Cross-Cancer Genome Analysis on Cancer Classification Using Both Unsupervised and Supervised Approaches.
BigData Congress(2020)
摘要
Many problems exist within the current cancer diagnosis pipeline, one of which is alarmingly high over-diagnosis rates in breast, prostate, and lung cancer. Through quantifying gene expression levels, next-generation sequencing techniques such as RNA-Seq offer an opportunity for researchers and clinicians to gain a more complete view of a cell’s transcriptome. With the adoption of this new data source, cross-cancer methods for cancer diagnosis have become more viable. We utilize mutual information in conjunction with a Gaussian mixture model and t-SNE to evaluate the separability of cancer and non-cancer tissue samples from RNA-Seq expression data. The Gaussian mixture and t-SNE combination produced clear clustering without supervision, suggesting the ability to separate tissue samples algorithmically. Afterwards, we use a collection of deep neural networks to classify tissue origin and status from tissue sample gene expressions. We use genes selected based on the prior mutual information technique. First, we select the top 500 genes from candidate genes without considerations for overlap in the predictability of those genes. We then applied Recursive Feature Elimination (RFE) to select 200 genes, thus accounting for covariation. We find that the performance using the top 500 genes is only slightly better than the 200 genes selected using RFE, and the two approaches achieved similar performance overall, indicating that only a small subset of genes is required for the identification of status and origin. This work indicates that RNA sequencing data is a useful tool for cross-cancer studies. Next steps include the implementation of a greater amount of non-cancer data from other datasets to decrease bias in model training.
更多查看译文
关键词
Cross-Cancer Genome, The Cancer Genome Atlas (TCGA), Mutual information, Recursive Feature Elimination (RFE), Least Absolute Shrinkage and Selection Operator (LASSO), Gaussian mixture model, Clustering, Dimension reduction, Neural network, MLP (Multilayer Perceptron)
AI 理解论文
溯源树
样例
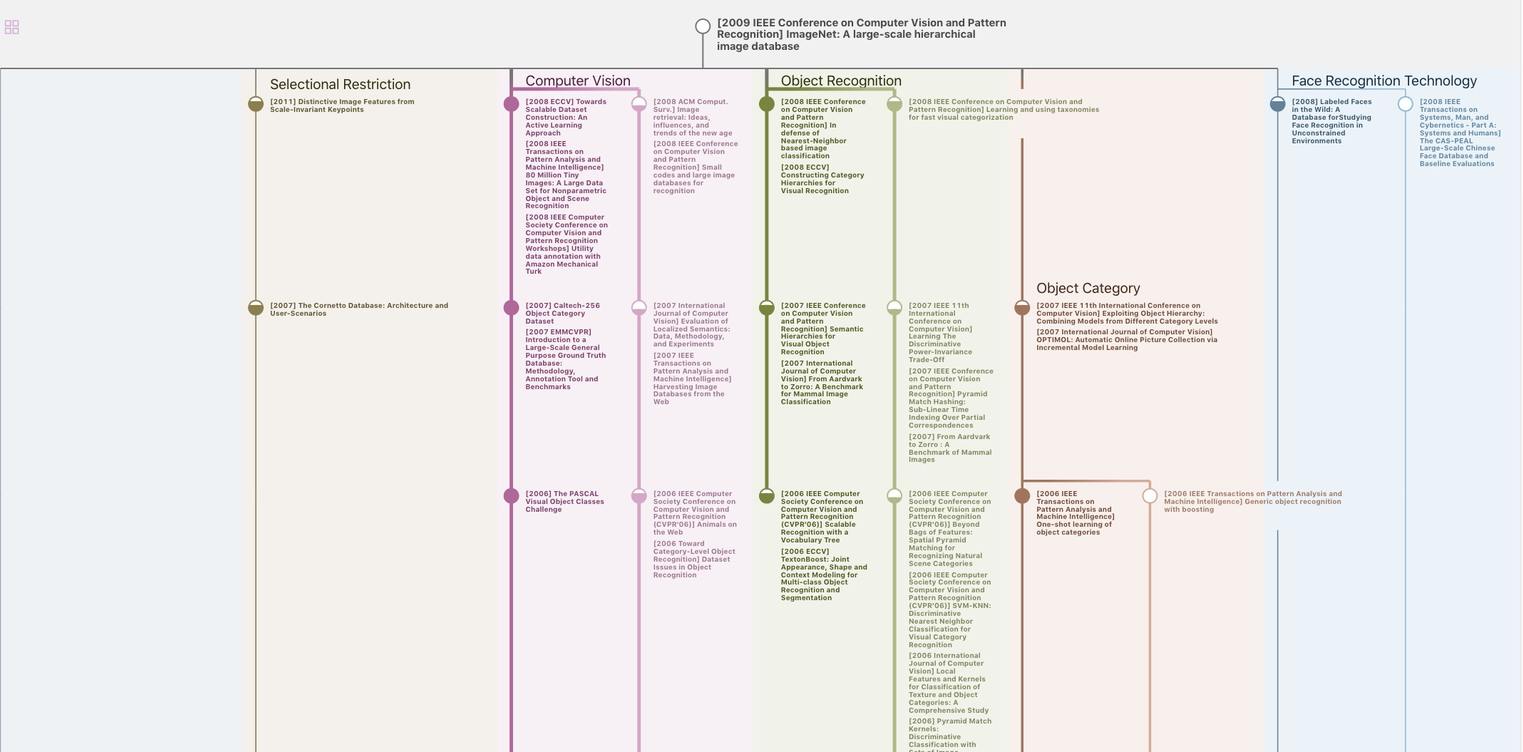
生成溯源树,研究论文发展脉络
Chat Paper
正在生成论文摘要