Pushing Data into CP Models Using Graphical Model Learning and Solving.
CP(2020)
摘要
Integrating machine learning with automated reasoning is one of the major goals of modern AI systems. In this paper, we propose a non-fully-differentiable architecture that is able to extract preferences from data and push it into (weighted) Constraint Networks (aka Cost Function Networks or CFN) by learning cost functions. Our approach combines a (scalable) convex optimization approach with empirical hyper-parameter tuning to learn cost functions from a list of high-quality solutions. The proposed architecture has the ability to learn from noisy solutions and its output is just a CFN model. This model can be analyzed, empirically hardened, completed with side-constraints, and directly fed to a Weighted Constraint Satisfaction Problem solver.
更多查看译文
关键词
Graphical Models, Cost Function Networks, Learning, Constraint Programming
AI 理解论文
溯源树
样例
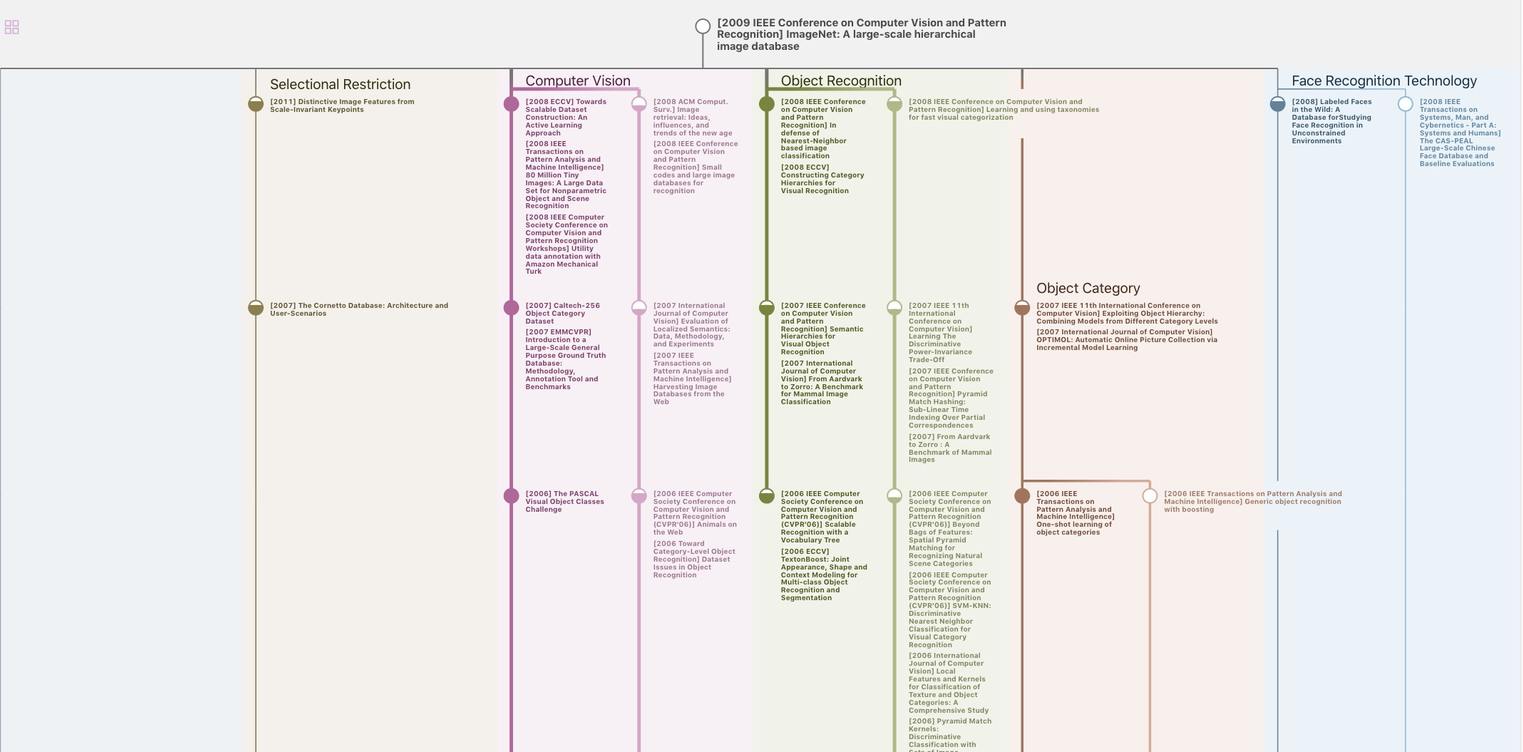
生成溯源树,研究论文发展脉络
Chat Paper
正在生成论文摘要