On Nonnegative CP Tensor Decomposition Robustness to Noise
2020 Information Theory and Applications Workshop (ITA)(2020)
摘要
In today's data-driven world, there is an unprecedented demand for large-scale temporal data analysis. Dynamic topic modeling has been widely used in social and data sciences with the goal of learning latent topics that emerge, evolve, and fade over time. Previous work on dynamic topic modeling primarily employ the method of nonnegative matrix factorization (NMF), where slices of the data tensor are each factorized into the product of lower dimensional nonnegative matrices. With this approach, however, noise can have devastating effects on the learned latent topics and obscure the true topics in the data. To overcome this issue, we propose instead adopting the method of nonnegative CANDECOMP/PARAFAC (CP) tensor decomposition (NNCPD), where the data tensor is directly decomposed into a minimal sum of outer products of nonnegative vectors. We show experimental evidence that suggests that NNCPD is robust to noise in the data when one overestimates the CP rank of the tensor.
更多查看译文
关键词
learned latent topics,data tensor,nonnegative vectors,nonnegative CP tensor decomposition,temporal data analysis,dynamic topic modeling,nonnegative matrix factorization,CANDECOMP/PARAFAC tensor decomposition,big data analysis
AI 理解论文
溯源树
样例
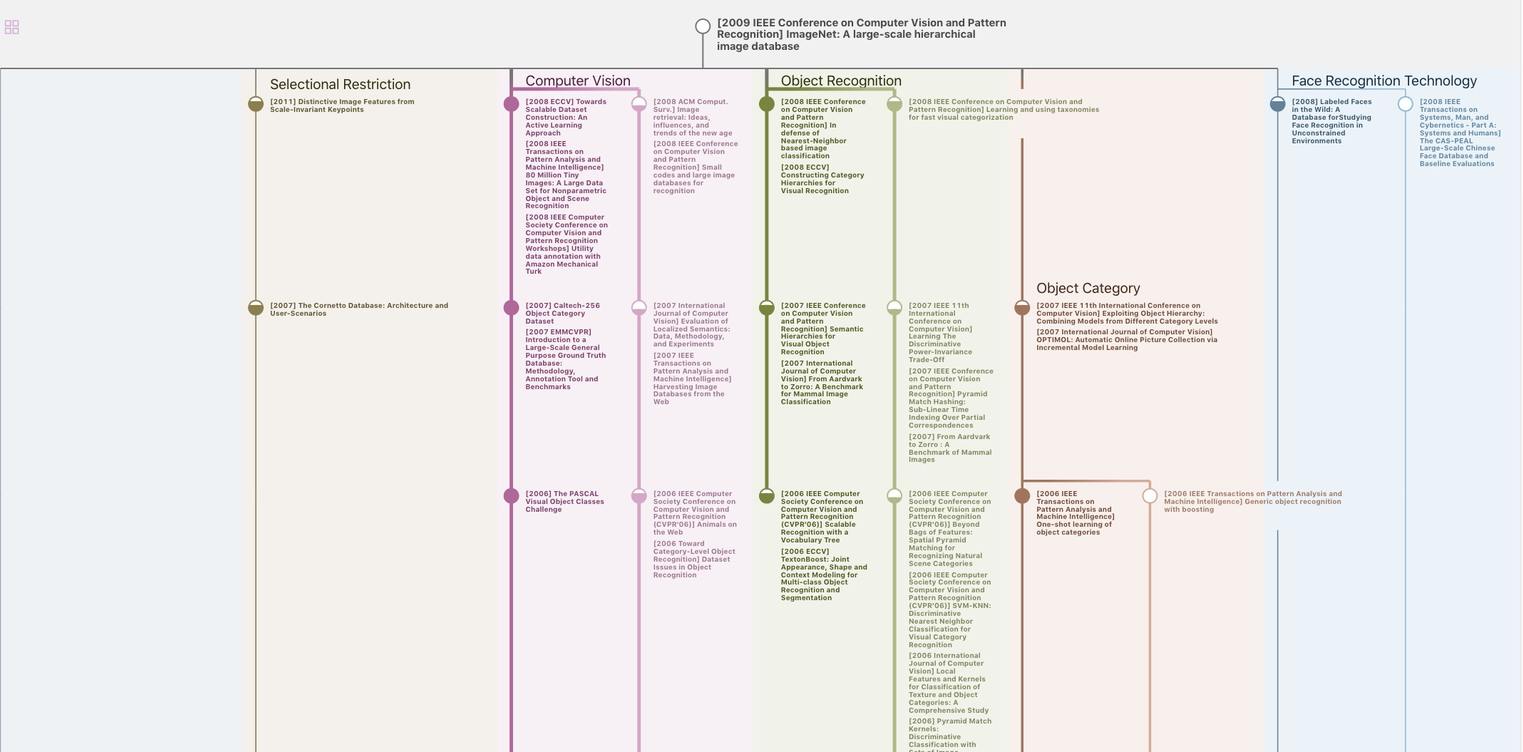
生成溯源树,研究论文发展脉络
Chat Paper
正在生成论文摘要