FISSA: Fusing Item Similarity Models with Self-Attention Networks for Sequential Recommendation
RECSYS(2020)
摘要
ABSTRACT Sequential recommendation has been a hot research topic because of its practicability and high accuracy by capturing the sequential information. As deep learning (DL) based methods being widely adopted to model the local and dynamic preferences beneath users’ behavior sequences, the modeling of users’ global and static preferences tends to be underestimated that usually, only some simple and crude users’ latent representations are introduced. Moreover, most existing methods hold an assumption that users’ intention can be fully captured by considering the historical behaviors, while neglect the possible uncertainty of users’ intention in reality, which may be influenced by the appearance of the candidate items to be recommended. In this paper, we thus focus on these two issues, i.e., the imperfect modeling of users’ global preferences in most DL-based sequential recommendation methods and the uncertainty of users’ intention brought by the candidate items, and propose a novel solution named fusing item similarity models with self-attention networks (FISSA) for sequential recommendation. Specifically, we treat the state-of-the-art self-attentive sequential recommendation (SASRec) model as the local representation learning module to capture the dynamic preferences beneath users’ behavior sequences in our FISSA, and further propose a global representation learning module to improve the modeling of users’ global preferences and a gating module that balances the local and global representations by taking the information of the candidate items into account. The global representation learning module can be seen as a location-based attention layer, which is effective to fit in well with the parallelization training process of the self-attention framework. The gating module calculates the weight by modeling the relationship among the candidate item, the recently interacted item and the global preference of each user using an MLP layer. Extensive empirical studies on five commonly used datasets show that our FISSA significantly outperforms eight state-of-the-art baselines in terms of two commonly used metrics.
更多查看译文
关键词
Sequential Recommendation, Self-Attention, Item Similarity Models, Gating Networks
AI 理解论文
溯源树
样例
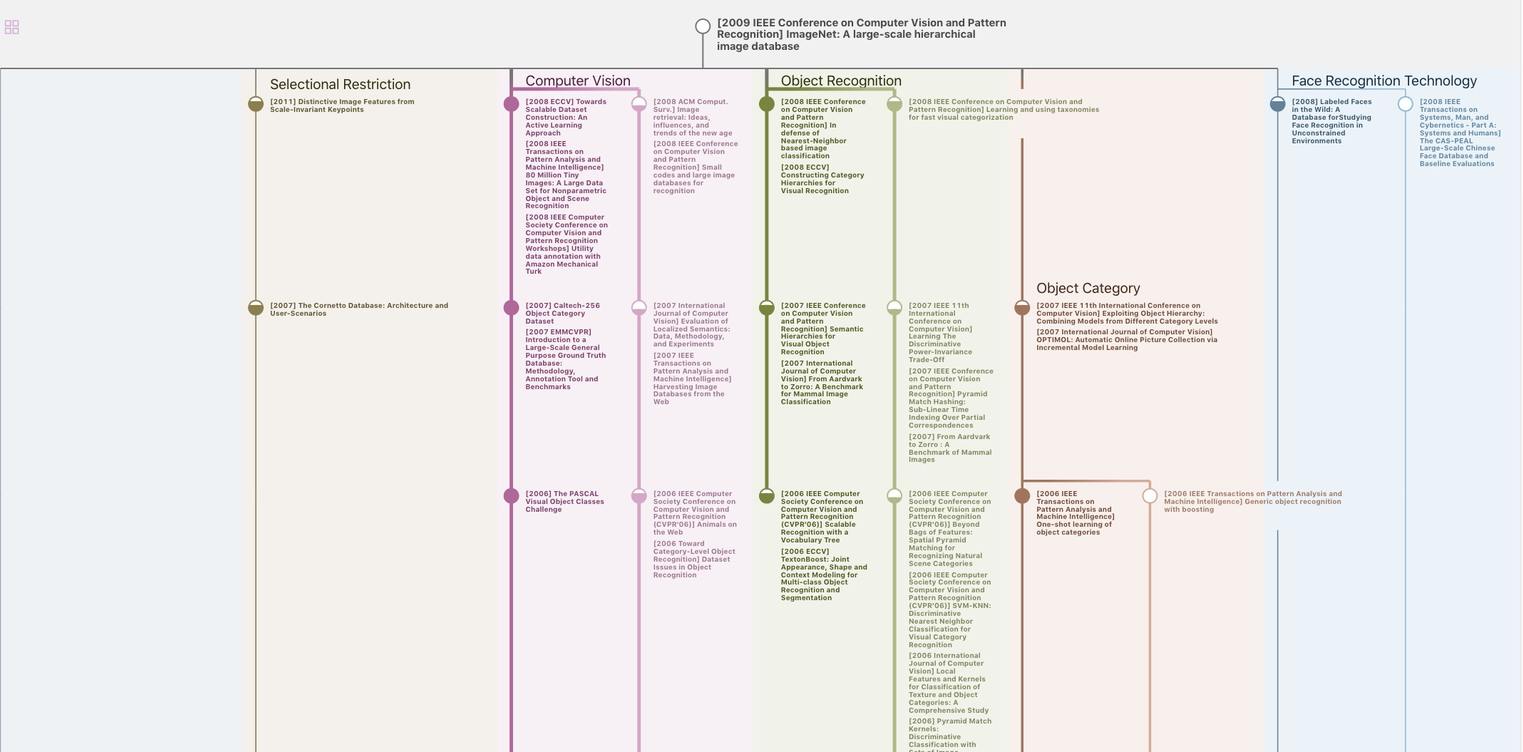
生成溯源树,研究论文发展脉络
Chat Paper
正在生成论文摘要