Understanding the Behavior of Reinforcement Learning Agents.
BIOMA(2020)
摘要
Reinforcement Learning (RL) is the process of training agents to solve specific tasks, based on measures of reward. Understanding the behavior of an agent in its environment can be crucial. For instance, if users understand why specific agents fail at a task, they might be able to define better reward functions, to steer the agents’ development in the right direction. Understandability also empowers decisions for agent deployment. If we know why the controller of an autonomous car fails or excels in specific traffic situations, we can make better decisions on whether/when to use them in practice. We aim to facilitate the understandability of RL. To that end, we investigate and observe the behavioral space: the set of actions of an agent observed for a set of input states. Consecutively, we develop measures of distance or similarity in that space and analyze how agents compare in their behavior. Moreover, we investigate which states and actions are critical for a task, and determine the correlation between reward and behavior. We utilize two basic RL environments to investigate our measures. The results showcase the high potential of inspecting an agents’ behavior and comparing their distance in behavior space.
更多查看译文
关键词
reinforcement learning agents,behavior
AI 理解论文
溯源树
样例
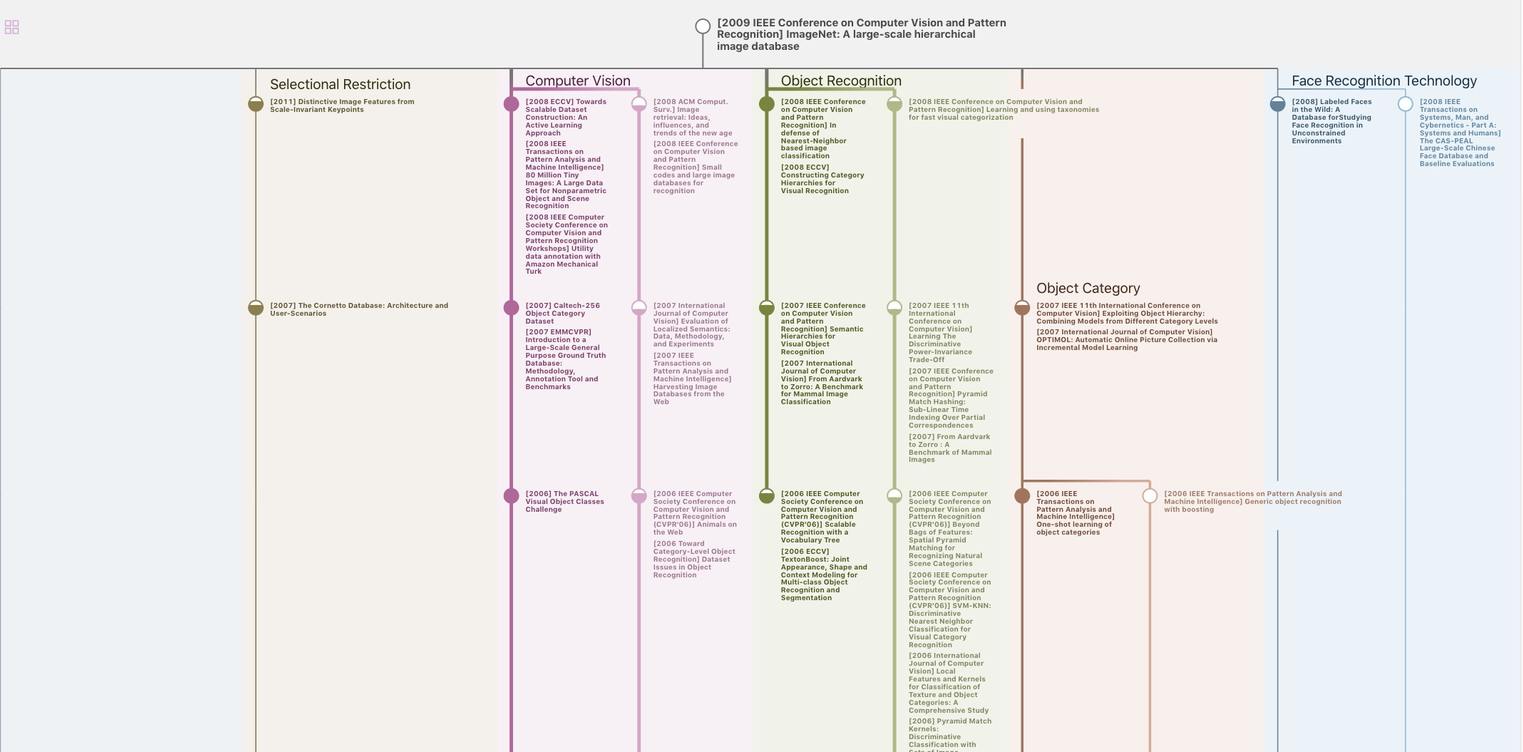
生成溯源树,研究论文发展脉络
Chat Paper
正在生成论文摘要