Machine-Learning Based Nonlinerity Correction for Coarse-Fine SAR-TDC Hybrid ADC
2020 IEEE 63rd International Midwest Symposium on Circuits and Systems (MWSCAS)(2020)
摘要
This paper presents a backend machine learning-based nonlinearity calibration scheme for a coarse-fine two stage SAR-TDC hybrid ADC. Different from conventional approaches, the machine learning-based nonlinearity calibration scheme avoids the on-chip pseudonumber (PN) generator or complex, specific matrix operations in the digital domain backend process. The scheme utilizes a two-layer neural network to extract and compensate the bit-weight error caused by circuit nonlinearities such as inter-stage gain error or time-to-digital converter (TDC) delay cell mismatch. The neural network uses the ADC DNL and INL testing results as training data, thus avoiding additional reference channel or a split ADC structure. A 10-bit 500 MS/s coarse-fine SAR-TDC ADC is designed in 22nm FDSOI technology to validate the scheme. The simulation results show the ADC achieves an SNDR of 57 dB, SFDR of 71.3 dB, and an ENOB of 9.18 bits, corresponding to a Walden FOM of 5.2 fJ/conv.-step after backend nonlinearity calibration.
更多查看译文
关键词
Machine learning-based nonlinearity correction,Coarse-fine ADC architecture,SAR ADC,Subrange TDC
AI 理解论文
溯源树
样例
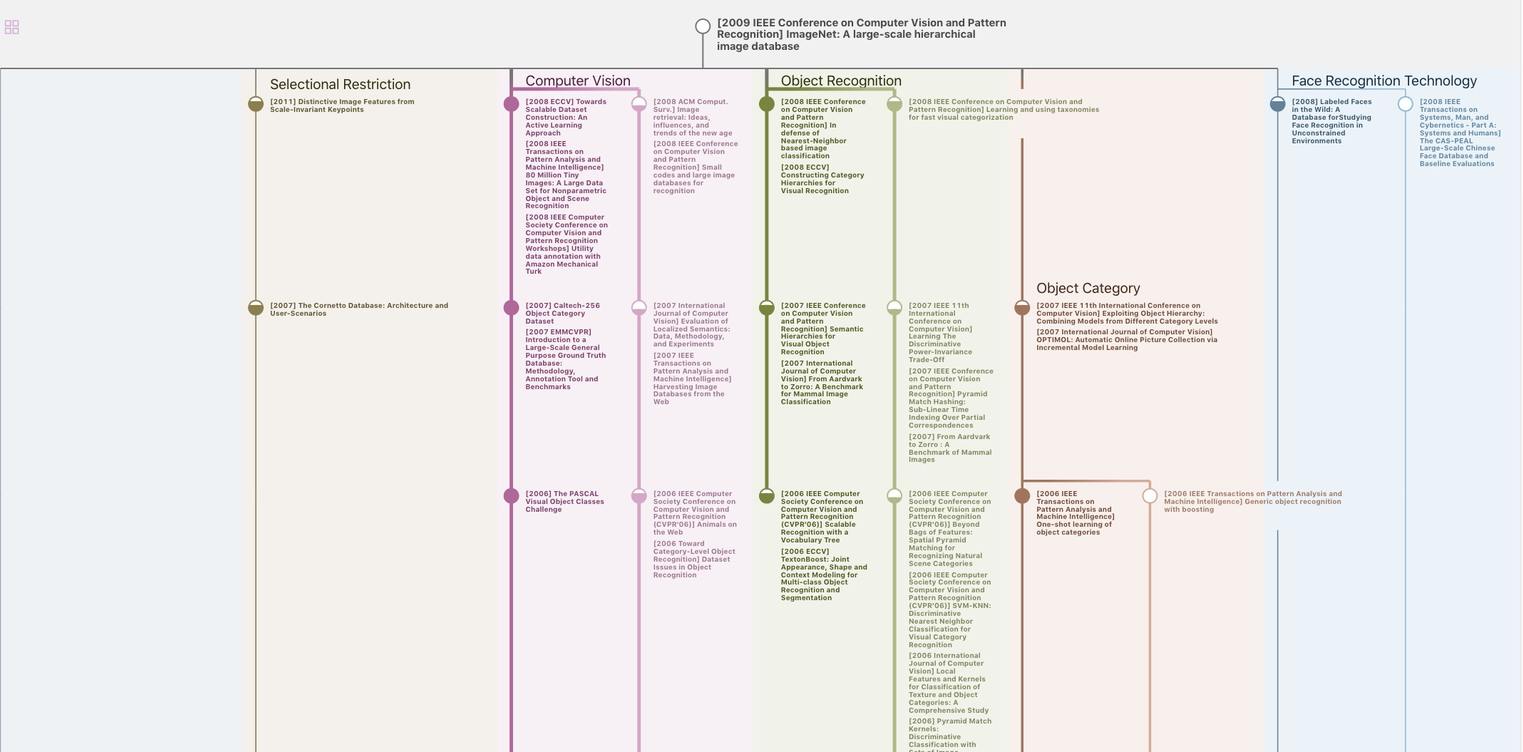
生成溯源树,研究论文发展脉络
Chat Paper
正在生成论文摘要