Filling Missing Values in Spatial-temporal Data Collected from Traffic Sensors
2020 IEEE International Smart Cities Conference (ISC2)(2020)
摘要
Intelligent transportation systems (ITS) are critical to any smart city strategy. They are used to optimize the flow of urban traffic which in turn leads to a reduction in time spent traveling. In order for ITS to work properly, sensors that collect real-time traffic floss information from streets and highways are required so the ITS can know the current slate of the traffic. However, such sensors are prone to failures and network faults. This poses a serious hindrance when performing data analysis and knowledge extraction on sensor data due to the fact that such data is composed of noisy and missing Vatu es In this work, we benchmark several deep learning based methods for filling missing values in a dataset collected from 2013 to 2015 in the city of Oporto, Portugal. The dataset is composed of readings of 26 sensors that measure traffic information in 5 minute intervals. Around 12% of all values are missing.
更多查看译文
关键词
Intelligent Transportation Systems,Neural networks,Machine Learning,Missing data
AI 理解论文
溯源树
样例
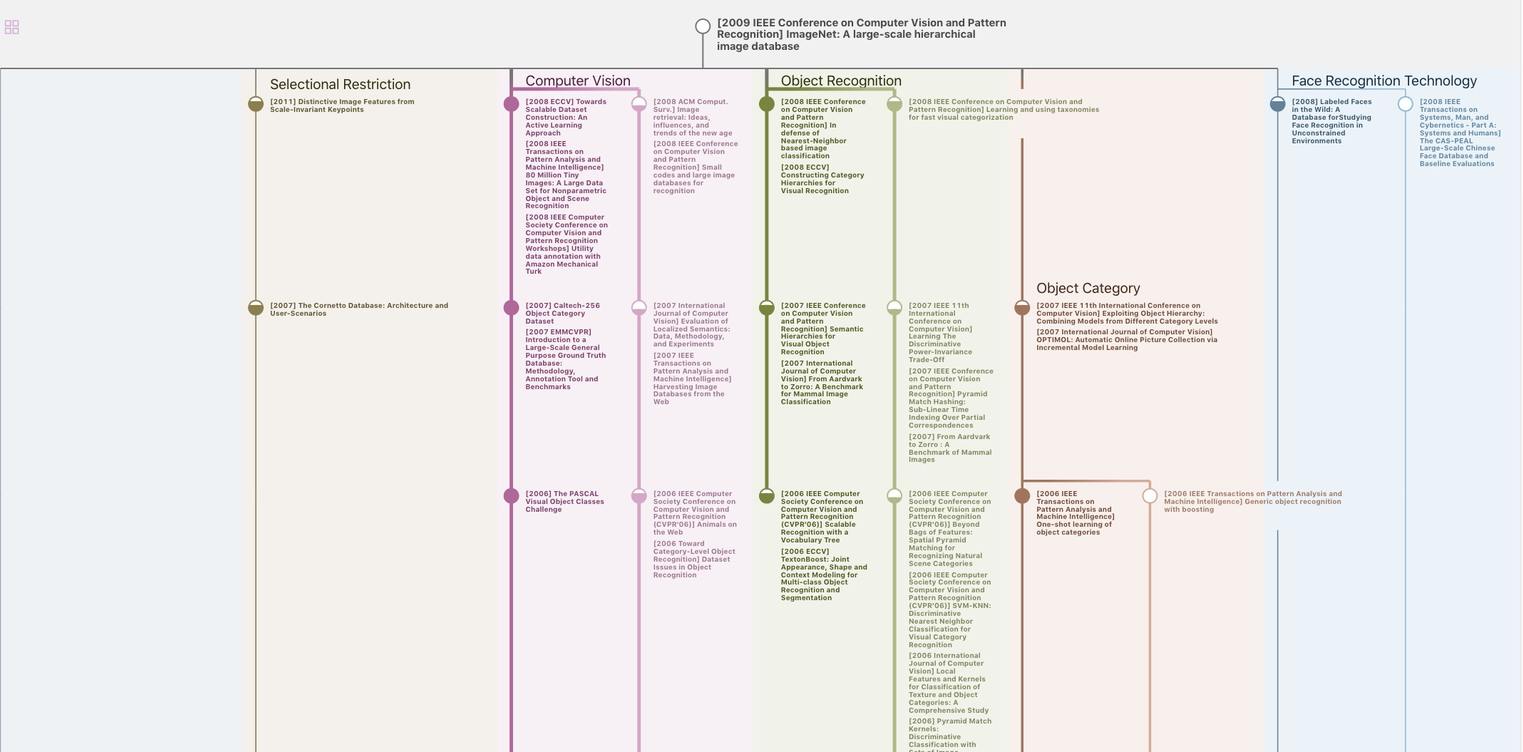
生成溯源树,研究论文发展脉络
Chat Paper
正在生成论文摘要