Interpretable Multivariate Time Series Classification Based on Prototype Learning.
GPC(2020)
摘要
Recently, the classification of multivariate time series has attracted much attention in the field of machine learning and data mining, due to its wide application values in biomedicine, finance, industry and so on. During the last decade, deep learning has achieved great success in many tasks. However, while many studies have applied deep learning to time series classification, few works can provide good interpretability. In this paper, we propose a deep sequence model with built-in interpretability by fusing deep learning with prototype learning, aiming to achieve interpretable classification of multivariate time series. In particular, an input sequence is classified by being compared with a set of prototypes, which are also sequences learned by the developed model, i.e., exemplary cases in the problem domain. We use the matched subset of the MIMIC-III Waveform Database to evaluate the proposed model and compare it with several baseline models. Experimental results show that our model can not only achieve the best performance but also provide good interpretability.
更多查看译文
关键词
Multivariate time series, Interpretable classification, Deep learning, Prototype
AI 理解论文
溯源树
样例
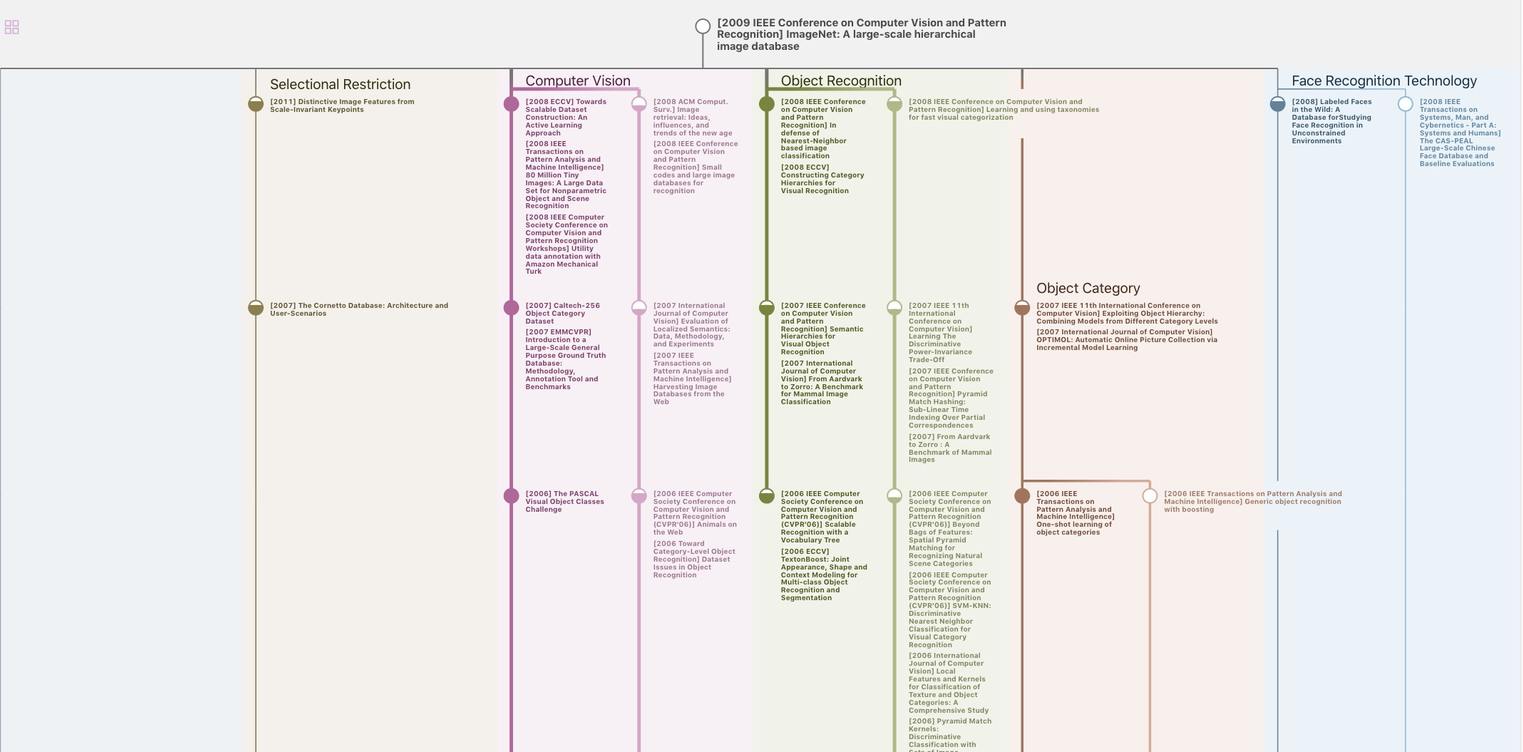
生成溯源树,研究论文发展脉络
Chat Paper
正在生成论文摘要