Behavior Based Dynamic Summarization On Product Aspects Via Reinforcement Neighbour Selection
ECAI 2020: 24TH EUROPEAN CONFERENCE ON ARTIFICIAL INTELLIGENCE(2020)
摘要
Dynamic summarization on product aspects, as a newly proposed topic, is an important task in E-commerce for tracking and understanding the nature of products. This can benefit both customers and sellers in different downstream tasks, such as explainable recommendations. However, most existing research works focus on analyzing product static reviews but miss dynamic sentiment changes. In this paper, we propose an innovative multi-task model to sample neighbour products whose information is simultaneously utilized to generate product summarization. In detail, a reinforcement learning approach selects neighbour products from a group of seed products by considering their pairwise similarities calculated from user behaviors. Meanwhile, a generative model helps to summarize product aspects via product descriptive phrases and selected neighbour products' sentimental phrases. To the best of our knowledge, this is the first work that studies dynamic product summarization leveraging user behaviors instead of self-reviews. It means that the proposed approach can naturally address the cold-start scenario where few recent product reviews are available. Extensive experiments are conducted with real-world reviews plus behavior data to validate the proposed method against several strong alternatives.
更多查看译文
AI 理解论文
溯源树
样例
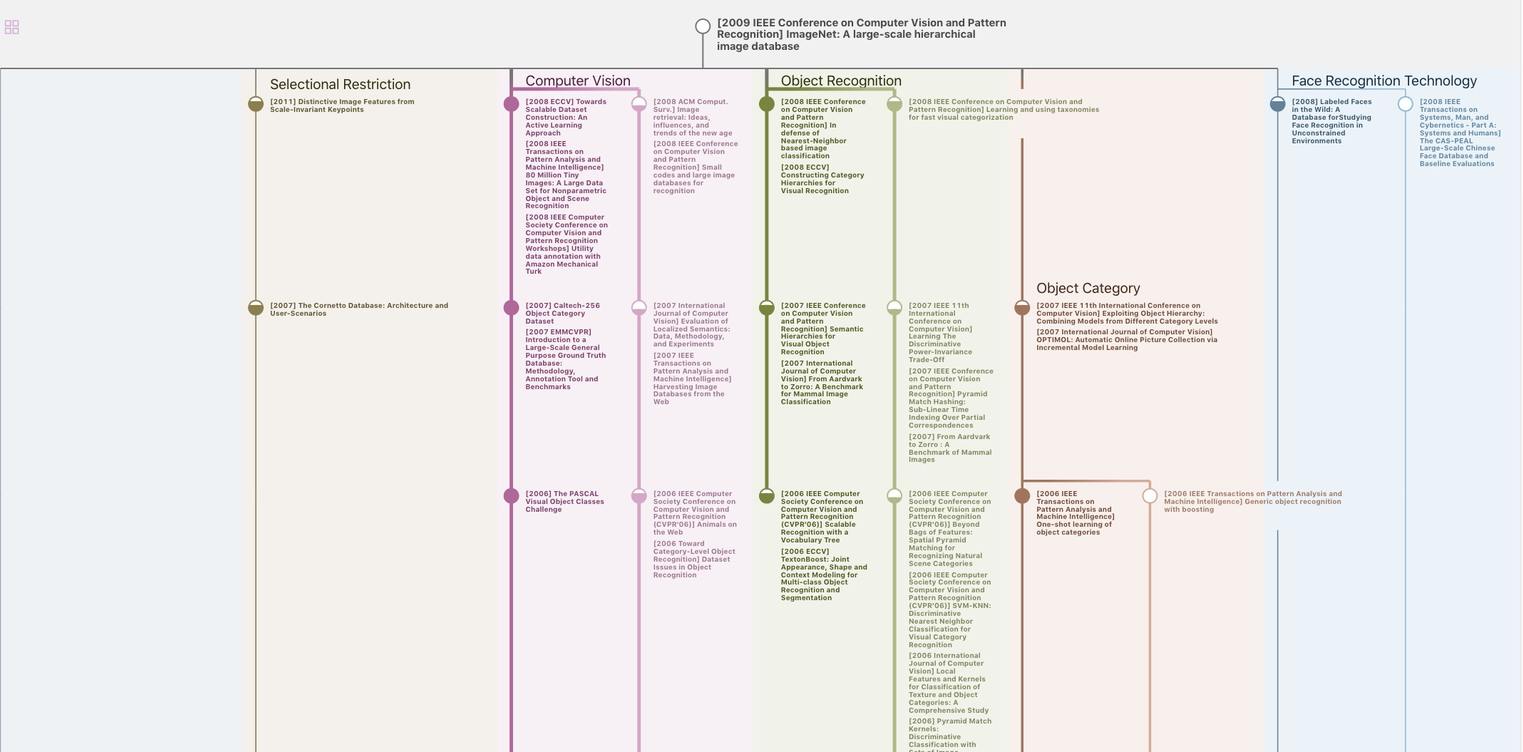
生成溯源树,研究论文发展脉络
Chat Paper
正在生成论文摘要