Incorporating Semantic Dependencies Extracted From Knowledge Graphs Into Joint Inference Template-Based Information Extraction
ECAI 2020: 24TH EUROPEAN CONFERENCE ON ARTIFICIAL INTELLIGENCE(2020)
摘要
In template-based information extraction, a template is described by a predefined set of slots that need to be filled with entities mentioned in a text. Compared to traditional relation extraction that aims at classifying binary relations involving a pair of entities only, template-based slot-filling is of higher complexity as interdependencies between slot-filler values need to be taken into account. To model these dependencies, we tackle the slot-filling task as a joint inference problem and build on factorized distributions as used in conditional random fields. Dependencies are often described by textual features only, but in some cases they are of semantic nature and not directly expressed in text. To exploit this potential, we propose the incorporation of semantic dependencies extracted from knowledge graphs into an information extraction model. Dependencies are extracted from the variable bindings of queries executed over a knowledge graph and capture semantic soft constraints that are weighted as part of model training. We evaluate our approach on five distantly supervised labeled datasets extracted from Wikipedia/DBpedia and compare our approach to a most frequent entity baseline as well as a purely textual model. We show that there is an overall positive impact of integrating factual background knowledge in all datasets, yielding an improvement of up to 10 points in F-1.
更多查看译文
AI 理解论文
溯源树
样例
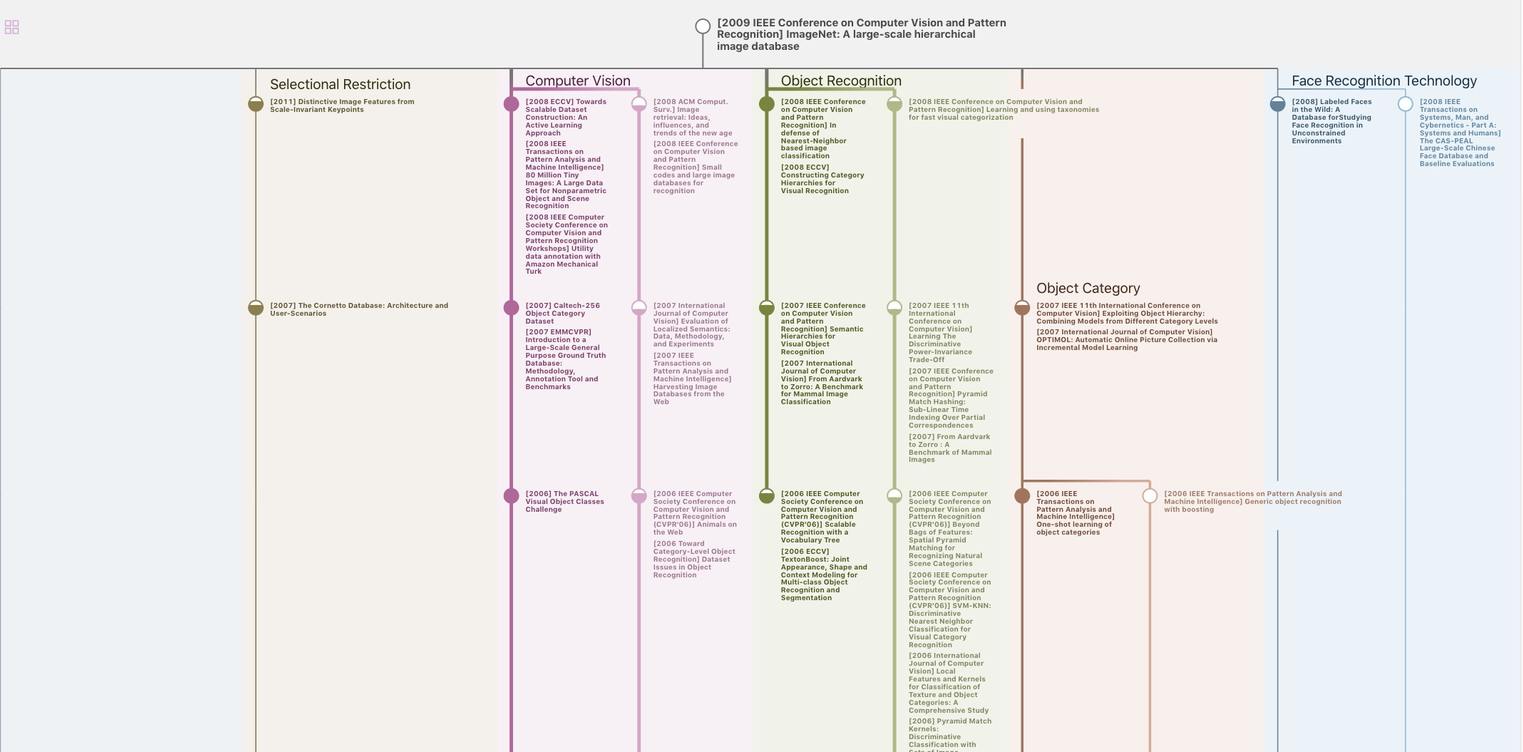
生成溯源树,研究论文发展脉络
Chat Paper
正在生成论文摘要