A Regularization-adaptive Non-negative Latent Factor Analysis-based Model For Recommender Systems.
ICHMS(2020)
摘要
Non-negative latent factor analysis (NLFA) can high-efficiently extract useful information from high dimensional and sparse (HiDS) matrices often encountered in recommender systems (RSs). However, an NLFA-based model requires careful tuning of regularization coefficients, which is highly expensive in both time and computation. To address this issue, this study proposes an adaptive NLFA-based model whose regularization coefficients become self-adaptive via particle swarm optimization. Experimental results on two HiDS matrices indicate that owing to such self-adaptation, it outperforms an NLFA model in terms of both convergence rate and prediction accuracy for missing data estimation.
更多查看译文
关键词
Recommender System, Non-negative Latent Factor Analysis, Particle Swarm Optimization, High-Dimensional and Sparse Matrix, Self-adaptive Model
AI 理解论文
溯源树
样例
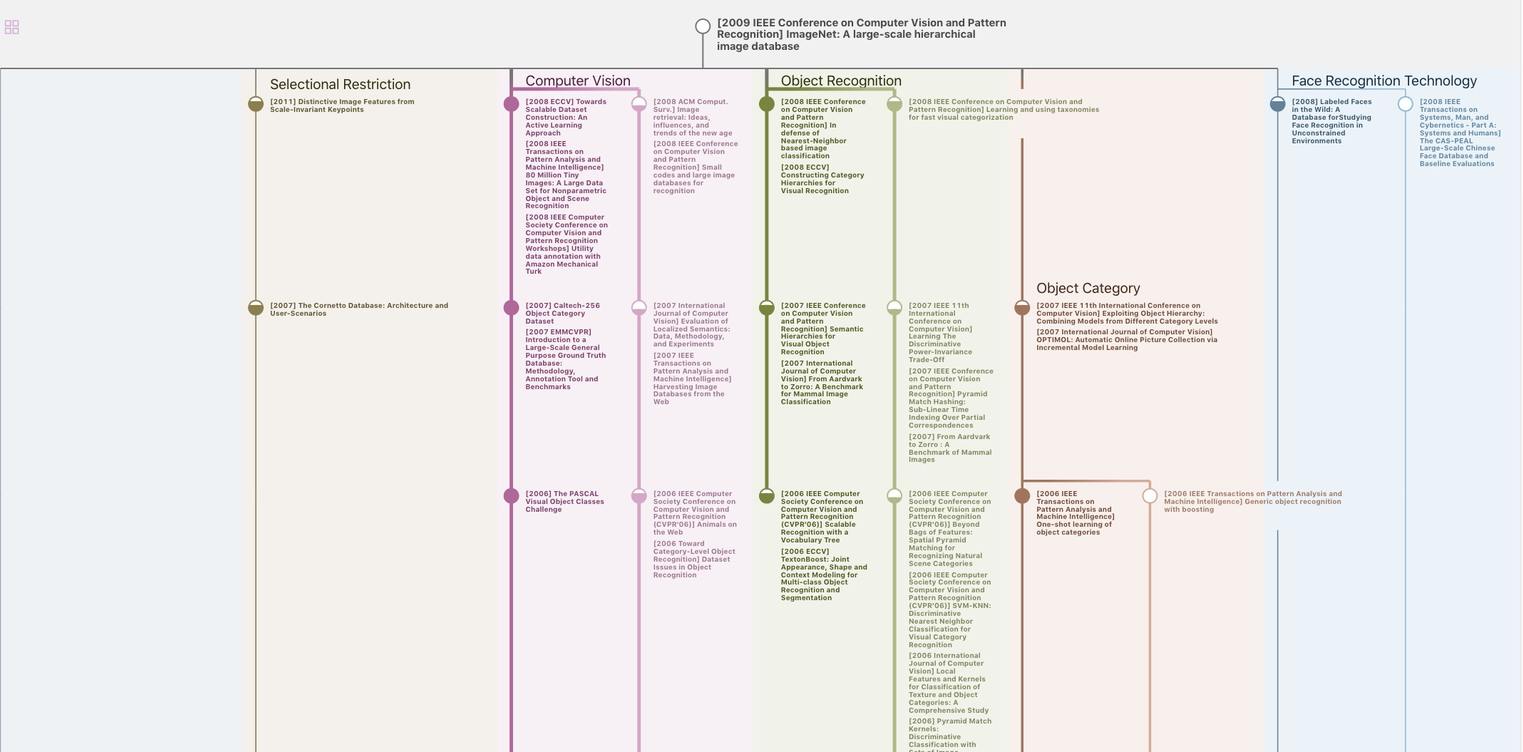
生成溯源树,研究论文发展脉络
Chat Paper
正在生成论文摘要