Anomaly Detection Forest
ECAI 2020: 24TH EUROPEAN CONFERENCE ON ARTIFICIAL INTELLIGENCE(2020)
摘要
In many applications of anomaly detection, data with labeled anomalies may not be readily available. When designing a system for detection of malicious network activity, for instance, comprehensive malicious data can not be obtained since attacks constantly evolve and hence change their pattern. In such cases, an automatic anomaly detection system needs, at least partly, to rely on one-class learning, where training data exclusively contains normal instances. In this paper, we present a new anomaly detection algorithm, the Anomaly Detection Forest, optimized for the one-class learning problem. The algorithm is an ensemble of binary trees, where each tree is trained on a random subset and where the location of empty leaves define the anomaly score attributed to a data point. Our experimental results on a set with 14 public datasets show that the new algorithm outperforms the state-of-the-art algorithms Isolation Forest and One-Class Random Forest for the task of anomaly detection in the one-class learning setting.
更多查看译文
关键词
Anomaly Detection,Outlier Detection,Botnet Detection,Intrusion Detection,Novelty Detection
AI 理解论文
溯源树
样例
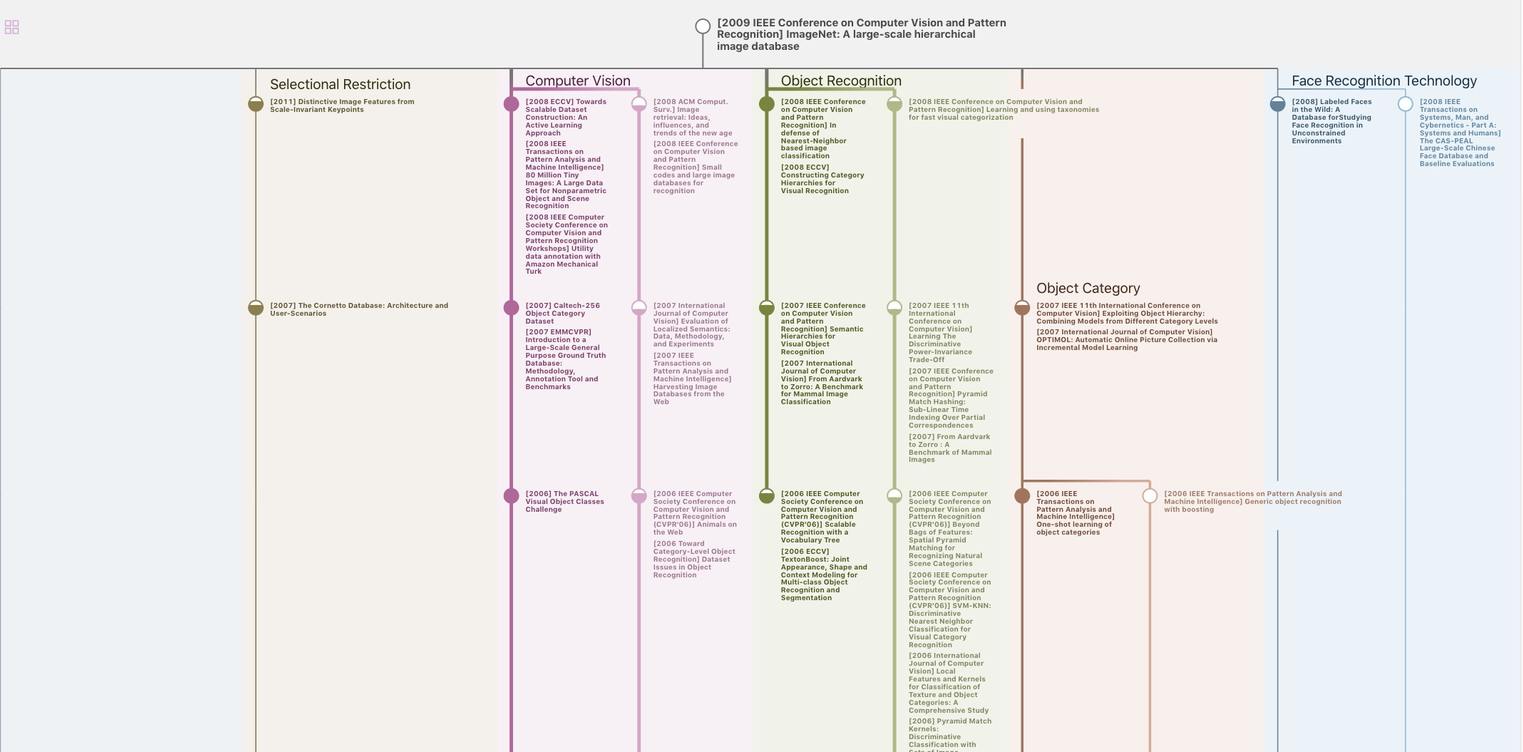
生成溯源树,研究论文发展脉络
Chat Paper
正在生成论文摘要