ST-MFM: A Spatiotemporal Multi-Modal Fusion Model for Urban Anomalies Prediction.
European Conference on Artificial Intelligence(2020)
摘要
Urban anomaly prediction is of great importance for urban management and public safety. Accurate anomaly prediction can avoid much unnecessary loss. Urban anomalies are usually caused by many complex factors, such as festivals, demonstrations and market promotions. It is not possible to predict anomalies from the perspective of reason, thus, most of the previous work analyzes the impacts of anomalies from multiple crowd flow datasets and observes the shift to ordinary distribution when they occur. Most existing models use observation-based methods to extract relevant spatiotemporal features, which are difficult to fully extract hidden relationships and eventually lead to low accuracy and low recall. In this paper, we propose an end-to-end deep learning based approach, called spatiotemporal multi-modal fusion model to collect the impacts of urban anomalies on multiple crowd flow datasets and predict anomalies in each region of the city for next time interval in turn. More specifically, we model the city into a graph and regard each region as a node. We use graph convolution network to obtain its spatial features and use gate recurrent units to obtain its temporal features. The features of those multiple modalities are further aggregated with points of interest in a two-stage-fusion method for assigning different weights to different functional regions. We evaluate our method using five datasets associated with New York City: 311 complaints, taxicab data, bike rental data, points of interest and road network dataset. Results show the advantages nearly 10% beyond the-state-of-the-art urban anomalies prediction methods.
更多查看译文
AI 理解论文
溯源树
样例
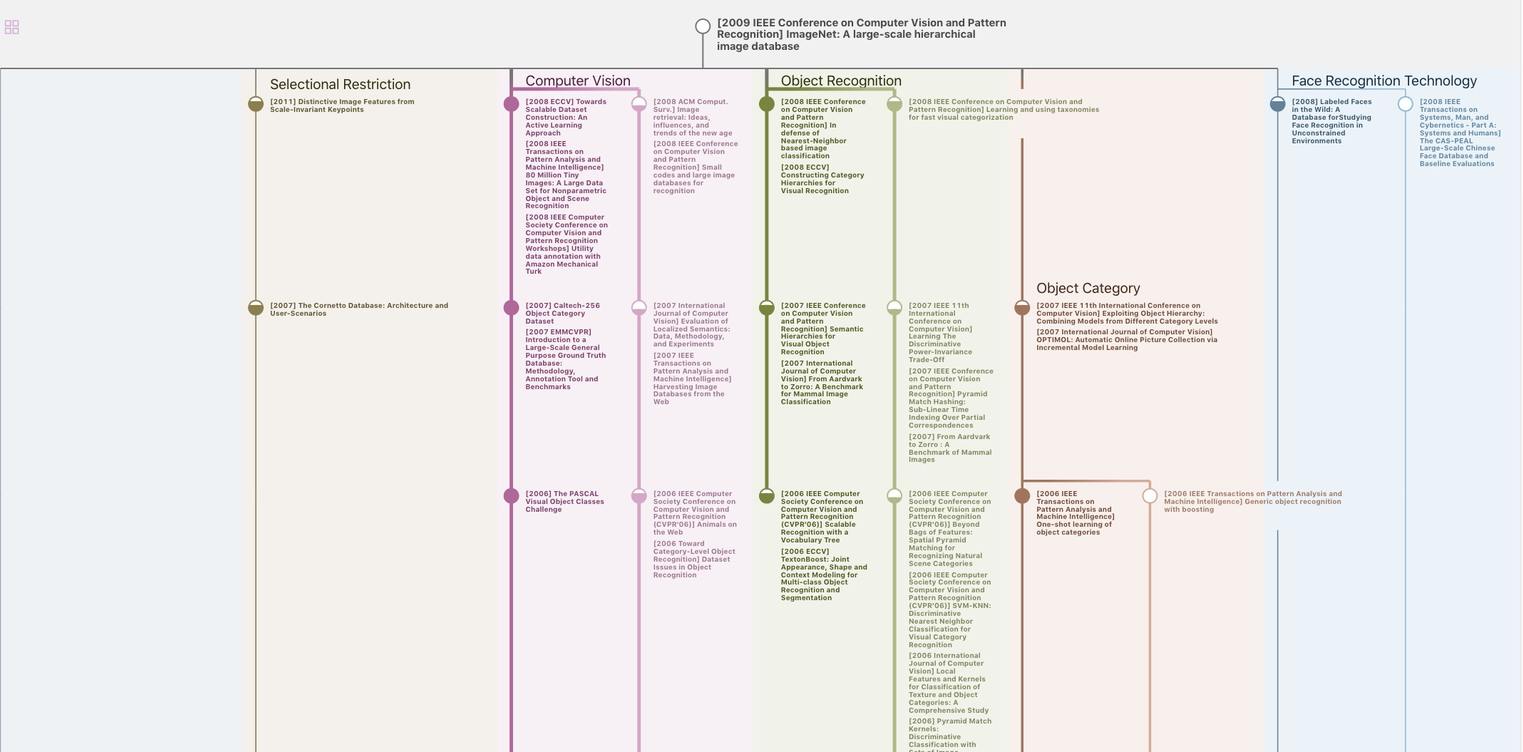
生成溯源树,研究论文发展脉络
Chat Paper
正在生成论文摘要