Reliable Saliency Maps for Weakly-Supervised Localization of Disease Patterns.
iMIMIC/MIL3ID/LABELS@MICCAI(2020)
摘要
Training convolutional neural networks with image-based labels leads to black-box image classification results. Saliency maps offer localization cues of class-relevant patterns, without requiring costly pixel-based labels. We show a failure mode for recently proposed weakly supervised localization models, e.g., models highlight the wrong input region, but classify correctly across all samples. Subsequently, we tested multiple architecture modifications, and propose two simple, but effective training approaches based on two-stage-learning and optional bounding box guidance, that avoid such misleading projections. Our saliency maps localize pneumonia patterns reliably and significantly better than gradCAM in terms of localization scores and expert radiologist’s ratings.
更多查看译文
关键词
localization,maps,weakly-supervised
AI 理解论文
溯源树
样例
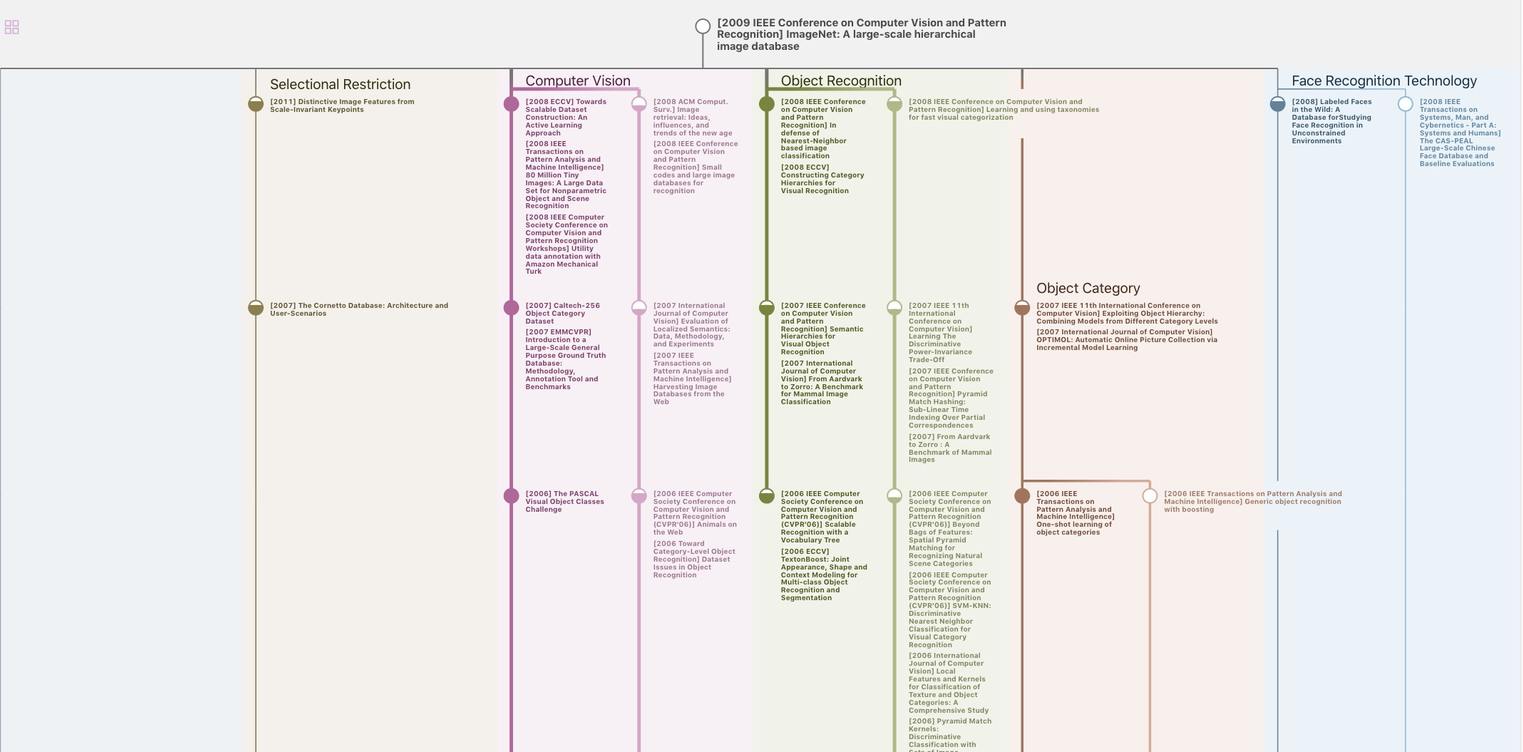
生成溯源树,研究论文发展脉络
Chat Paper
正在生成论文摘要