POSIT: simultaneously tagging natural and programming languages
International Conference on Software Engineering(2020)
摘要
ABSTRACTSoftware developers use a mix of source code and natural language text to communicate with each other: Stack Overflow and Developer mailing lists abound with this mixed text. Tagging this mixed text is essential for making progress on two seminal software engineering problems --- traceability, and reuse via precise extraction of code snippets from mixed text. In this paper, we borrow code-switching techniques from Natural Language Processing and adapt them to apply to mixed text to solve two problems: language identification and token tagging. Our technique, POSIT, simultaneously provides abstract syntax tree tags for source code tokens, part-of-speech tags for natural language words, and predicts the source language of a token in mixed text. To realize POSIT, we trained a biLSTM network with a Conditional Random Field output layer using abstract syntax tree tags from the CLANG compiler and part-of-speech tags from the Standard Stanford part-of-speech tagger. POSIT improves the state-of-the-art on language identification by 10.6% and PoS/AST tagging by 23.7% in accuracy.
更多查看译文
关键词
part-of-speech Tagging, Mixed-Code, Code-Switching, Language Identification
AI 理解论文
溯源树
样例
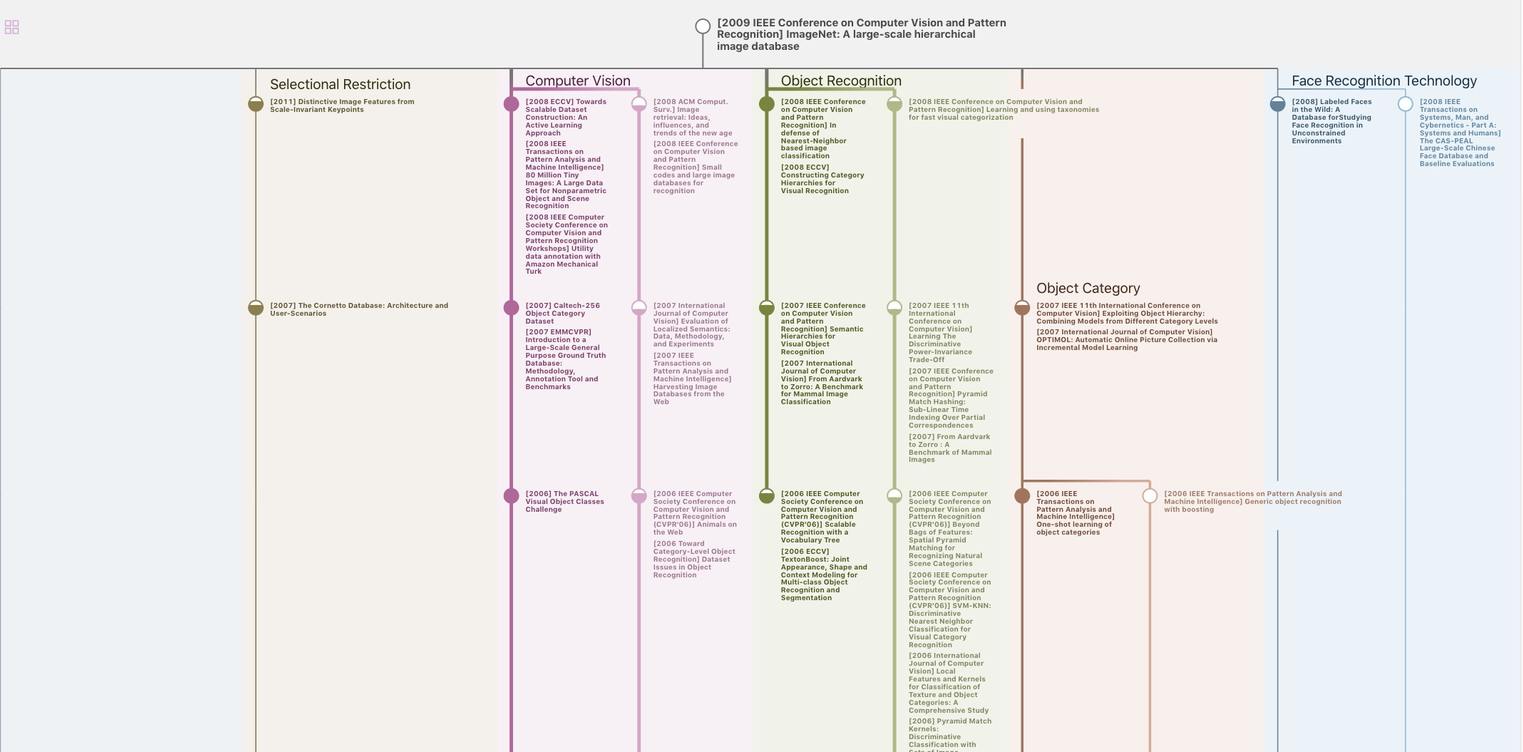
生成溯源树,研究论文发展脉络
Chat Paper
正在生成论文摘要