An Axiomatic Role Similarity Measure Based on Graph Topology.
SFDI/LSGDA@VLDB(2020)
摘要
RoleSim and SimRank are popular graph-theoretic similarity measures with many applications in, e.g., web search, collaborative filtering, and sociometry. While RoleSim addresses the automorphic (role) equivalence of pairwise similarity which SimRank lacks, it ignores the neighboring similarity information out of the automorphically equivalent set. Consequently, two pairs of nodes, which are not automorphically equivalent by nature, cannot be well distinguished by RoleSim if the averages of their neighboring similarities over the automorphically equivalent set are the same. To alleviate this problem: 1) We propose a novel similarity model, namely RoleSim*, which accurately evaluates pairwise role similarities in a more comprehensive manner. RoleSim* not only guarantees the automorphic equivalence that SimRank lacks, but also takes into account the neighboring similarity information outside the automorphically equivalent sets that are overlooked by RoleSim. 2) We prove the existence and uniqueness of the RoleSim* solution, and show its three axiomatic properties (i.e., symmetry, boundedness, and non-increasing monotonicity). 3) We provide a concise bound for iteratively computing RoleSim* formula, and estimate the number of iterations required to attain a desired accuracy. 4) We induce a distance metric based on RoleSim* similarity, and show that the RoleSim* metric fulfills the triangular inequality, which implies the sum-transitivity of its similarity scores. Our experimental results on real and synthetic datasets demonstrate that RoleSim* achieves higher accuracy than its competitors while retaining comparable computational complexity bounds of RoleSim.
更多查看译文
关键词
axiomatic role similarity measure,graph
AI 理解论文
溯源树
样例
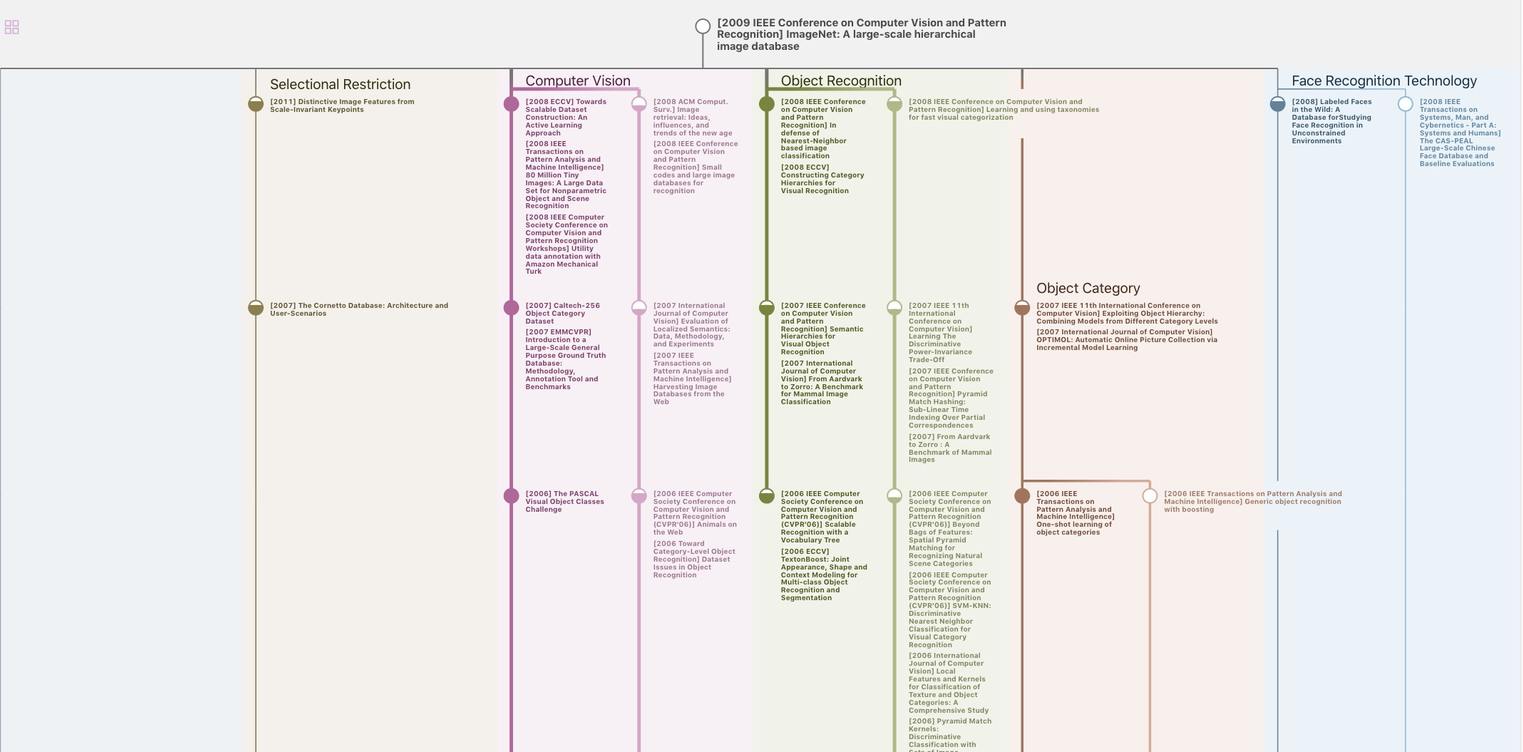
生成溯源树,研究论文发展脉络
Chat Paper
正在生成论文摘要