Blind Speech Signal Quality Estimation for Speaker Verification Systems.
INTERSPEECH(2020)
摘要
The problem of system performance degradation in mismatched acoustic conditions has been widely acknowledged in the community and is common for different fields. The present state-of-the-art deep speaker embedding models are domain-sensitive. The main idea of the current research is to develop a single method for automatic signal quality estimation, which allows to evaluate short-term signal characteristics. This paper presents a neural network based approach for blind speech signal quality estimation in terms of signal-to-noise ratio (SNR) and reverberation time (RT60), which is able to classify the type of underlying additive noise. Additionally, current research revealed the need for an accurate voice activity detector that performs well in both clean and noisy unseen environments. Therefore a novel neural network VAD based on U-net architecture is presented.The proposed algorithms allow to perform the analysis of NIST, SITW, Voices datasets commonly used for objective comparison of speaker verification systems from the new point of view and consider effective calibration steps to improve speaker recognition quality on them.
更多查看译文
关键词
Blind speech quality estimation, SNR, RT60, VAD, speaker recognition
AI 理解论文
溯源树
样例
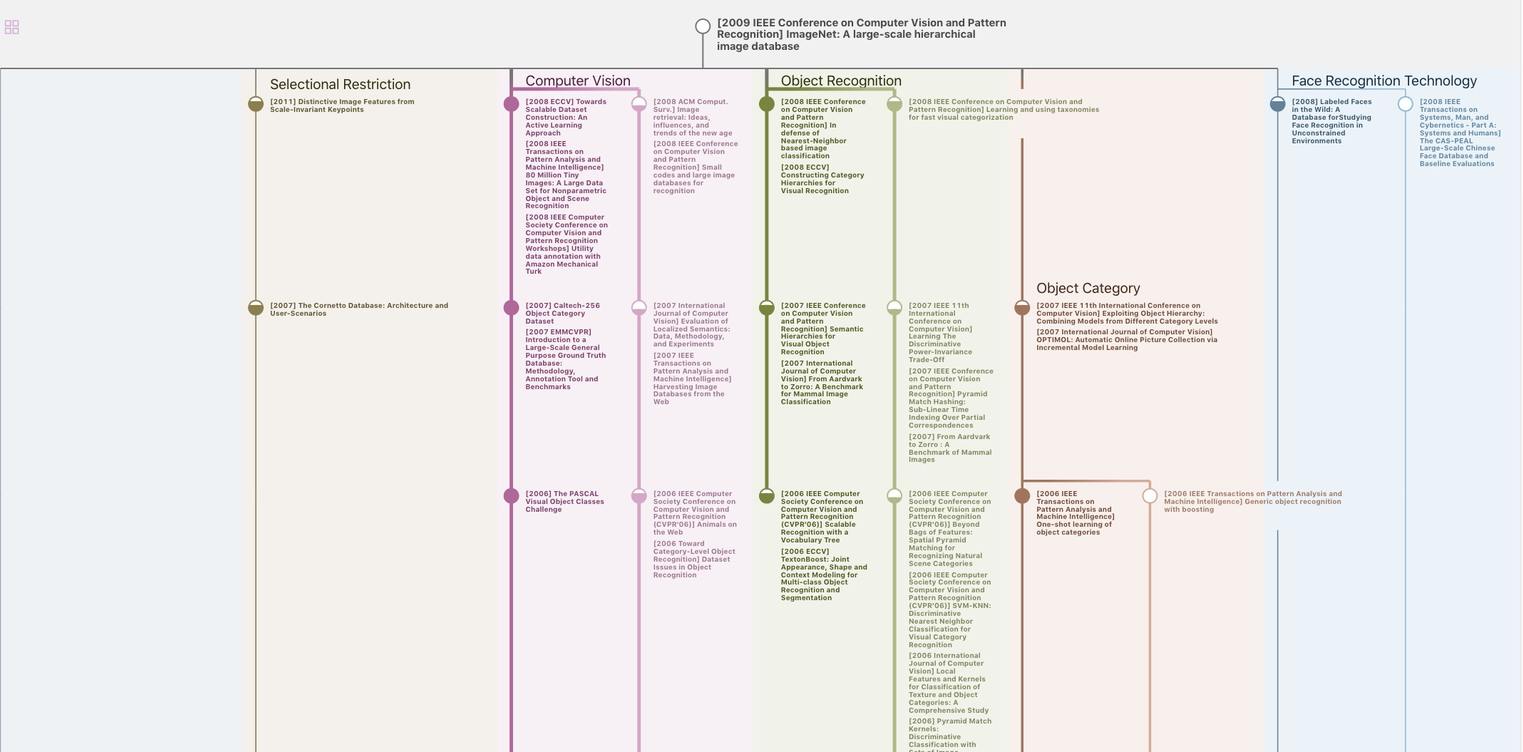
生成溯源树,研究论文发展脉络
Chat Paper
正在生成论文摘要