Quantum-Inspired Algorithm with Evolution Strategy.
TPNC(2020)
摘要
Quantum-inspired algorithms are efficient for solving global search optimization problems. Nevertheless, their application is limited by two main requirements: a knowledge of a cost function and a big computational effort. To address both limitations, this paper presents a global optimization algorithm mixing a Quantum Diffusion Monte Carlo (DMC) method and an Evolution Strategy (ES). The proposed approach takes advantage of quantum models for efficiently finding solutions close the global optimum by applying the DMC. However, the DMC needs to be adapted to an optimization process. In order to improve relatively slow convergence rate of the DMC, we integrate a step size control for a diffusion displacement process of the DMC. Then, an (1+1)-ES is applied to find the minimum value of the cost function. Experimentation shows that the proposed approach can find global minima with fewer objective function evaluations than Quantum Particle Swarm Optimization and Quantum Annealing. Thus, it seems to be promising for solving black-box problems, where a reliable trade-off between exploration and exploitation is required.
更多查看译文
关键词
algorithm,evolution,quantum-inspired
AI 理解论文
溯源树
样例
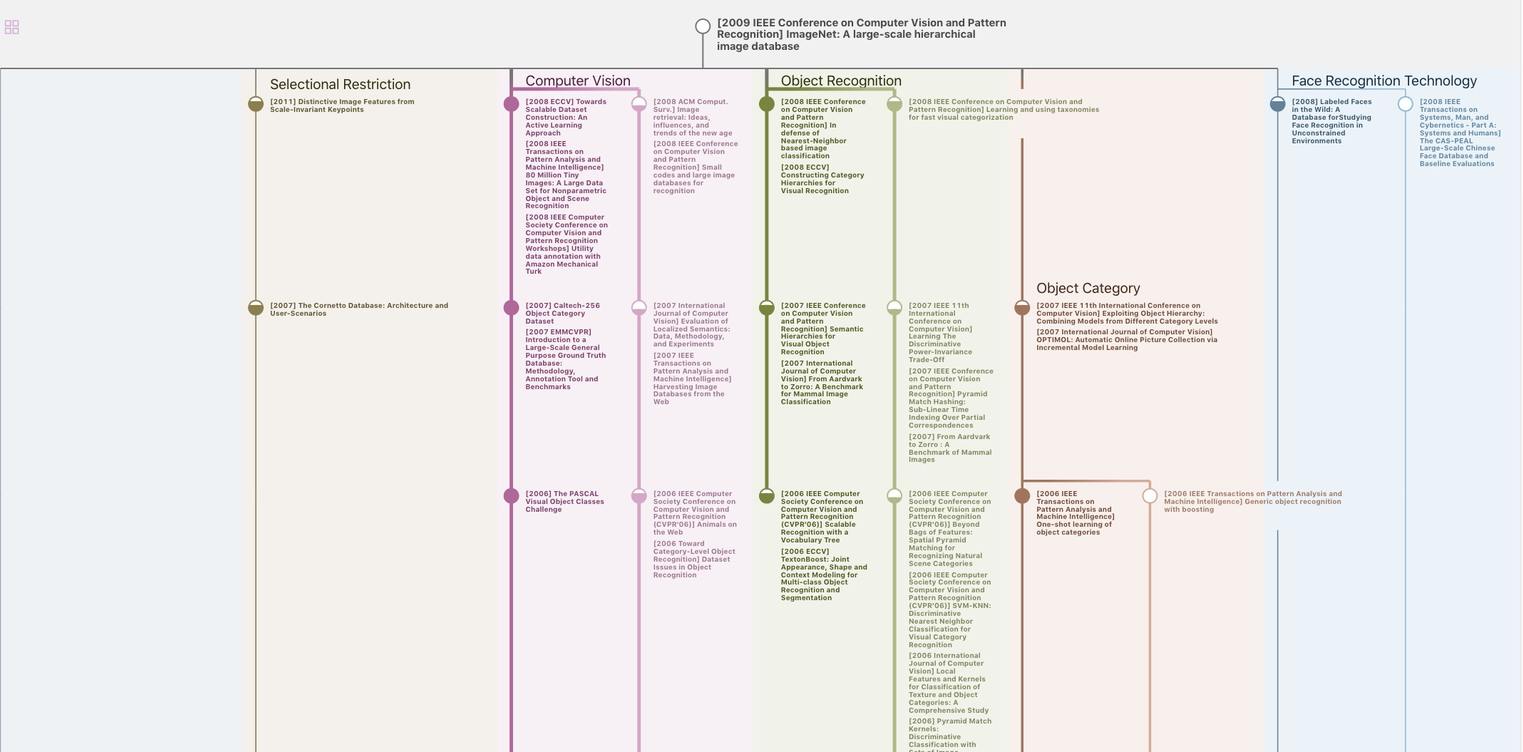
生成溯源树,研究论文发展脉络
Chat Paper
正在生成论文摘要