Staging Based Task Execution for Data-driven, In-Situ Scientific Workflows
2020 IEEE International Conference on Cluster Computing (CLUSTER)(2020)
摘要
As scientific workflows increasingly use extreme-scale resources, the imbalance between higher computational capabilities, generated data volumes, and available I/O bandwidth is limiting the ability to translate these scales into insights. In-situ workflows (and the in-situ approach) are leveraging storage levels close to the computation in novel ways in order to reduce the required I/O. However, to be effective, it is important that the mapping and execution of such in-situ workflows adopts a data-driven approach, enabling in-situ tasks to be executed flexibly based upon data content. This paper first explores the design space for data-driven in-situ workflows. Specifically, it presents a model that captures different factors that influence the mapping, execution, and performance of data-driven in-situ workflows and experimentally studies the impact of different mapping decisions and execution patterns. The paper then presents the design, implementation, and experimental evaluation of a data-driven in-situ workflow execution framework that leverages in-memory distributed data management and user-defined task-triggers to enable efficient and scalable in-situ workflow execution.
更多查看译文
关键词
in-situ workflows,in-situ/in-transit execution,data staging,dynamic task triggers
AI 理解论文
溯源树
样例
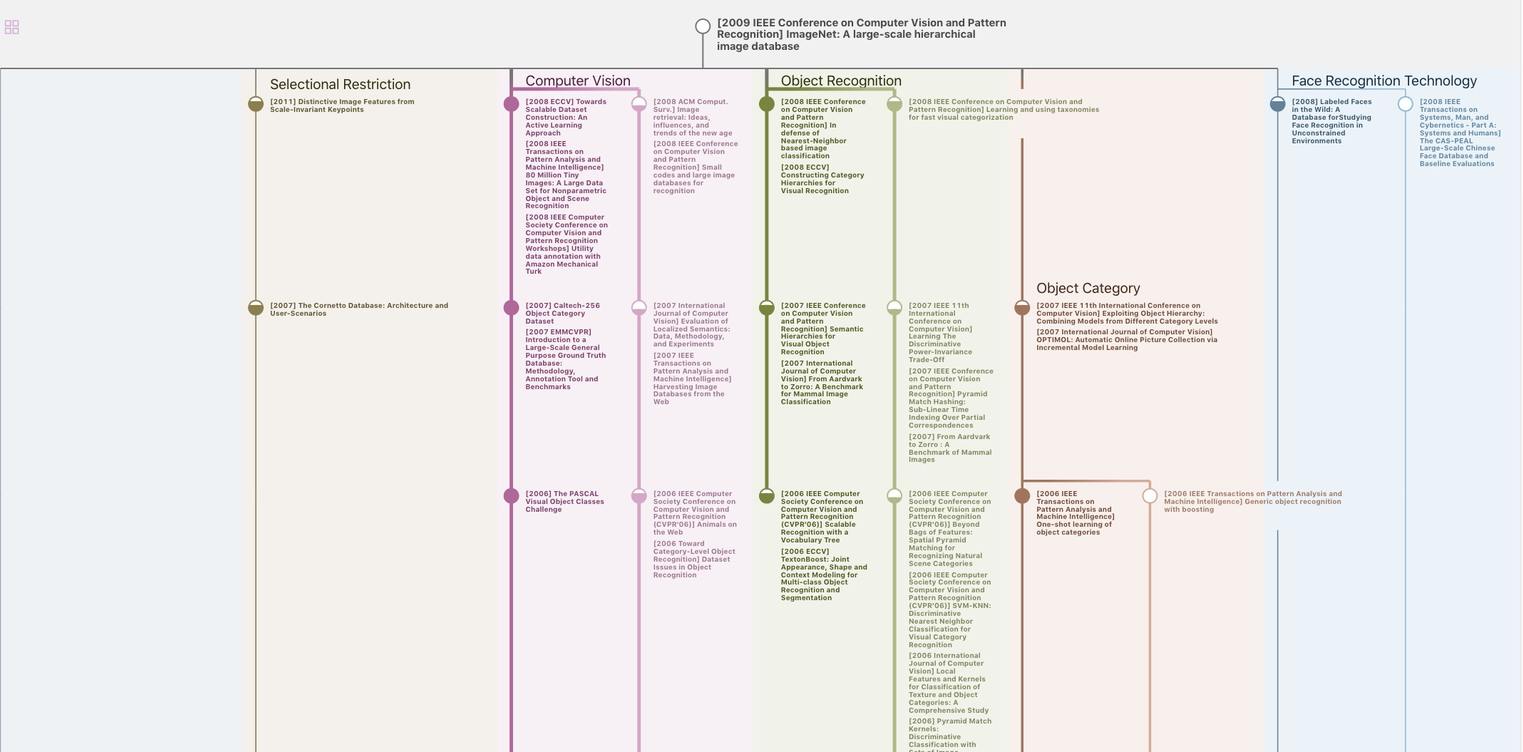
生成溯源树,研究论文发展脉络
Chat Paper
正在生成论文摘要