Learning Fair and Transferable Representations with Theoretical Guarantees
2020 IEEE 7th International Conference on Data Science and Advanced Analytics (DSAA)(2020)
摘要
Developing learning methods which do not discriminate subgroups in the population is the central goal of algorithmic fairness. One way to reach this goal is by modifying the data representation in order to satisfy prescribed fairness constraints. This allows to reuse the same representation in other context (tasks) without discriminate subgroups. In this work we measure fairness according to demographic parity, requiring the probability of the possible model decisions to be independent of the sensitive information. We argue that the goal of imposing demographic parity can be substantially facilitated within a multi-task learning setting. We leverage task similarities by encouraging a shared fair representation across the tasks via low rank matrix factorization. We derive learning bounds establishing that the learned representation transfers well to novel tasks both in terms of prediction performance and fairness metrics. We present experiments on three real world datasets, showing that the proposed method outperforms state-of-the-art approaches by a significant margin.
更多查看译文
关键词
Algorithmic Fairness,Learning Fair Representation,Demographic Parity,Multi-Task Learning,Transfer Representation
AI 理解论文
溯源树
样例
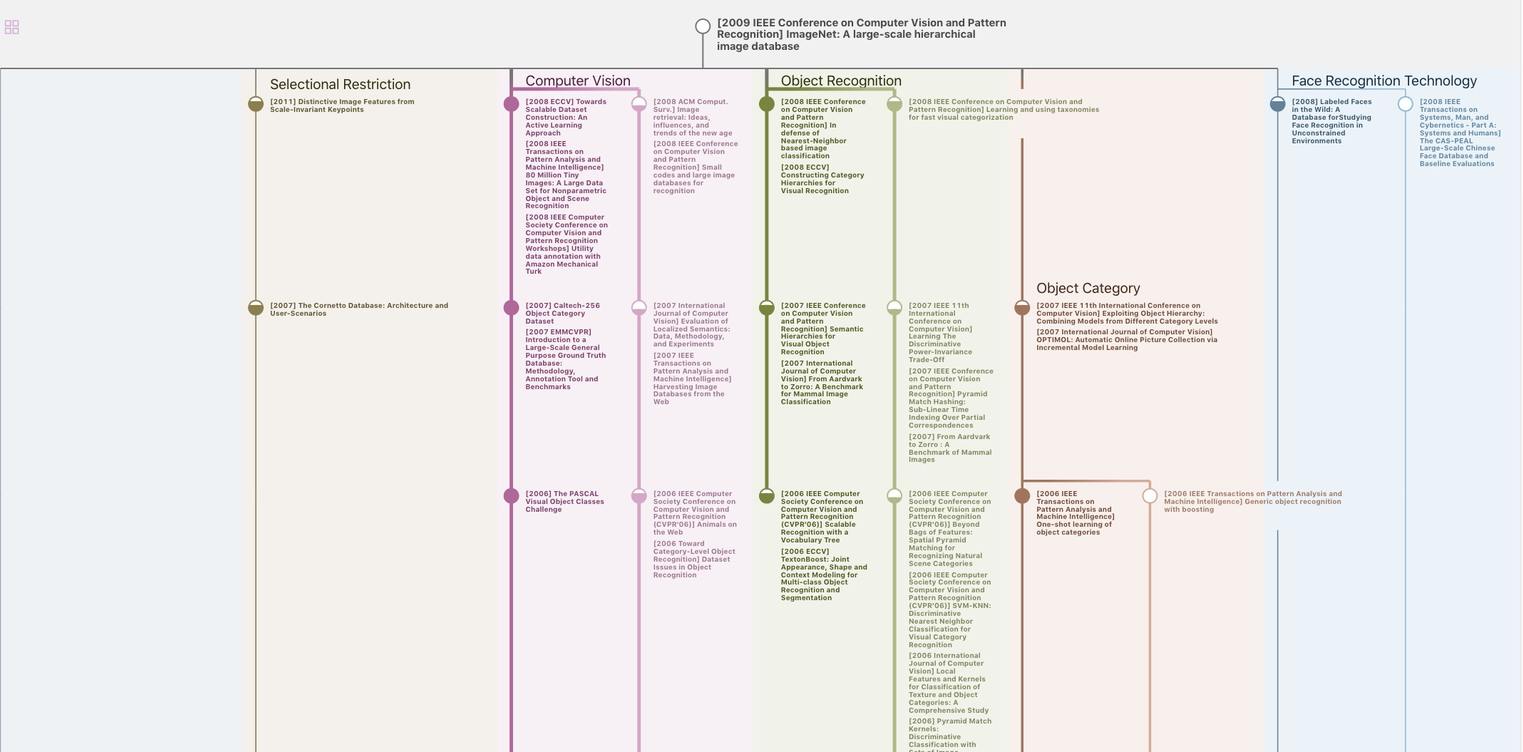
生成溯源树,研究论文发展脉络
Chat Paper
正在生成论文摘要