On the suitability of Data Selection for Cross-building Knowledge Transfer.
HPCS(2019)
摘要
Supervised deep learning has achieved remarkable success in various applications. Such advances were mainly attributed to the rise of computational powers and the amounts of training data made available. Therefore, accurate large-scale data collection services are often needed. Once representative data is retrieved, it becomes possible to train the supervised machine learning predictor. However, a model trained on existing data, that generally comes from multiple datasets, might generalize poorly on the unseen target data. This problem is referred to as domain shift. In this paper, we explore the suitability of data selection to tackle the domain shift challenge in the context of domain generalization. We perform our experimental study on the use case of building energy consumption prediction. Experimental results suggest that minimal building description is capable of improving cross-building generalization performances when used to select data.
更多查看译文
关键词
Data selection,domain generalization,knowledge transfer,data-driven modeling,energy consumption modeling
AI 理解论文
溯源树
样例
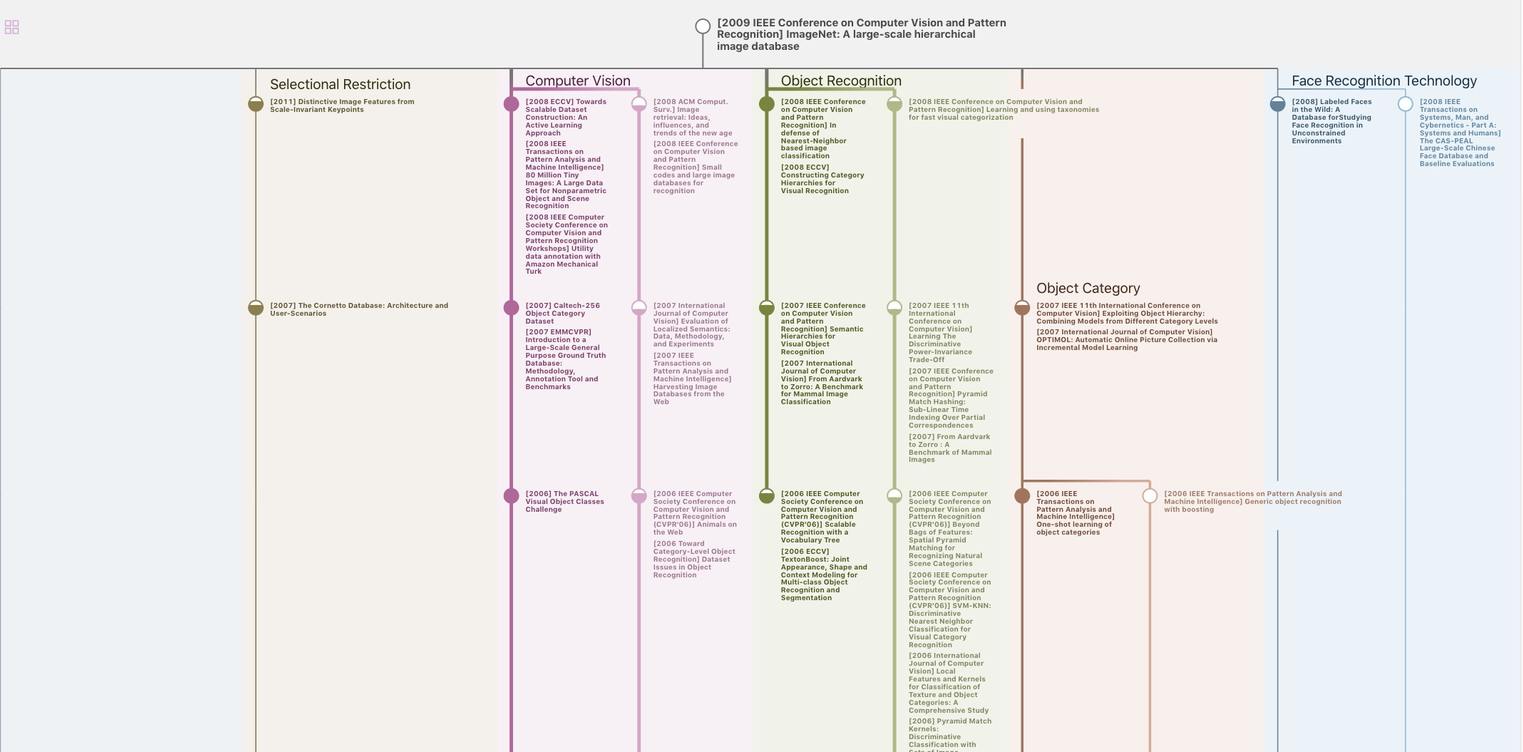
生成溯源树,研究论文发展脉络
Chat Paper
正在生成论文摘要