A Pragmatic Approach to Membership Inferences on Machine Learning Models
2020 IEEE European Symposium on Security and Privacy (EuroS&P)(2020)
Abstract
Membership Inference Attacks (MIAs) aim to determine the presence of a record in a machine learning model's training data by querying the model. Recent work has demonstrated the effectiveness of MIA on various machine learning models and corresponding defenses have been proposed. However, both attacks and defenses have focused on an adversary that indiscriminately attacks all the records without regard to the cost of false positives or negatives. In this work, we revisit membership inference attacks from the perspective of a pragmatic adversary who carefully selects targets and make predictions conservatively. We design a new evaluation methodology that allows us to evaluate the membership privacy risk at the level of individuals and not only in aggregate. We experimentally demonstrate that highly vulnerable records exist even when the aggregate attack precision is close to 50% (baseline). Specifically, on the MNIST dataset, our pragmatic adversary achieves a precision of 95.05% whereas the prior attack only achieves a precision of 51.7%.
MoreTranslated text
Key words
evaluation methodology,highly vulnerable records,membership privacy risk,pragmatic adversary,Membership Inference Attacks,machine learning models,pragmatic approach
AI Read Science
Must-Reading Tree
Example
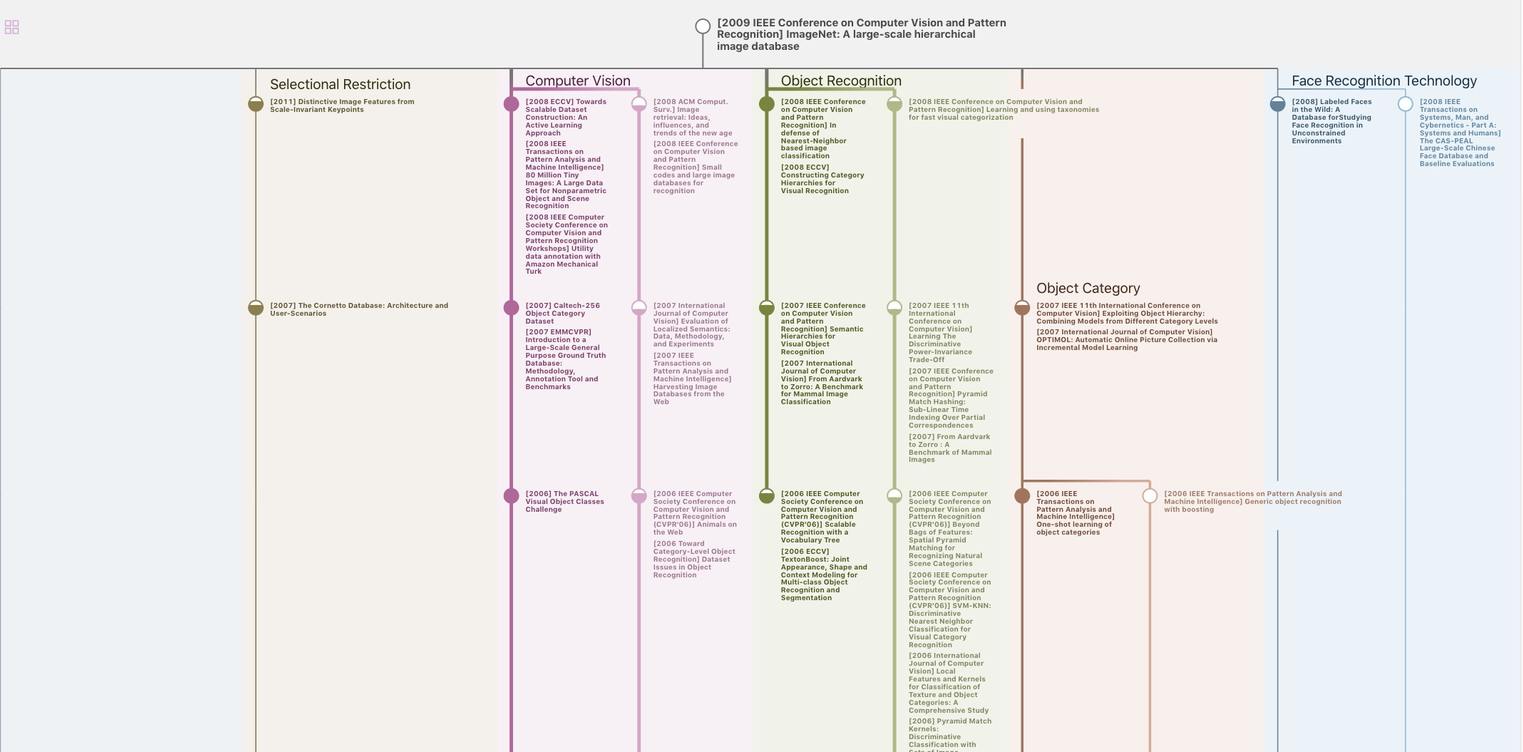
Generate MRT to find the research sequence of this paper
Chat Paper
Summary is being generated by the instructions you defined