An Experimental Study on Applying Metamorphic Testing in Machine Learning Applications.
SAST(2020)
摘要
Machine learning techniques have been successfully employed in various areas and, in particular, for the development of healthcare applications, aiming to support in more effective and faster diagnostics (such as cancer diagnosis). However, machine learning models may present uncertainties and errors. Errors in the training process, classification, and evaluation can generate incorrect results and, consequently, to wrong clinical decisions, reducing the professionals' confidence in the use of such techniques. Similar to other application domains, the quality should be guaranteed to produce more reliable models capable of assisting health professionals in their daily activities. Metamorphic testing can be an interesting option to validate machine learning applications. Using this testing approach is possible to define relationships that define changes to be made in the application's input data to identify faults. This paper presents an experimental study to evaluate the effectiveness of metamorphic testing to validate machine learning applications. A Machine learning application to verify breast cancer diagnostic was developed, using an available dataset composed of 569 samples whose data were taken from breast cancer images, and used as the software under test, in which the metamorphic testing was applied. The results indicate that metamorphic testing can be an alternative to support the validation of machine learning applications.
更多查看译文
AI 理解论文
溯源树
样例
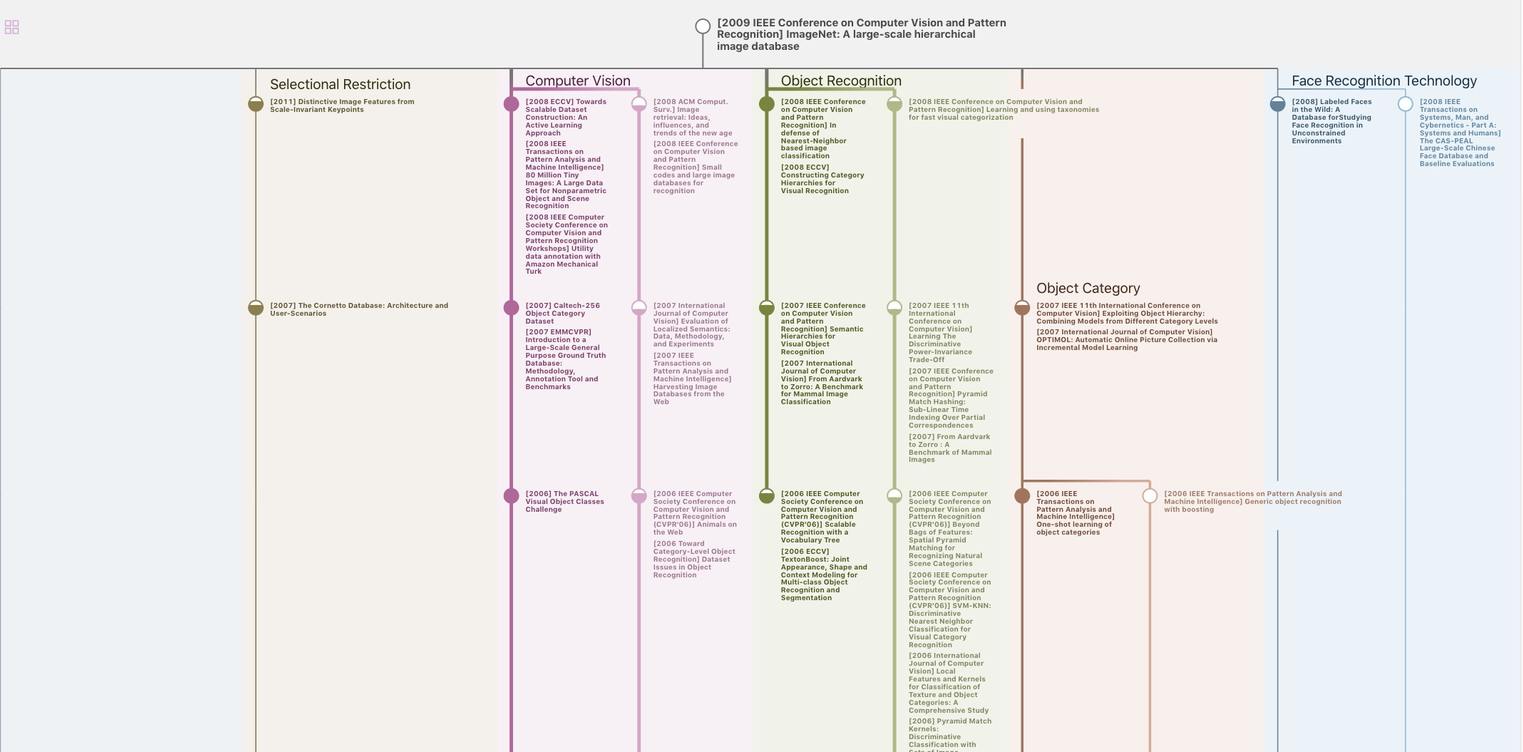
生成溯源树,研究论文发展脉络
Chat Paper
正在生成论文摘要