A Driver-Centric Vehicle Reposition Framework via Multi-agent Reinforcement Learning.
GPC(2020)
摘要
The e-hailing platforms have transformed the way people travel, live, and socialize. The efficiency of the platform is substantially influenced by the distribution differences between demands and supplies in the city. Therefore, an appropriate reposition vehicle strategy can significantly balance this distribution difference, which will promote platform benefits, customer goodwill and greatly alleviate traffic congestions. Due to the complicated relationship between vehicles and the temporal correlation of reposition actions, it is a challenging task to reposition vehicles in the city. Existing studies mostly focus on individual drivers that can hardly capture the relationship between drivers and long-term variations of demands and supplies in the city. In this paper, we introduce the reinforcement learning with geographic information and propose a geographic-based multi-agent deep deterministic policy gradient algorithm (gbMADDPG). The algorithm is driver-centric which takes the passenger searching time as an optimization goal to reduce the idle time of vehicles. We will demonstrate the effectiveness of our proposed algorithm framework through simulation experiments based on real data.
更多查看译文
关键词
Vehicle reposition, Multi-agent reinforcement learning, Deep reinforcement learning
AI 理解论文
溯源树
样例
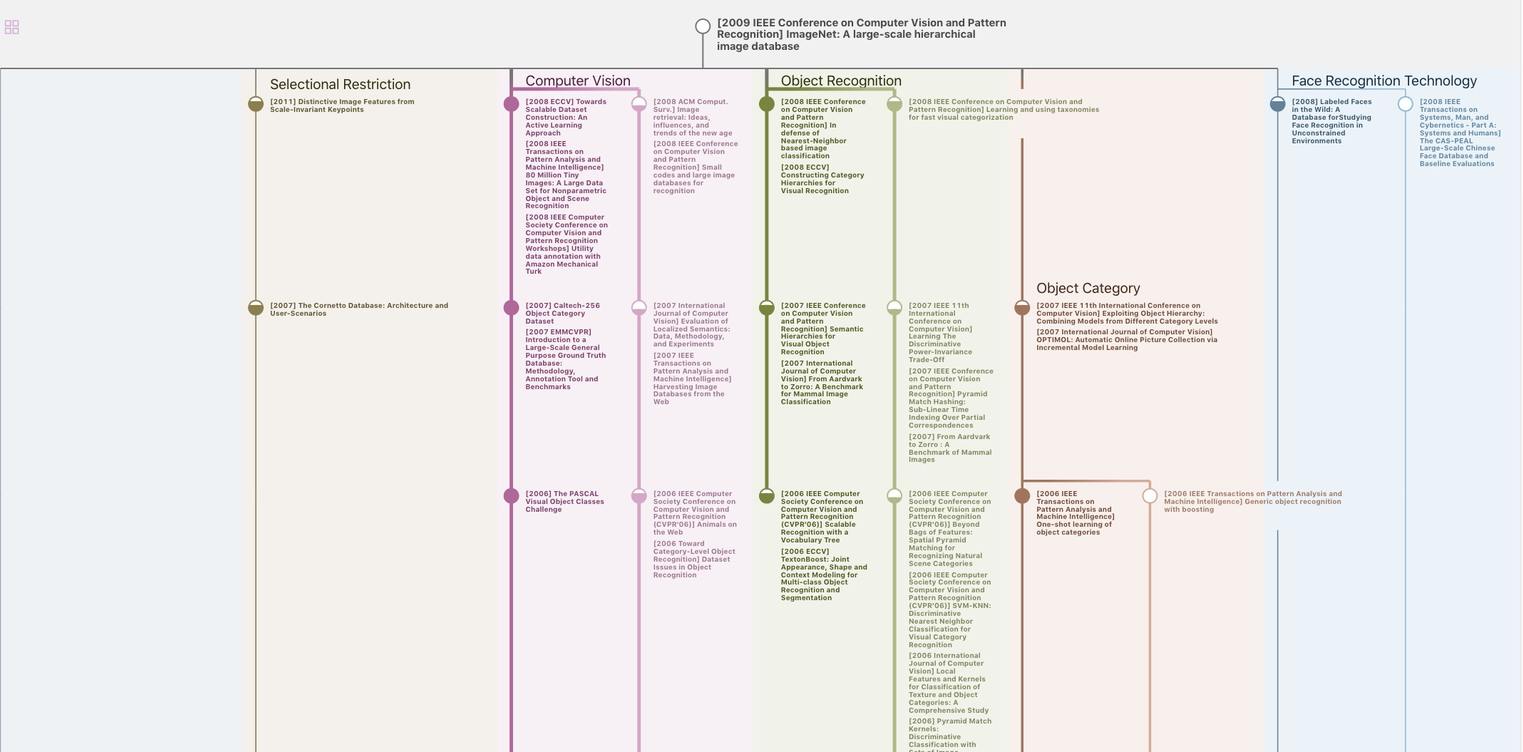
生成溯源树,研究论文发展脉络
Chat Paper
正在生成论文摘要