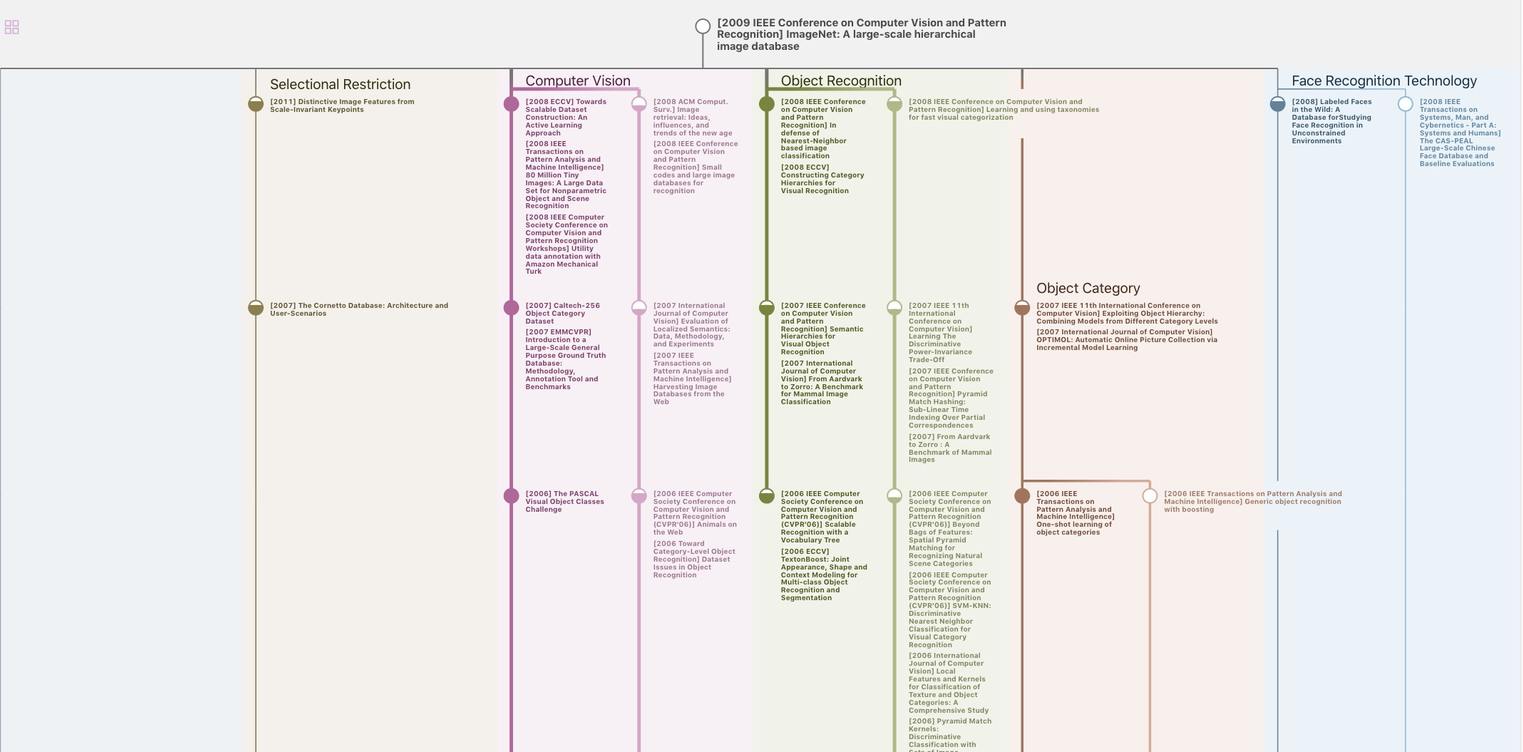
生成溯源树,研究论文发展脉络
Chat Paper
正在生成论文摘要