Near-duplicated Loss for Accurate Object Localization
2020 IEEE 7th International Conference on Data Science and Advanced Analytics (DSAA)(2020)
摘要
Multi-class object detection always involves the tasks of accurate target localization which is mainly related to bounding box regression. Smooth L1 loss is the most popular bounding box regression loss used in the current state-of-the-art object detection systems. However, such loss for regressing the parameters of a bounding box can't accurately and consistently regress the bounding box to the associated ground truth well. We instead propose the near-duplicated loss, a loss that better evaluate the disparity between the bounding box and the ground truth consistently. We present an approximate algorithm associated with a kernel function that not only considers the absolute distance but also involves the relative overlap area between the two bounding boxes. The new loss doesn't need additional supervision and is easy to embed into existing networks. Our final result, by incorporating the near-duplicated loss into the state-of-the-art object detection detectors (Faster RCNN, RetinaNet), shows consistent and significant improvements on popular object detection benchmarks (MS COCO and Pascal VOC).
更多查看译文
关键词
Object detection,accurate target localization,bounding box regression,Smooth L1 loss
AI 理解论文
溯源树
样例
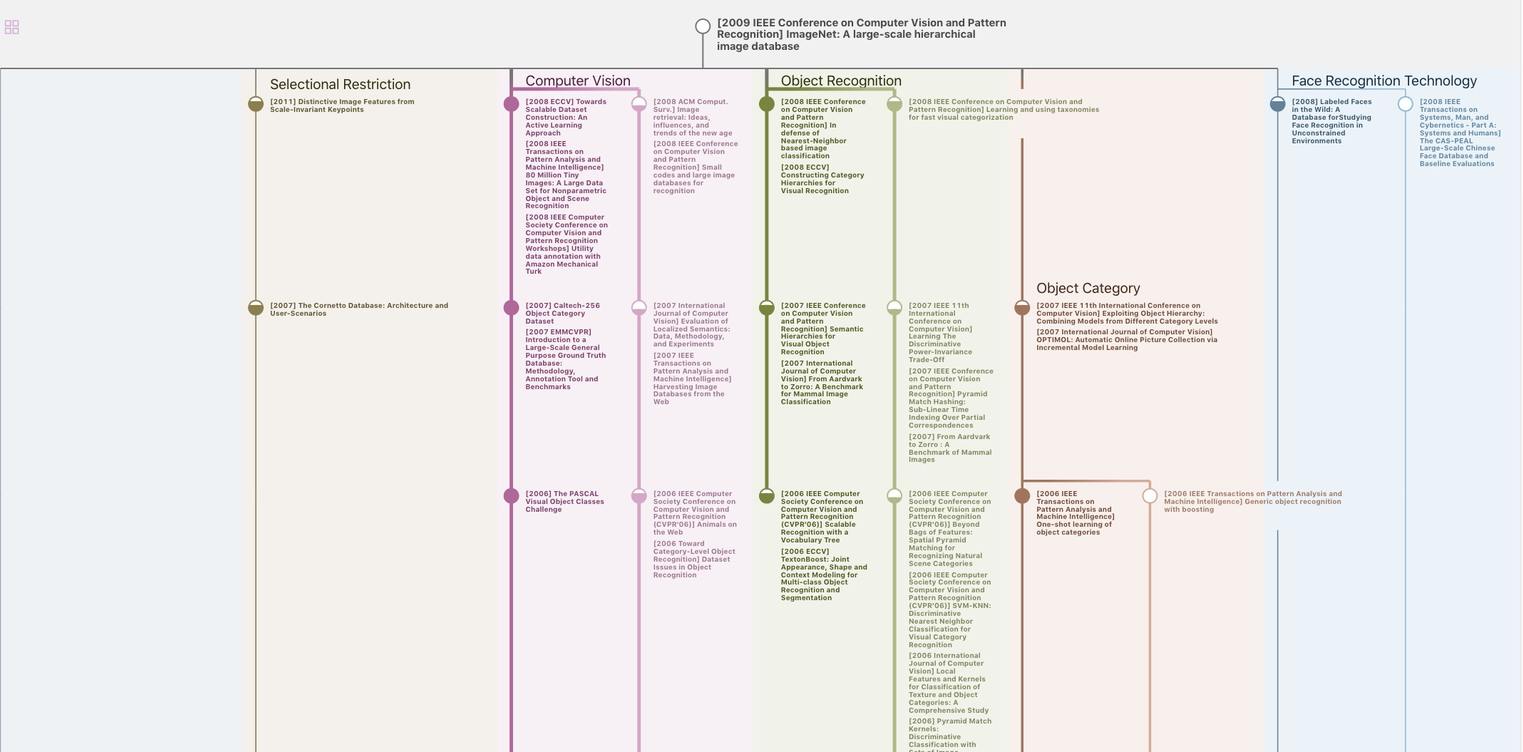
生成溯源树,研究论文发展脉络
Chat Paper
正在生成论文摘要