Belief Map Enhancement Network For Accurate Human Pose Estimation
ECAI 2020: 24TH EUROPEAN CONFERENCE ON ARTIFICIAL INTELLIGENCE(2020)
Abstract
It is a common practice for pose estimation models to output fixed-size low-resolution belief maps for the body keypoints. The coordinates of the highest belief location are then extracted for each of the body keypoints. When mapping this coarse-grained coordinates back into the fine-grained input space, a minor deviation from the ground-truth location will be magnified many times. So, we can usually get more accurate estimation by using larger belief maps. However, the problem is that we can not use too large belief maps due to the limited computational resources. To alleviate this problem, we propose the Belief Map Enhancement Network (EnhanceNet) for more accurate human pose estimation. EnhanceNet enlarges the belief maps by using the efficient sub-pixel operations, which not only increases the belief map resolution but also corrects some wrong predictions at the same time. Our EnhanceNet is simple yet effective. Extensive experiments are conducted on MPII and COCO datasets to verify the effectiveness of our proposed network. Specifically, we achieve consistently improvements on MPII dataset and COCO human pose dataset by applying our EnhanceNet to the state-of-the-art methods. Our EnhanceNet can be easily inserted into existing networks.
MoreTranslated text
Key words
belief map enhancement network,accurate human pose estimation
AI Read Science
Must-Reading Tree
Example
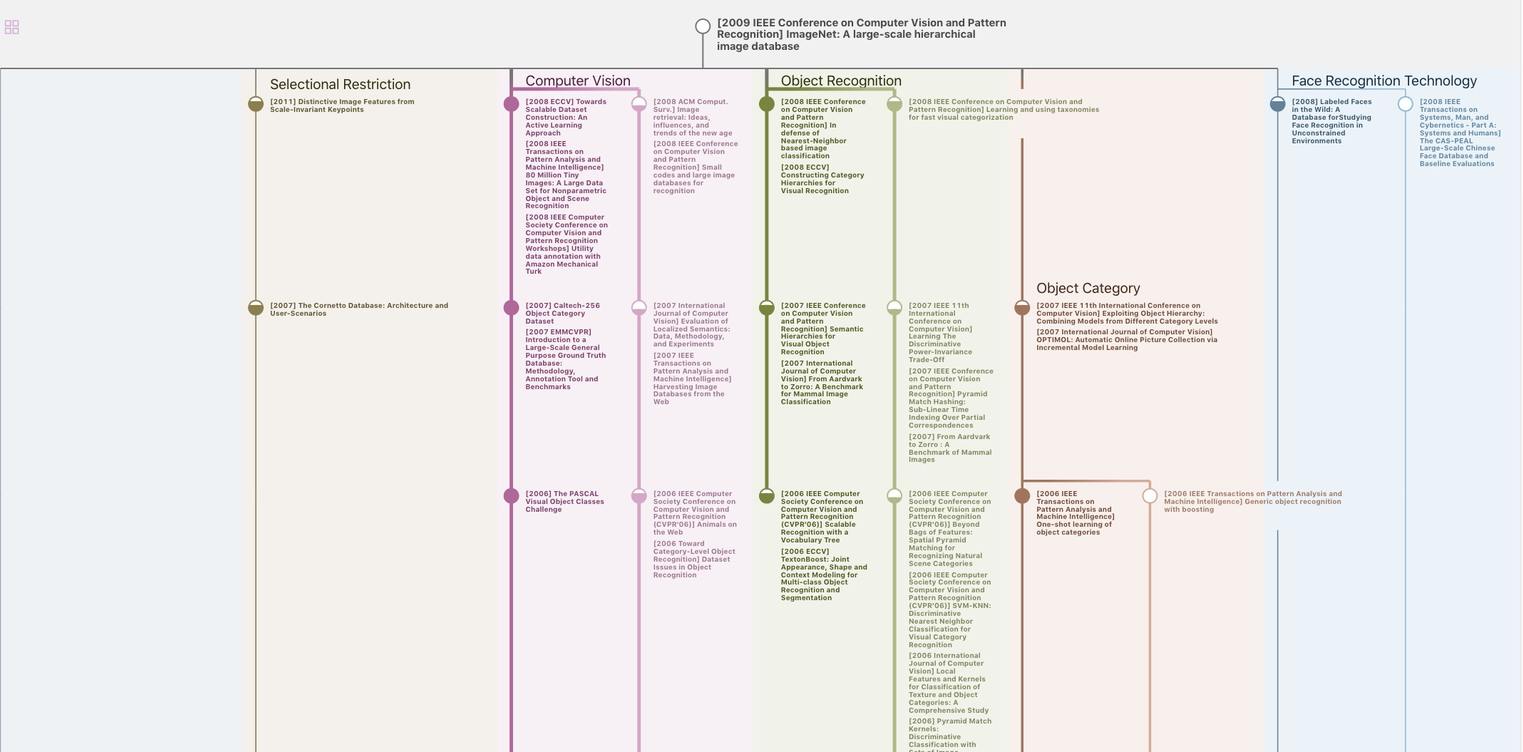
Generate MRT to find the research sequence of this paper
Chat Paper
Summary is being generated by the instructions you defined