Convergence-Aware Neural Network Training
PROCEEDINGS OF THE 2020 57TH ACM/EDAC/IEEE DESIGN AUTOMATION CONFERENCE (DAC)(2020)
摘要
Training a deep neural network (DNN) is expensive, requiring a large amount of computation time. While the training overhead is high, not all computation in DNN training is equal. Some parameters converge faster and thus their gradient computation may contribute little to the parameter update; in near-stationary points a subset of parameters may change very little. In this paper we exploit the parameter convergence to optimize gradient computation in DNN training. We design a light-weight monitoring technique to track the parameter convergence; we prune the gradient computation stochastically for a group of semantically related parameters, exploiting their convergence correlations. These techniques are efficiently implemented in existing GPU kernels. In our evaluation the optimization techniques substantially and robustly improve the training throughput for four DNN models on three public datasets.
更多查看译文
关键词
convergence-aware neural network training,computation time,training overhead,DNN training,gradient computation,parameter update,parameter convergence,light-weight monitoring technique,semantically related parameters,convergence correlations,DNN models,public datasets
AI 理解论文
溯源树
样例
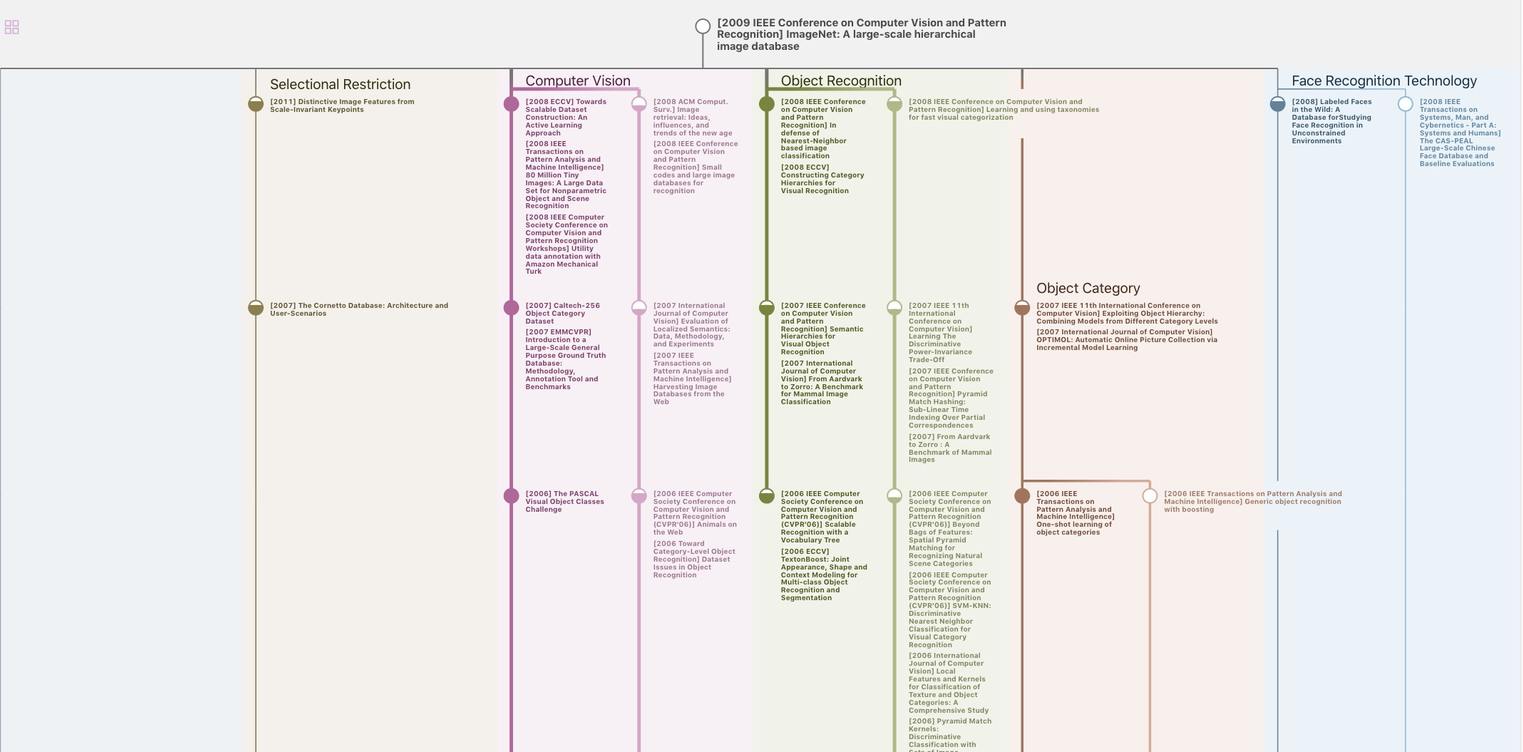
生成溯源树,研究论文发展脉络
Chat Paper
正在生成论文摘要