A Study of APIs for Graph Analytics Workloads
2020 IEEE International Symposium on Workload Characterization (IISWC)(2020)
Abstract
Traditionally, parallel graph analytics workloads have been implemented in systems like Pregel, GraphLab, Galois, and Ligra that support graph data structures and graph operations directly. An alternative approach is to express graph workloads in terms of sparse matrix kernels such as sparse matrix-vector and matrix-matrix multiplication. An API for these kernels has been defined by the GraphBLAS project. The SuiteSparse project has implemented this API on shared-memory platforms, and the LAGraph project is building a library of graph algorithms using this API. How does the matrix-based approach perform compared to the graph-based approach? Our experiments on a 56 core CPU show that for representative graph workloads, LAGraph/SuiteSparse solutions are 5x slower on the average than Galois solutions. We argue that this performance gap arises from inherent limitations of a matrix-based API: regardless of which architecture a matrix-based algorithm is run on, it is subject to the same inherent limitations of the matrix-based API.
MoreTranslated text
Key words
graph analytics,performance study,Graph-BLAS,high performance computing
AI Read Science
Must-Reading Tree
Example
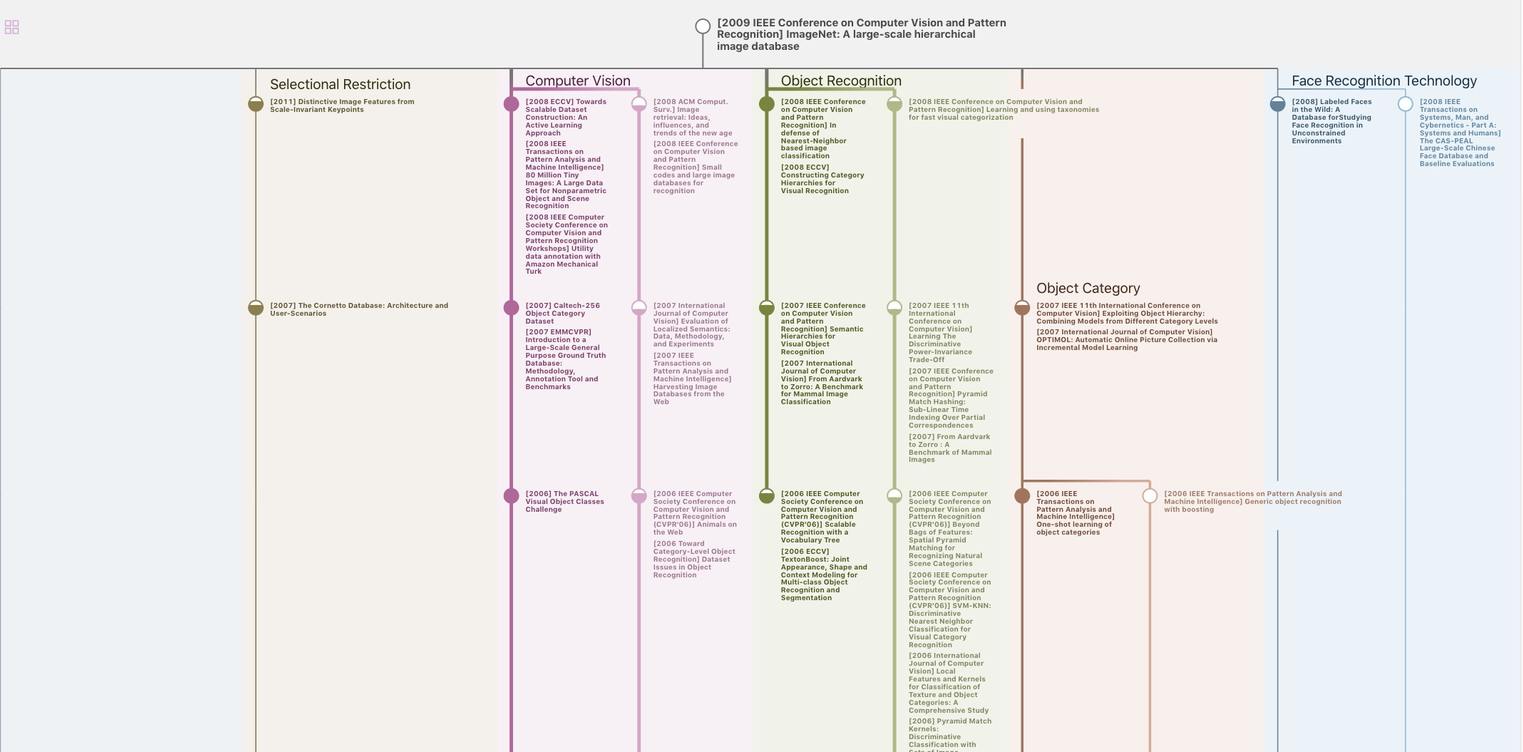
Generate MRT to find the research sequence of this paper
Chat Paper
Summary is being generated by the instructions you defined