Tracking Moving Optima of Dynamic Multi-objective Problem via Prediction in Objective Space
CEC(2020)
摘要
Solving dynamic multi-objective optimization problem (DMOP) requires optimizing multiple conflicting objectives simultaneously. When a dynamic is detected in the changing environment, most of existing prediction-based strategies predict the trajectory of changing Pareto-optimal solutions (POS), based on the historical solutions obtained in the solution space. In this paper, we present a new prediction method to track the moving optima for solving DMOP. In contrast to existing approaches, we propose to build the prediction model in the objective space. As the evaluation for solving a DMOP is based on the Pareto-optimal front (POF), to predict directly in the objective space could provide more useful information than the prediction in the solution space. In particular, to efficiently capture the complex relationships among POFs found along the evolutionary search, here we build a prediction model in Reproducing Kernel Hilbert Space, which holds a closed-form solution. To evaluate the performance of the proposed method, empirical studies have been conducted by comparing against three state-of-the-art prediction-based strategies on fourteen commonly used DMOP benchmarks. The results obtained by using different optimization solvers confirmed the superiority of the proposed method for solving DMOP in terms of both solution quality and time efficiency.
更多查看译文
关键词
Evolutionary optimization,Dynamic Multi-objective optimization Problem,Prediction in Objective Space
AI 理解论文
溯源树
样例
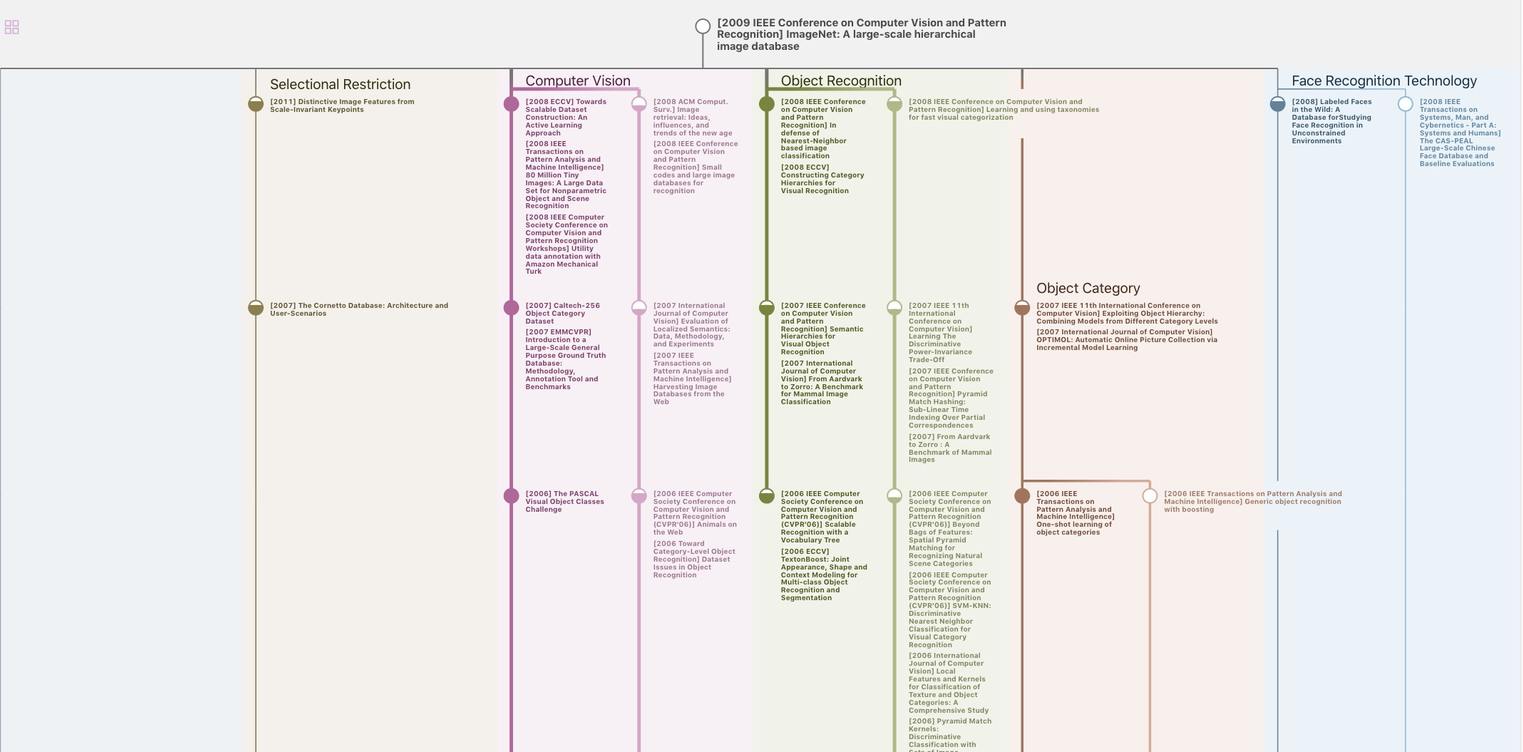
生成溯源树,研究论文发展脉络
Chat Paper
正在生成论文摘要