From the lab to production: A case study of session-based recommendations in the home-improvement domain
RECSYS(2020)
摘要
ABSTRACTE-commerce applications rely heavily on session-based recommendation algorithms to improve the shopping experience of their customers. Recent progress in session-based recommendation algorithms shows great promise. However, translating that promise to real-world outcomes is a challenging task for several reasons, but mostly due to the large number and varying characteristics of the available models. In this paper, we discuss the approach and lessons learned from the process of identifying and deploying a successful session-based recommendation algorithm for a leading e-commerce application in the home-improvement domain. To this end, we initially evaluate fourteen session-based recommendation algorithms in an offline setting using eight different popular evaluation metrics on three datasets. The results indicate that offline evaluation does not provide enough insight to make an informed decision since there is no clear winning method on all metrics. Additionally, we observe that standard offline evaluation metrics fall short for this application. Specifically, they reward an algorithm only when it predicts the exact same item that the user clicked next or eventually purchased. In a practical scenario, however, there are near-identical products which, although they are assigned different identifiers, they should be considered as equally-good recommendations. To overcome these limitations, we perform an additional round of evaluation, where human experts provide both objective and subjective feedback for the recommendations of five algorithms that performed the best in the offline evaluation. We find that the experts’ opinion is oftentimes different from the offline evaluation results. Analysis of the feedback confirms that the performance of all models is significantly higher when we evaluate near-identical product recommendations as relevant. Finally, we run an A/B test with one of the models that performed the best in the human evaluation phase. The treatment model increased conversion rate by 15.6% and revenue per visit by 18.5% when compared with a leading third-party solution.
更多查看译文
关键词
session-based recommendations, evaluation using human experts, comparison of offline evaluation metrics with labels from human experts, A/B test
AI 理解论文
溯源树
样例
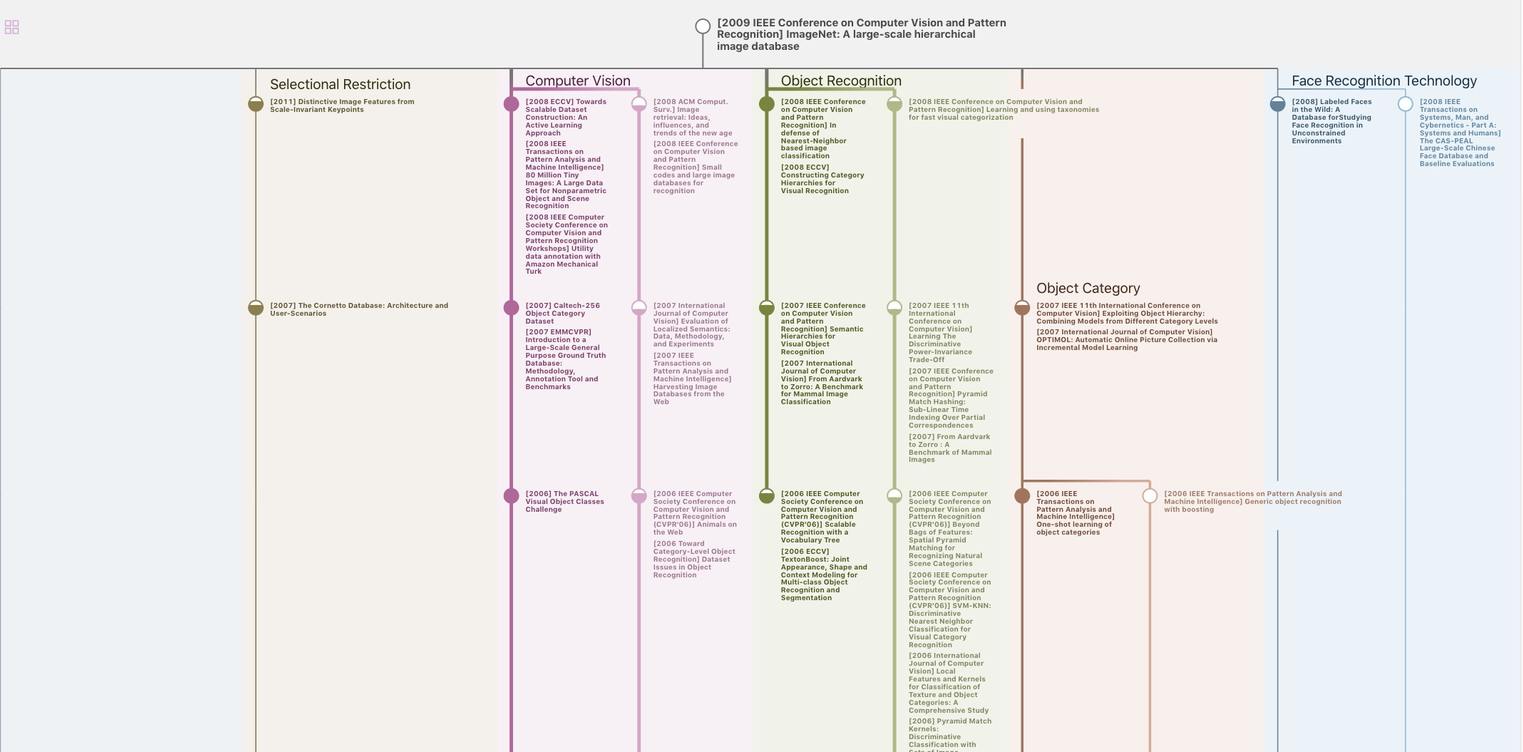
生成溯源树,研究论文发展脉络
Chat Paper
正在生成论文摘要