Dynamic Kernel Fusion for Bulk Non-contiguous Data Transfer on GPU Clusters
2020 IEEE International Conference on Cluster Computing (CLUSTER)(2020)
摘要
In the last decade, many scientific applications have been significantly accelerated by large-scale GPU systems. However, the movement of non-contiguous GPU-resident data is one of the most challenging components of scaling these applications using communication middleware like MPI. Although plenty of research has discussed improving non-contiguous data movement within communication middleware, the packing/unpacking operations on GPUs are still expensive. They cannot be hidden due to the limitation of MPI standard and the not-well-optimized designs in existing MPI implementations for GPU-resident data. Consequently, application developers tend to implement customized packing/unpacking kernels to improve GPU utilization by avoiding unnecessary synchronizations in MPI routines. However, this reduces productivity as well as performance as it cannot overlap the packing/unpacking operations with communication. In this paper, we propose a novel approach to achieve low-latency and high-bandwidth by dynamically fusing the packing/unpacking GPU kernels to reduce the expensive kernel launch overhead. The evaluation of the proposed designs shows up to 8X and 5X performance improvement for sparse and dense non-contiguous layout, respectively, compared to the state-of-the-art approaches on the Lassen system. Similarly, we observe up to 19X improvement over existing approaches on the ABCI system. Furthermore, the proposed design also outperforms the production libraries, such as SpectrumMPI, OpenMPI, and MVAPICH2, by many orders of magnitude.
更多查看译文
关键词
Datatype,GPU,MPI
AI 理解论文
溯源树
样例
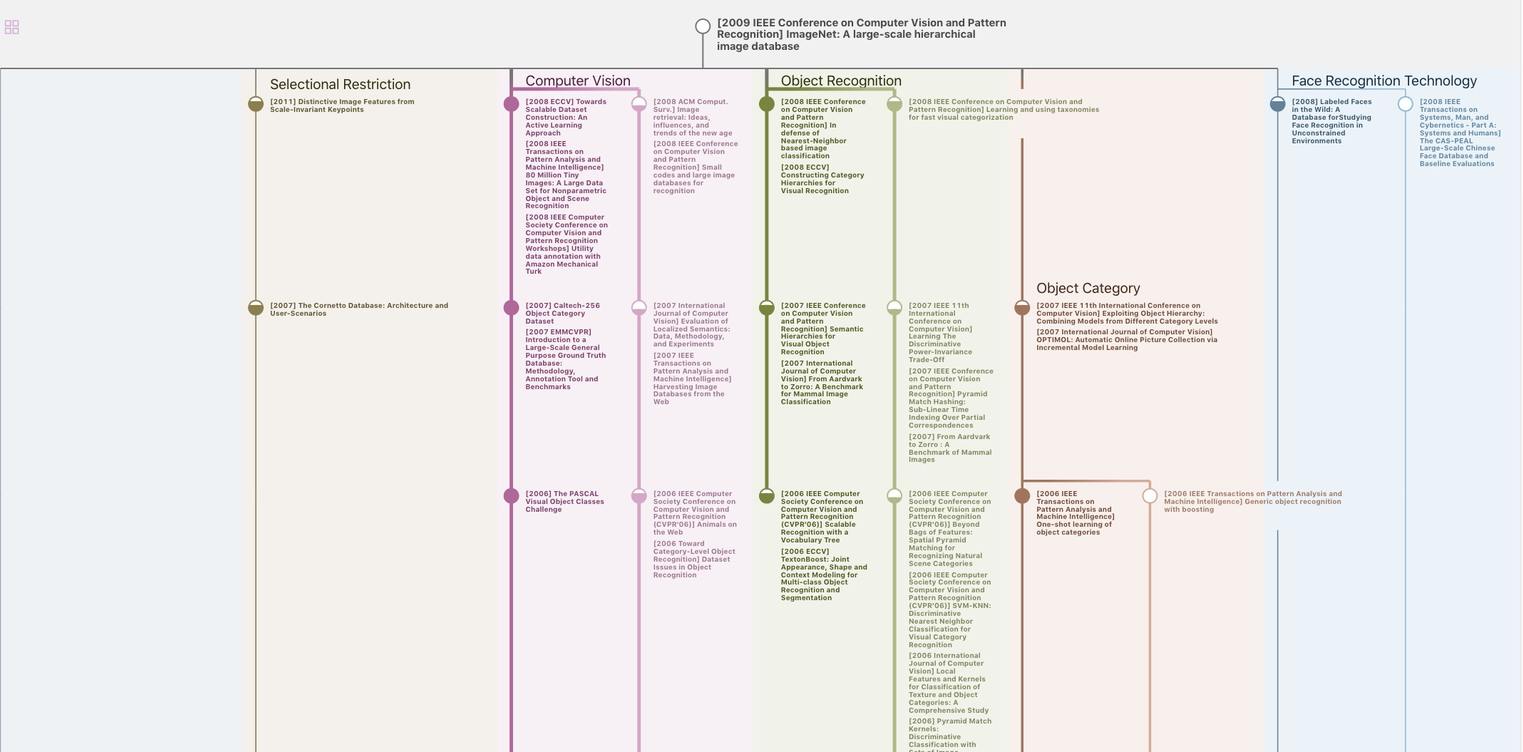
生成溯源树,研究论文发展脉络
Chat Paper
正在生成论文摘要