Causal Strategic Linear Regression.
ICML(2020)
摘要
In many predictive decision-making scenarios, such as credit scoring and academic testing, a decision-maker must construct a model that accounts for agents’ propensity to “game” the decision rule by changing their features so as to receive better decisions. Whereas the strategic classification literature has previously assumed that agents’ outcomes are not causally affected by their features (and thus that strategic agents’ goal is deceiving the decision-maker), we join concurrent work in modeling agents’ outcomes as a function of their changeable attributes. As our main contribution, we provide efficient algorithms for learning decision rules that optimize three distinct decision-maker objectives in a realizable linear setting: accurately predicting agents’ post-gaming outcomes (prediction risk minimization), incentivizing agents to improve these outcomes (agent outcome maximization), and estimating the coefficients of the true underlying model (parameter estimation). Our algorithms circumvent a hardness result of Miller et al. (2019) by allowing the decision maker to test a sequence of decision rules and observe agents’ responses, in effect performing causal interventions through the decision rules.
更多查看译文
关键词
regression,linear
AI 理解论文
溯源树
样例
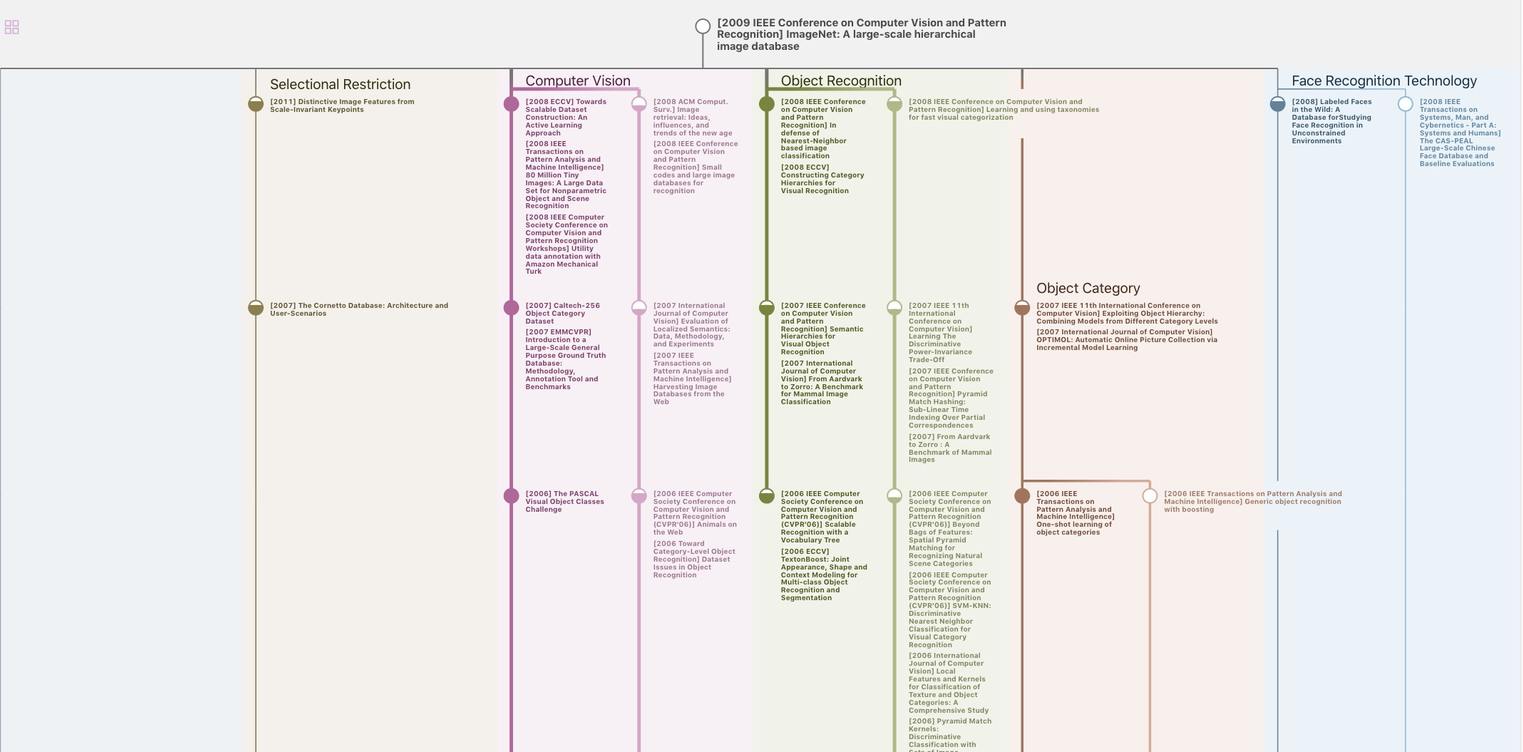
生成溯源树,研究论文发展脉络
Chat Paper
正在生成论文摘要