An Efficient Deep Learning Accelerator For Compressed Video Analysis
PROCEEDINGS OF THE 2020 57TH ACM/EDAC/IEEE DESIGN AUTOMATION CONFERENCE (DAC)(2020)
摘要
Previous neural network accelerators tailored to video analysis only accept data of RGB/YUV domain, requiring decompressing the video that are often compressed before transmitted from the edge sensors. A compressed video processing accelerator can remove the decoding overhead, and gain performance speedup by operating on more compact input data. This work proposes a novel deep learning accelerator architecture, Alchemist, which predicts results directly from the compressed video bitstream instead of reconstructing the full RGB images. By utilizing the metadata of motion vector and critical blocks extracted from bitstream, Alchemist contributes to remarkable performance speedup of 5x with negligible accuracy loss.
更多查看译文
关键词
deep learning accelerator,compressed video analysis,neural network accelerators,edge sensors,compressed video processing accelerator,decoding overhead,compact input data,deep learning accelerator architecture,Alchemist,compressed video bitstream,RGB images
AI 理解论文
溯源树
样例
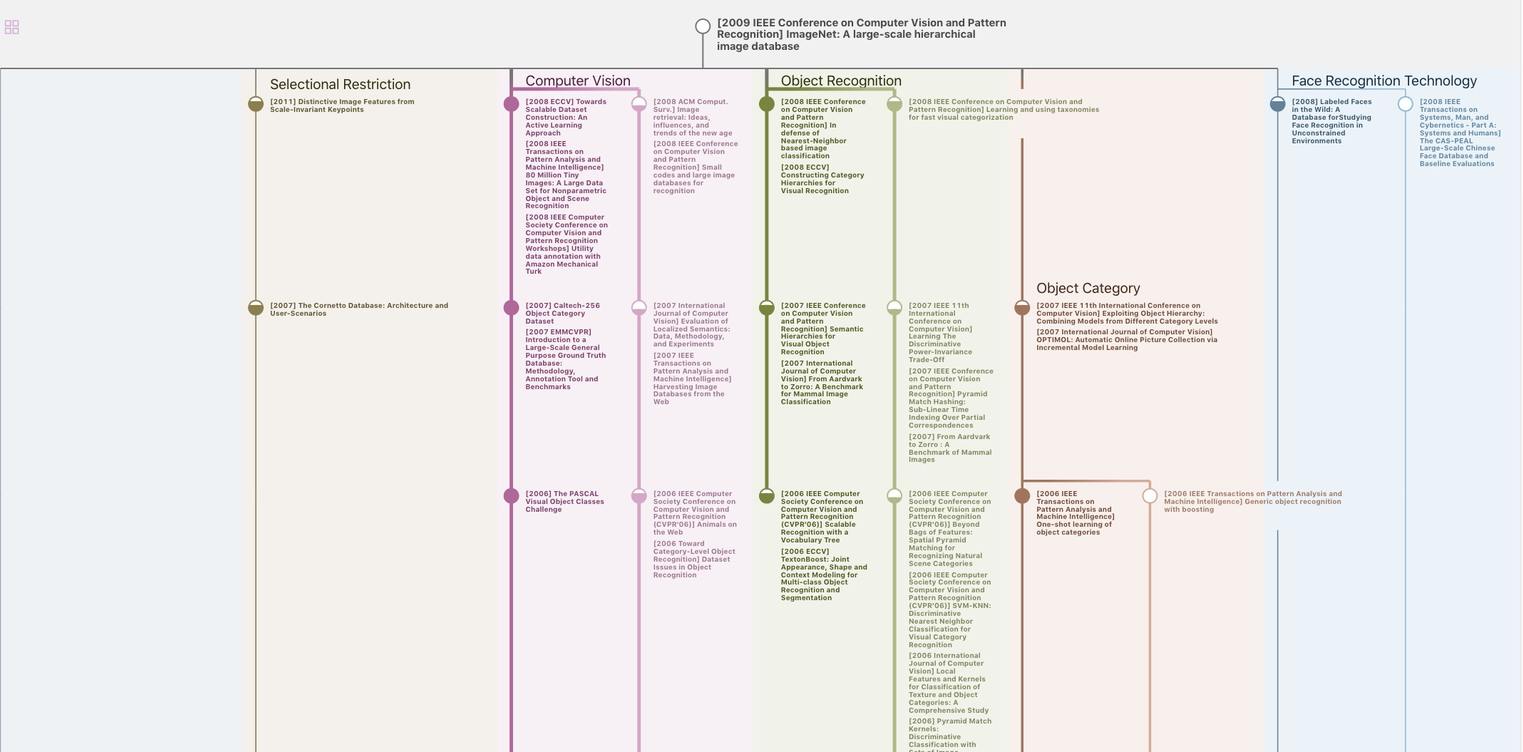
生成溯源树,研究论文发展脉络
Chat Paper
正在生成论文摘要