Scalable Taxonomy Generation and Evolution on Apache Spark
DASC/PiCom/CBDCom/CyberSciTech(2020)
摘要
Big data mainly refers to a huge volume of rapidly growing data over size exabytes (1018). A major chunk of this data is unstructured text data produced from several sources. In order to use such data effectively, they need to be processed and organized. Taxonomy, a hierarchical structure, is considered an effective way of organizing the data. In the past, many techniques have been proposed to generate taxonomy automatically. Recently some attempts have also been made to evolve the static structure of taxonomy to deal with the rapidly changing nature of data. However, the voluminous nature of today's data currently exceeds the processing capabilities of conventional techniques. In this regard, there is a need for a scalable technique that potentially speeds up the process of taxonomy generation and evolution and caters to a large amount of unstructured big data. This paper presents a technique for both the generation and the evolution of taxonomy on the Apache Spark framework. The technique is tested on a text dataset belonging to a computing domain. The test results show that the scalable taxonomy generation and evolution technique proposed in this paper is not only timeefficient but also produces a good quality taxonomy as compared to state-of-the-art techniques.
更多查看译文
关键词
Big Data,Apache Spark,Scalable Taxonomy Generation,Scalable Taxonomy Evolution,Unstructured Data
AI 理解论文
溯源树
样例
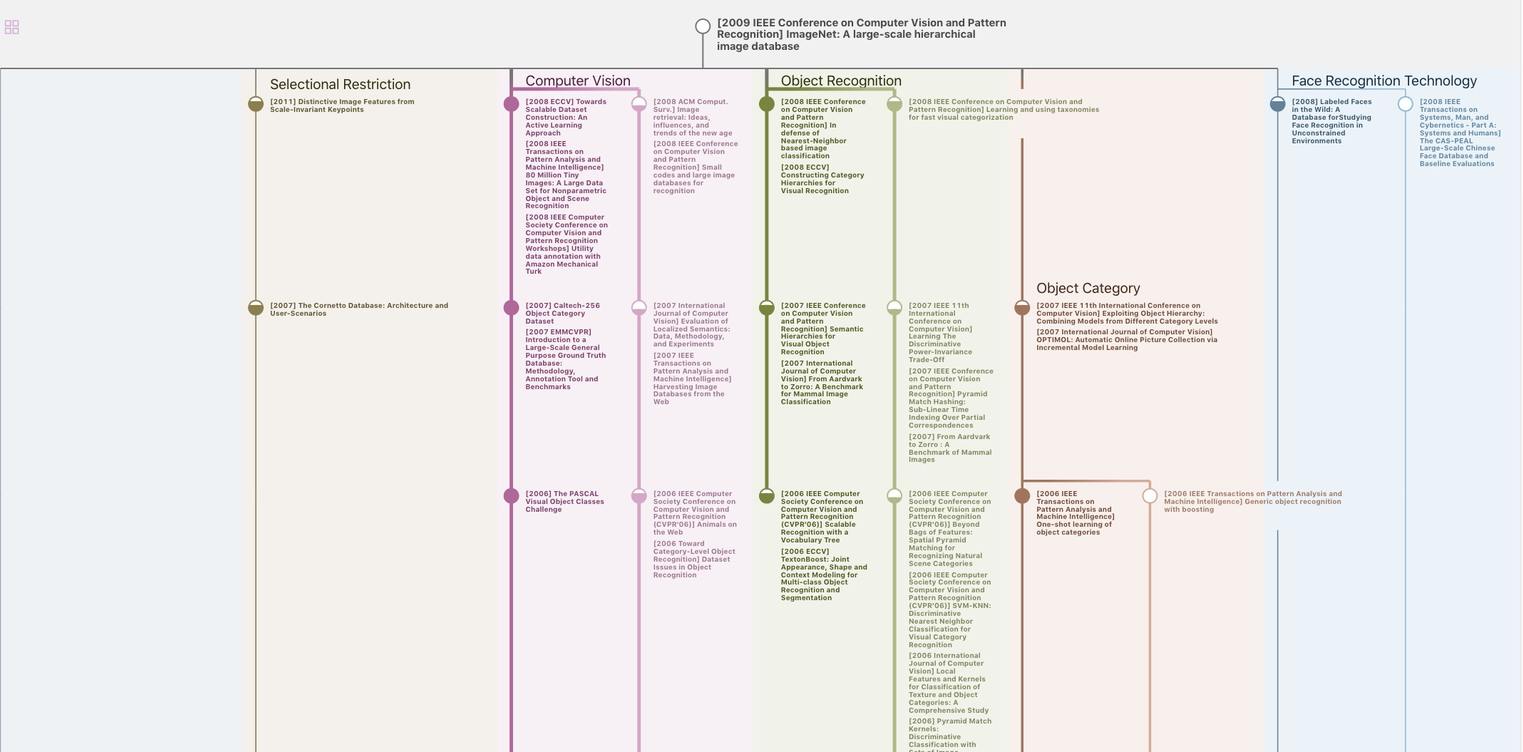
生成溯源树,研究论文发展脉络
Chat Paper
正在生成论文摘要