Scalable Machine Learning on High-Dimensional Vectors - From Data Series to Deep Network Embeddings.
WIMS(2020)
摘要
There is an increasingly pressing need, by several applications in diverse domains, for developing techniques able to analyze very large collections of static and streaming sequences (a.k.a. data series), predominantly in real-time. Examples of such applications come from Internet of Things installations, neuroscience, astrophysics, and a multitude of other scientific and application domains that need to apply machine learning techniques for knowledge extraction. It is not unusual for these applications, for which similarity search is a core operation, to involve numbers of data series in the order of hundreds of millions to billions, which are seldom analyzed in their full detail due to their sheer size. Such application requirements have driven the development of novel similarity search methods that can facilitate scalable analytics in this context. At the same time, a host of other methods have been developed for similarity search of high-dimensional vectors in general. All these methods are now becoming increasingly important, because of the growing popularity and size of sequence collections, as well as the growing use of high-dimensional vector representations of a large variety of objects (such as text, multimedia, images, audio and video recordings, graphs, database tables, and others) thanks to deep network embeddings. In this work, we review recent efforts in designing techniques for indexing and analyzing massive collections of data series, and argue that they are the methods of choice even for general high-dimensional vectors. Finally, we discuss the challenges and open research problems in this area.
更多查看译文
AI 理解论文
溯源树
样例
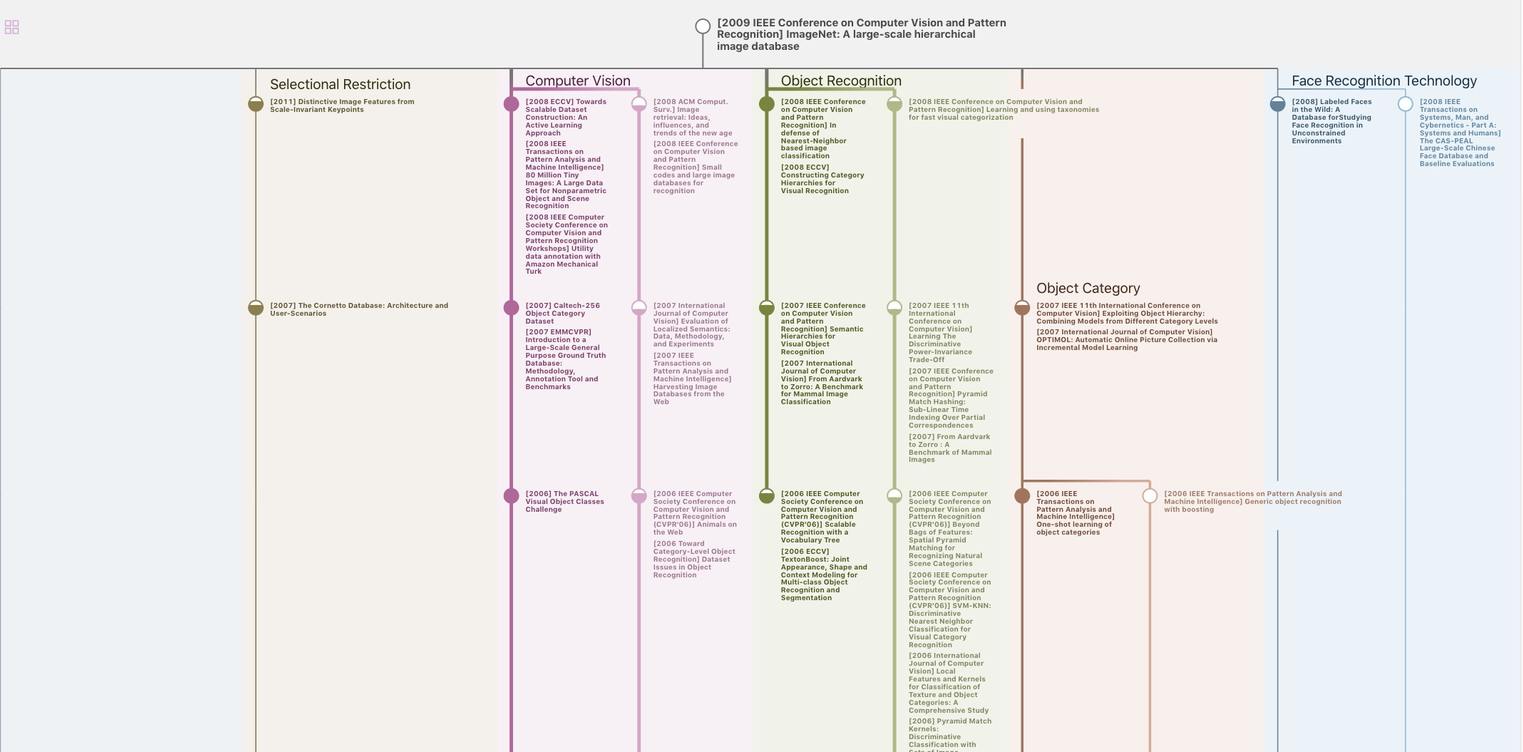
生成溯源树,研究论文发展脉络
Chat Paper
正在生成论文摘要